VIDEO
Poornima Muthukumar (Senior Technical Product Manager at Microsoft) shares how data can help product managers validate their assumptions, test their hypotheses, and measure their outcomes.
In this ELEVATE session, Poornima Muthukumar (Senior Technical Product Manager at Microsoft) shares how data can help product managers validate their assumptions, test their hypotheses, and measure their outcomes.
Attendees learn to build data-driven products backed by insightful analysis and how to utilize big data, data science and machine learning to inform complex product decisions.
Like what you see here? Our mission-aligned Girl Geek X partners are hiring!
- Check out open jobs at our trusted partner companies!
- Watch more ELEVATE 2024 videos from the event, or just the “Best Of 2024” Videos!
- Does your company want to sponsor a Girl Geek Dinner or Virtual Conference? Talk to us!
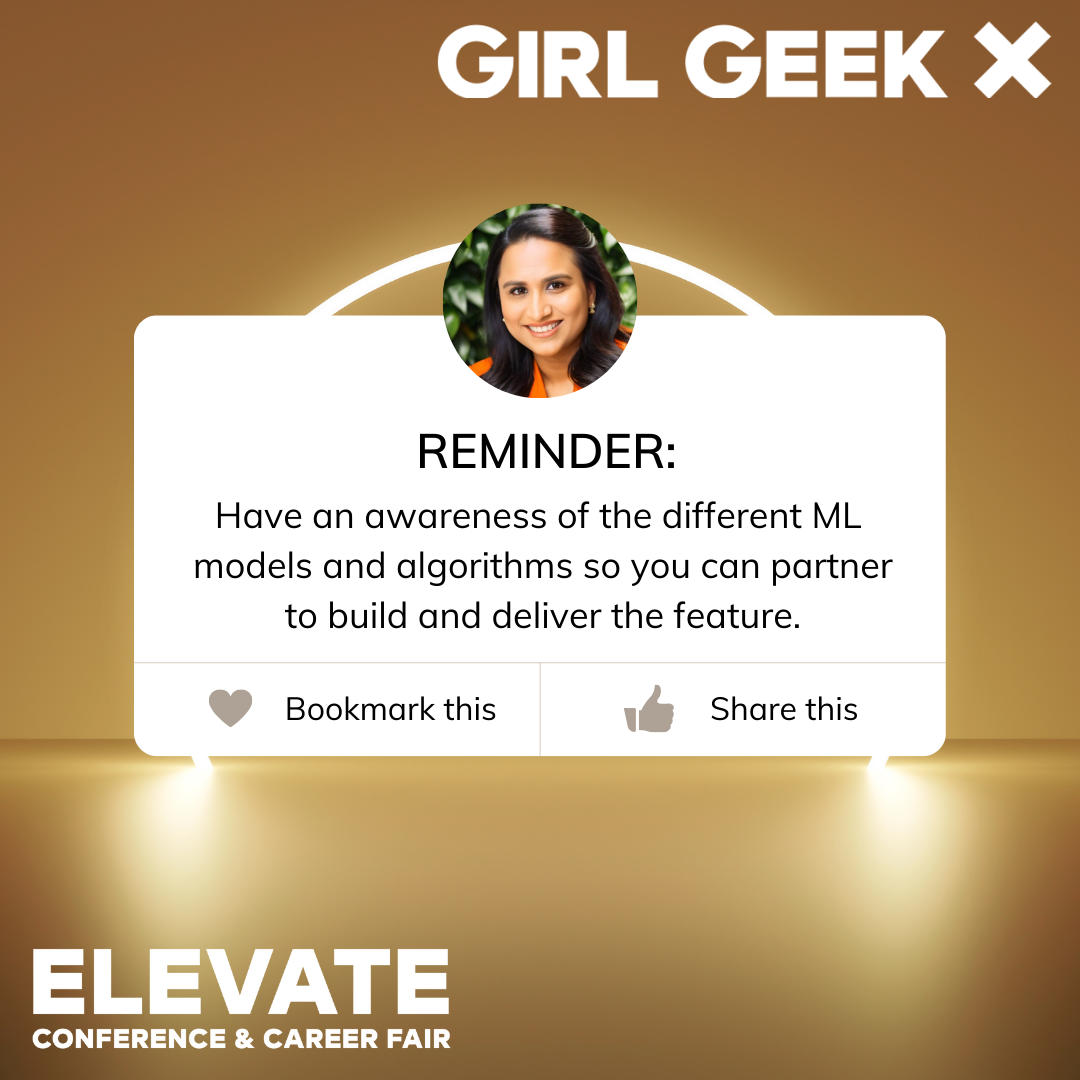
Transcript of ELEVATE Session:
Poornima Muthukumar:
Hi everyone. Good morning. Thank you so much for joining today’s presentation. I’m super excited to speak to all of you today on how to unlock product growth with big data, data science, and machine learning. Some of you might be interested in getting into a career either as a data scientist, business analyst, data engineer, technical product manager. so if you’re in any of these careers, I hope that this talk resonates with you and I hope that you can take back something for your job.
I also want to thank the Girl Geek IO for giving me this opportunity to speak to all of you today. And I want to add that I’m not speaking on behalf of Microsoft, but rather sharing the knowledge and experience that I have gained along the way in my journey. So yeah, without further ado, let’s get started. Brief look into today’s agenda so you know what you can expect from this talk.
First, we’ll go over my background so there is context on some of the things that I shared. Next, I will talk about how data is at the center of nearly every product you own and how that data is used to customize product to your needs, allowing companies like Netflix and Uber to build great data-driven products.
Next, we’ll talk about why companies need individuals who can use data from all of that big data, and what are those different data types that you as a product manager can leverage to extract insight to give customers the product that you want. And finally, if we have time, we will take some question and answers. Cool.
A brief background. I grew up in India. I spent a majority of my childhood in Mumbai and Chennai finishing my education in India. Post that I went to Singapore where I got my bachelor’s degree in computer engineering from the National University of Singapore. During my time at Singapore, I also interned at Bank of America and Goldman Sachs as a software engineer. After that, I went to New York where I worked in Goldman Sachs as a software engineer, building software for banking systems and capital market. After that, I went to Ireland where I worked in Microsoft Ireland research center as a software engineer in the office team. During there, I also traveled all across Europe, so that was a lot of fun.
After that, I came to Seattle where I grew in my career as a senior software engineer in the office release and delivery experience team at Microsoft. My team was basically in charge of delivering office updates that you got each month for all of your apps, like Word, Excel, PowerPoint on all platforms like Mac, iOS, windows, and Android. During this time is where I realized the power of big data and decided to pursue my part-time masters in data science from the University of Washington.
I also transitioned into my career as a senior technical product manager for the Microsoft 365 team because I wanted to have an end-to-end breadth of ownership of a product and be able to do that in a data-driven fashion. Today I am a data science volunteer at the Women in Data Science Puget Sound Community. I own patents in AI,ML, and big data at Microsoft. I am also volunteering at the UDub Foster School of Business as a product management accelerator.
Here I have five products that I want to quickly talk about how these companies are using data to drive their product growth. Netflix is something that all of us know how. Netflix uses data to build a recommendation model. They also use data to decide how to invest their money and what kind of producing content that resonates with user. They also use data to decide which movie to store and which CDN location based on where the users are streaming movie from in order to efficiently stream movies so that they can optimize for storage cost of CDN.
We know Tesla uses data for powering their autonomous driving system. They also have these cameras and sensors that’s constantly sending data back to Tesla, which in turn is used to optimize their self-driving car.
Amazon is one such product that uses data throughout their entire product stack. They use it for their search result optimization for price forecasting, warehouse optimization, inventory management. There’s just many, many ways that Amazon uses data because it has such a huge customer base. They have all of that huge amount of data which they can use to build and improve their product constantly.
Instagram, I’m sure all of you are aware that all the reels and all the contents and all the things that you see, there is a machine learning model that is running real time customized for you.
That is taking in all your engagement data, that is taking in all your usage data, which in turn is used to customize the model and send data back to you, which in turn gives you content that resonates with you in order to keep you on the product longer.
Next, we have Microsoft 365. Obviously now we have copilot. We have all of that ChatGPT integration that integrates with all your different Office 365 apps in order to give you in order to optimize your productivity suite experience with Microsoft, so if you see what is common to all of these products is they have a huge customer base that generate a huge amount of data, and today’s storage and compute and processing has become so cheap that you can store all of this data.
You can run data science techniques, you can run machine learning models, you can run algorithms on top of it to extract in site, which in turn can be used to optimize your product, which in turn can be used to build products that delight your customers.
Let’s say you join as a product manager for any of these products. You are constantly getting data from various signals. Could be feedback data, could be usage data, could be finance data, could be sales data engagement, data retention data.
How do you as a product manager organize all of this data in a clever way, in an intelligent way so that you can extract insight, which in turn can be used to drive product growth? How do you leverage those different data science algorithms techniques to optimize your product? Which is why I feel that the future of technical product management involves the melding of data science and product management because there’s so much that you can leverage to drive product optimization.
What you can expect from this talk is how to build data-driven products backed by insightful analysis and how can you utilize big data, data science and machine learning to inform complex product decisions.
Here are list seven techniques that I use in my day-to-day job to drive product growth and use data to drive them. First, I list the seven techniques, but because of the time constraint, I’ll only go in detail into three of them today in the talk. Tthe first one being funnel analysis, funnel analysis, how do you look at your customer journey end to end and see where customers are dropping off in the funnel so you can optimize your customer journey and thereby improve the conversion rate.
Next is retention analysis, right? Retention is a very important metric for any product. It’s great to have customers sign up for your product, but you also want to see of that, how many of them are actually using your product? How many of them are enjoying using your product? Let’s say you have a subscription service. You want to know what percentage of customers are renewing your subscription versus what percentage are canceling your subscription.
Next is segmentation analysis is how do you slice and dice your customers segment based on different things? Could be customer demographics, could be age, income, gender, their preferences, their needs of their purchase characteristics. How do you take all of this different data and slice and dice your customer into different segments, which will help you identify your most profitable segment and in turn cater your products differently to different segments?
Next is engagement analysis. This is how do customers interact with your product? How often do they interact with your product? How deeply do they interact with your product? What is it about your product that they like and what is it that they don’t like? So let’s say you have a website and you notice that majority of your customers have who visit the website, leave the website in a very short duration of time, right?
Let’s say you’re noticing that majority of your customers have a very short session duration. How do you use this data? Once you measure it, you have this data and now that you have that data, how do you use it to understand how you can improve engagement for your product?
Next is feedback. Feedback analysis is nothing but how do you collect feedback from various signal sources? Like could be feedback or [inaudible] ratings, reviews, all of that data and use that to understand what are your strengths and weaknesses for your product. And next is AB experimentation. This is where you show two different variations of your product to your customer and see which one resonates with your user and use that data to eventually launch the change to all the users.
And finally, machine learning. Machine learning is a very important tool that as a product manager you can leverage to give user centric and innovative solutions for your customers.
It’s important for you to know and have an awareness of what are the different machine learning models, algorithms so you can partner effectively with your engineering team, with your data science team to build the end-to-end pipeline to deliver the feature. Of these seven techniques, we will first look at funnel analysis. Like I already said, funnel analysis is a method used to analyze the sequence of events leading up to a point of conversion. Let’s say you have an e-commerce website.
Let’s look at one customer journey, right? Let’s say the customer came to your website, they searched for a product that they wanted to purchase, they added the product to cart, they went through checkout, and at which case they finally completed the purchase, right? This is just one customer, but not every customer will follow the same journey. Some maybe will come to your website, at which point they lose interest and they leave.
Some maybe will come to your website, they’ll add the product to cart, at which point they leave only a small section of customer eventually go all the way up till purchase, entering their payment details and completing, which is why it looks like a funnel. The ideal journey is obviously the whole thing. You want every customer to go through every step, but the funnel keeps getting shorter because customers keep dropping off.
Once you have this data, let’s say you measured this data for your journey for whichever feature you own, you measured the data in the form of a funnel, and let’s say you notice that majority of your customers are dropping off at the homepage, maybe you can hypothesize that your page is too slow, which is why customers are losing interest and they’re leaving. And whereas if you notice that majority of customers are leaving at the payment and checkout screen, at which point you can hypothesize, maybe the pricing is too expensive.
Once you have these different hypothesis, you can run experiments and improve the overall conversion rate for your product. Okay, next is AB experimentation. Here I have two different greeting cards for a Christmas, right? Maybe the one on the left resonates with the customers more and they click on it and they open it. Maybe the one on the right is not as appealing. Here, this is a trivial example.
In this case, the customer greetings, it maybe doesn’t matter if customers really open it and see it because it doesn’t translate into business outcome. But that’s not always the case, right? Let’s say you have an open house website, you want customers to click on the website, sign up for the open house so that your house is eventually sold, maybe in this case the color of the button results in different conversion rate and that it really matters what color of the button. That is something you can maybe experiment and see which one results in a higher conversion rate, not just for visual things.
Here I have Nike website, maybe the search algorithm on the left. There’s different from the search algorithm on the search result ranking on the right. Maybe the one on the left is resulting in higher units of shoes sold and higher revenue for the company, in which case you can totally AB experiment this as well.
What I mean to say here is that AB experimentation is not just limited to visual things, UI elements and things like that, but you could totally even AB experiment algorithms, APIs, backend systems or different systems that eventually translate into better user experience for your customer. So what exactly is AB testing? It is called split testing, bucket testing, randomized control experiment. It’s typically used to compare different versions of a webpage, but you can test anything from the color of a button to the backend algorithm to the layout of a page.
The AB groups are typically called control group and test group, and all elements are held constant except for that one thing that you really care about and you measure it. And it’s the best scientific way to establish causality with high probability. What it means really is that you’re not going by gut feeling, you’re not going by instant, but rather you’re running a scientific experiment and saying that based on the results of the experiment, I can conclusively say I can conclude that changing something results in a higher something else.
You can establish that causality in a very scientific way. What are the different stages of AB experiment is the first is you have a problem statement. You define the hypothesis, you design the experiment, you run the experiment, and then you eventually interpret the results based on the problem, based on the business that you’re in, based on the company that you’re running AB experiment. For you problem statements will be very different because you want the experiment to ladder up to the uber goal that the company has set.
Let’s say that I join as a product manager for a travel company like Expedia or booking.com. I will run experiments that eventually impact these metrics because that’s what the company cares about. The company wants to increase number of bookings, they want to increase their loyalty participation program, they want to increase maybe number of searches that people are conducting on their website.
Whereas if you are a media company like Netflix or Amazon Prime, they want to increase engagement, they want to increase subscription rate, they want to increase content consumption time. So your experiments that you run will impact different metrics. And as a product manager, if you’re running AB experimentation, you want to be very clear on the problem statement even before you get started, even before you design the experiment.
That is something you start off your ab experimentation process with. Again, if you’re an e-commerce company, your goal is to increase products viewed, products added to cart, resulting in higher conversion. And finally, if you’re a social media company like Instagram or Facebook, your goal is to increase engagement or maybe increase revenue through advertisement and things like that. Here what I’ve captured is that the problem statement could be very, very different, and that is something you want to be very clear about and define it at the start of the process itself.
Next is defining the hypothesis, right? A hypothesis is nothing but a testable statement that predicts how changing something will affect certain metric or a user behavior. So here these are the three steps that I use to define the hypothesis is you want to be clear on the problem based on evidence, and you want to decide changing something impact certain outcome and how that impacts the problem.
How do you know you have achieved the outcome is when you see the metrics change, right? Here below I have defined an example of how you could do that. So let’s say you are a product manager for an e-commerce website. You’re seeing lesser number of units sold on the website through sales data. That is the problem you have and that is the evidence you have.
Let’s say you believe that incorporating something like a social things like X number of people purchase in the last 24 hours will influence them to purchase and make the purchase. That will result in people actually converting. And that’s your gut feeling and that’s your hypothesis that you start off with. At the end of the experiment, you’re seeing whether indeed doing that change results in higher revenue and higher units sold. So that is what your null hypothesis is, and that is what your alternate hypothesis.
You can also define the significance level and statistics, power, and these are industry standards that you use a level of 0.05 and 0.8 to define the sample size that you want to use for running the hypothesis. Next is designing the experiment. When you design the experiment, you want to be very clear on what the metric is.
The primary metric, and you also want to be clear on the revenue. Maybe you have one primary metric, but maybe in this case it is revenue per user per month. But you could also have secondary metric and other metric that you want to test. You also want to determine the population that you want to test it for. Let’s say whether you want to run the experiments specifically in US in Europe for certain section of the market or all users.
Next is how many people do you want to run the experiment for is determining the sample size here I already talked about using an industry standard of alpha and power to determine how big your sample size should be in order to have statistically significant data to draw conclusion.
And finally, how long do you want to run the experiment? In this case, you could run it for two weeks, you could run it for two months. You can run it for much longer. And you also need to think about seasonality days of the week and holidays. You don’t want to design some email engagement experiment during holiday season when people are on vacation, not really checking their emails. Those are some factors you would decide take into factor when you’re designing the experiment.
Next is once you have all of these things finalized, you randomly assign users to group A and group B, and it’s very important to randomize so you’re not introducing any bias into the process. And you partner with the dev team to instrument logging for any necessary metric, collecting data to make sure you have a dashboard that surfaces the metric that you care about.
As you can see on the right, you are tracking revenue and you’re tracking how does revenue differ between the control group and the treatment group. And that will help you decide how your experiment is doing. And then you want to avoid looking at results before running the experiment for the entire duration of it and avoid peaking and jumping into conclusion. And then finally, once the experiment is run, you want to make sure that the data is reliable.
You want to perform some sanity check. If the data is obviously unreliable, you want to discard it and rerun it and then make some trade offs. Let’s say at the start of the experiment, you decided to measure engagement and revenue. And at the end of the experiment you saw that, okay, based on the changes that you’ve introduced, revenue is looking good, it’s going up, that’s great.
But if engagement is going down, you want to make the trade off that. Is it really worth introducing the change? How do you want to look at the result? How do you want to interpret the result and things like that? And then eventually launch the change to everyone. This is one way you take a data-driven approach to introduce changes.
An AB experimentation is widely used within Microsoft is something I’ve used throughout my career. We have these office bills that are released each month to millions of users, so before we introduce a change to such a worldwide population, we launch it to a small segment of population.
We collect telemetry signals, we collect all the signals, crash signals, we make sure that it’s looking good, and then eventually launch the change through a different release pipeline that we have. And that is something that throughout industry, it’s practiced in Instagram everywhere where they test some change with a small section of user, use that data to then eventually launch the change.
Cool. Next one is machine learning. Machine learning is not a magic wand, but it’s an application of AI that provides system the ability to learn and improve from experience without being explicitly programmed. When do you want to use machine learning is when you have lots of data, when you have a complex logic, something that cannot be solved with if statements cannot be solved by classic programming. That’s a good example.
When you want introduce some sort of personalization, like you have the case with Uber, you have the case with DoorDash, Instacart, all of them provide you a very personalized experience. And when you want the system to learn with time, that’s also a classic example where you want to introduce machine learning. Something like Twitter, what’s standing on Twitter today might not be training tomorrow. And that’s where machine learning is a classic example and fits the scenario.
Here I have three different types of machine learning. One is the supervised machine learning where you have machine learns from training data that is labeled where you train the system while it learns to do on its own. Next is you have non labeled training data. And finally is reinforcement learning where the machine learns on its own.
Here I’ve listed quickly different techniques of machine learning that you can use. One is ranking. This is something I already talked about that Amazon uses machine learning for, powering the search result ranking recommendation. Again, Netflix uses it for powering their home screen. Different recommendation, I guard them.
The great thing about recommendation, it doesn’t have to be perfect as long, it’s close to accurate. Customers are happy classification. Facebook uses it for tagging different users on their product. Classic example of classification regression is something we use for seeing, for casting, clustering for Spotify, uses it for clustering songs. And finally, chase uses anomaly detection for flagging fraudulent transaction. Thank you.
Sukrutha Bhadouria:
Thank you so much. This was a wonderful session. Yes, going to hop on to the next one. Thank you so much.
Like what you see here? Our mission-aligned Girl Geek X partners are hiring!
- Check out open jobs at our trusted partner companies!
- Watch more ELEVATE 2024 videos from the event, or just the “Best Of 2024” Videos!
- Does your company want to sponsor a Girl Geek Dinner or Virtual Conference? Talk to us!