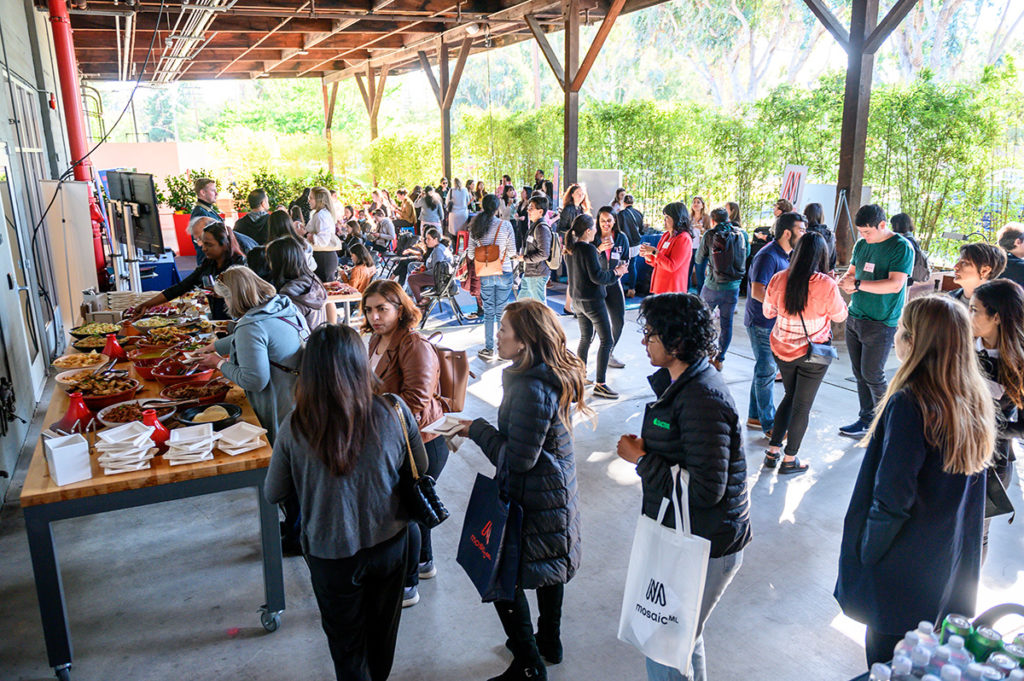
Over 120 girl geeks joined networking and talks at the sold-out MosaicML Girl Geek Dinner from women working in machine learning at MosaicML, Meta AI, Atomwise, Salesforce Research, OpenAI, Amazon, and Hala Systems.
Speakers discuss efficient machine learning training with MosaicML, reinforcement learning, ML-based drug discovery with AtomNet, evaluating recommendation robustness with RGRecSys, turning generative models into products at OpenAI, seeking the bigger picture at AWS, and more.
Table of Contents
Welcome – Julie Choi, VP and Chief of Growth at MosaicML – watch her talk or read her words
Making ML Training Faster, Algorithmically – Laura Florescu, AI Researcher at MosaicML – watch her talk or read her words
Reinforcement Learning: A Career Journey – Amy Zhang, Research Scientist at Meta AI – watch her talk or read her words
Addressing Challenges in Drug Discovery – Tiffany Williams, Staff Software Engineer at Atomwise – watch her talk or read her words
Evaluating Recommendation System Robustness – Shelby Heinecke, Senior Research Scientist at Salesforce Research – watch her talk or read her words
Turning Generative Models From Research Into Products – Angela Jiang, Product Manager at OpenAI – watch her talk or read her words
Seeking the Bigger Picture – Banu Nagasundaram, Machine Learning Product Leader at Amazon Web Services – watch her talk or read her words
10 Lessons Learned from Building High Performance Diverse Teams – Lamya Alaoui, Director of People Ops at Hala Systems – watch her talk or read her words
Like what you see here? Our mission-aligned Girl Geek X partners are hiring!
- See open jobs at MosaicML and check out open jobs at our trusted partner companies.
- Find more MosaicML Girl Geek Dinner photos from the event – please tag yourselves!
- Does your company want to sponsor a Girl Geek Dinner? Talk to us!
Transcript of MosaicML Girl Geek Dinner – Lightning Talks:
Angie Chang: Thank you so much for coming out. I’m so glad you’re here. My name is Angie Chang and I’m the founder of Girl Geek X. We’ve been doing Girl Geek Dinners in the San Francisco Bay Area for, if you can believe it, almost 15 years now. It’s the first Girl Geek Dinner in over two years, because Julie is a rock star and wanted to do a Girl Geek Dinner in person in the pandemic and we’re like, “Yes!” It was postponed and now in May, we’re finally doing this event. I’m so glad that we have a sold out event of amazing women in machine learning that we’re going to be hearing from tonight!
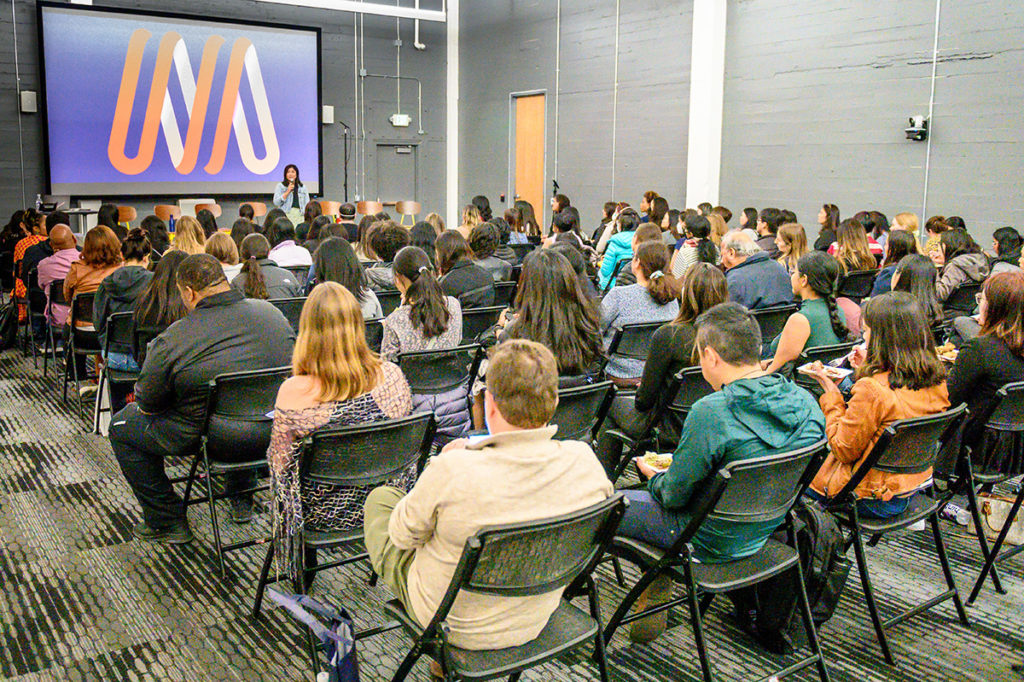
Girl Geek X founder Angie Chang welcomes the sold-out crowd to our first IRL Girl Geek Dinner in over two years during the pandemic! (Watch on YouTube)
Angie Chang: I don’t want to steal too much of the time, but I wanted to do a quick raffle of a tote bag that I have. I’m going to ask, who has been to the first Girl Geek Dinner and can name a speaker from that event? Sometimes I meet people who have. Are we making this really hard? Okay, the first Girl Geek Dinner was at Google. We had over 400 women show up for a panel of inspiring women. I just wanted to see because that’s a lot of people. Who thinks they’ve been to the most Girl Geek Dinners in the room? Okay.
Audience Member: It’s actually not me, but like, this clutch was designed by somebody who hates women because its super heavy – and I see that that [Girl Geek X tote] has handles.
Angie Chang: Okay, so we have a full agenda of machine learning lightning talks and I’m going to introduce you to our host for tonight. Julie Choi is the Chief Growth Officer of MosaicML and she is an amazing supporter of women and I would like to invite her up.
Julie Choi: Oh, thank you. Hi everyone. Thank you so much for coming out to the MosaicML Girl Geek Dinner. I am Julie Choi and I actually did go to the first Girl Geek Dinner. I want to thank Angie Chang and the Girl Geek Dinner organization. Angie has just been a pioneer and truly, just a very special person, bringing us together ever since, was that 2010 or something, I don’t know. When was that?
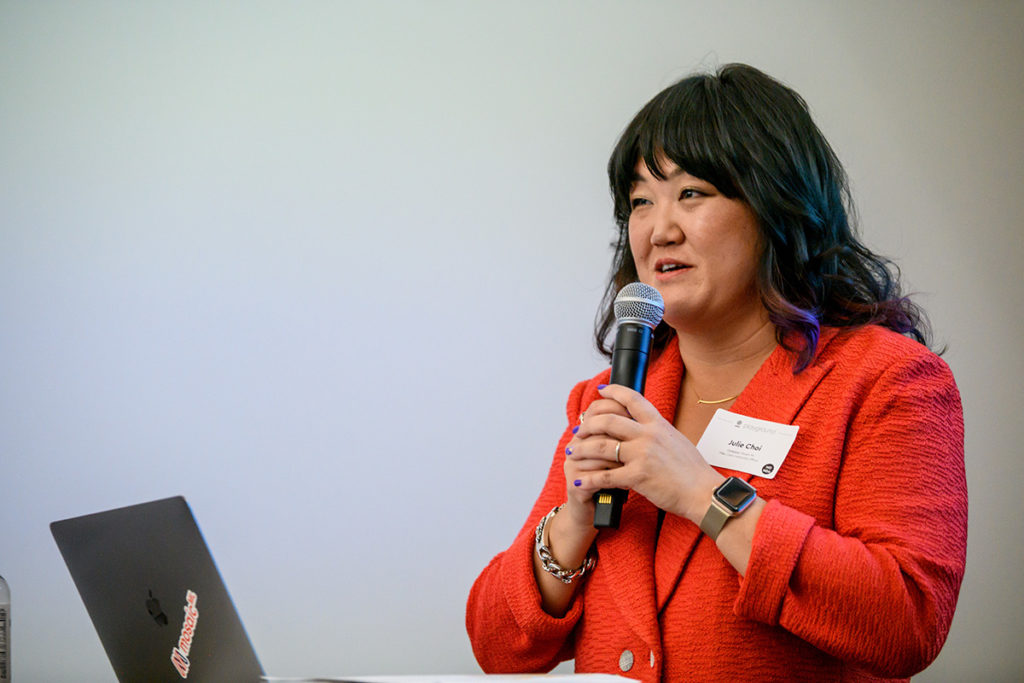
MosaicML VP and Chief Growth Officer Julie Choi welcomes the audience. She emcees the evening at MosaicML Girl Geek Dinner. (Watch on YouTube)
Angie Chang: The first event was 2008.
Julie Choi: 2008. Yes. And tonight we have amazing speakers to share with us about machine learning, about engineering, about diversity, and how that can really supercharge productivity in high growth organizations and machine learning research just from some of the world’s best AI companies and organizations.
Julie Choi: Our first speaker of the evening is my dear colleague at MosaicML, Laura Florescu. Laura and I met a little over a year ago. You greeted me at the front door. You were the first face I saw at MosaicML. She has just been an inspiration to me as an ML researcher at our company. Prior to joining MosaicML, Laura actually worked at several unicorn AI hardware startups. Then prior to that, she got her PhD from NYU in mathematics and is just a brilliant lady. Laura, can you come up and tell us about this amazing topic?
Laura Florescu: Thank you.
Julie Choi: Let’s give her a hand.
Laura Florescu: Can you hear me? Hi, thank you so much everybody for being here. Thanks to Julie, Sarah, Angie, Playground, for having this event. Very honored to be here.
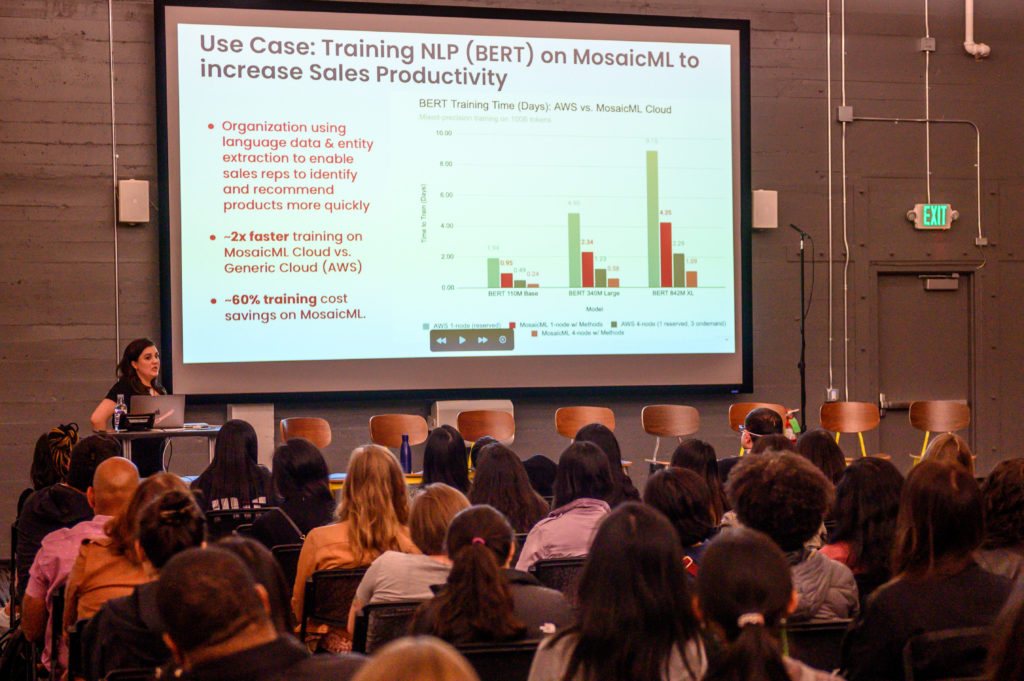
MosaicML AI Researcher Laura Florescu talks about making ML training faster, algorithmically with Composer and Compute, MosaicML’s latest offerings for efficient ML. (Watch on YouTube)
Laura Florescu: As Julie said, I’m a researcher at MosaicML. A little bit about myself. I’m originally from Romania. I came to the states, did my undergrad in math, did a PhD at NYU, and then I got the kind of Silicon Valley bug. And now I’m at MosaicML. And so what we do is develop algorithms and infrastructure to train neural networks efficiently.
Laura Florescu: Basically for the people in the audience who are not into ML, training neural networks is at the core of artificial intelligence. It uses a lot of data. It’s applied to many different fields with image, language, speech, and kind of like a takeaway from this is that, for large powerful models, the training costs for one single run can get in the millions of dollars, to train one such model. And in order to get to a really good model, you need to do several such runs. So the cost can get extremely, extremely expensive.
Laura Florescu: Our belief at MosaicML is that state of the art, large, powerful models should not be limited to just the top companies. As we have seen over the last few years, the costs are getting larger and larger due to both the size of the models, also the data that the models ingest is just exploding.
Laura Florescu: A couple of years ago, a state-of-the-art model, Megatron, actually cost $15 million to train. As you can imagine, startups probably cannot really train models like that. And at MosaicML, this is our belief, that this kind of training should be accessible to other partners as well.
Laura Florescu: That’s where we come in. We want the state-of-the-art efficient ML training. Our co-founders are Naveen and Hanlin from Nirvana and Intel AI. Also, founding advisors from MIT and also founding engineers from leading AI companies. All of us have the same kind of goal to train machine learning basically faster and better and cheaper.
Laura Florescu: Our thesis is, the core of Mosaic is that algorithmic and system optimizations are the key to ML efficiency, right? And then the proof to that so far, is that we are working with enterprises to train ML models efficiently. And we want to enable our organizations to train the best ML models, the cheapest and the most efficient.
Laura Florescu: Some results that we already have. As I said, we want to be agnostic about the kind of models, data that we ingest. For some image classification tasks, we have shown 6x speed ups, like 6x cheaper, faster than like regular training. About 3x faster for image segmentation, about 2x for language models, language generation, and 1.5x for language understanding. We have been around as Julie said for about a year, a year and a few months, and these are already some of the results that we have achieved.
Laura Florescu: A use case is to train NLP models, such as BERT, for those of you who know that, and on our specific platform and without algorithms. Use case is for example, to increase sales productivity. If you see there, on our MosaicML 4-node, with our speed ups, which I’m going to discuss in a little bit, we can see up to 2x speed ups of training such models. And also about 60% training costs reduced by training with both our algorithms and on our platform, on our cloud.
Laura Florescu: The MosaicML cloud, we want it to be the first AI optimized cloud designed specifically for AI and directly to reduce training cost at any layer of the stack. For example, in the training flow, we want to reuse data from past runs. In the models that we’re using, so that’s where the kind of optimized model definitions come in, Composer, which is our open source library. We are doing the algorithmic speed ups, the training. And kind of like at the lower level, we want to also be able to choose the best hardware in order to get to the lowest training cost. At each layer, we are optimizing all of these system optimizations and composing all of those basically leads to the best training runs.
Laura Florescu: As I mentioned, Composer is our open source library. We have a QR code there if you want to check it out. We have about 25+ algorithms that we have worked on and given the name, they compose together, and that’s how we achieve basically the best, 2x to 5x speed ups. And as an example, for a BERT model, we have seen 2.5x speed up for pre-training, which as you can see, goes from nine days to about three days. That’s a huge win. Check it out if you would like. As I said, open source, so we’re always looking for feedback and contributions.
Laura Florescu: We’re open to partnering with any kind of corporate users, for anybody who has vision or language tasks, and then also industry, we want to be industry agnostic and global. Again, we want to optimize basically any kind of models. And as I said, the open source Composer speed up, we’re open to feedback and partnerships for that as well. And of course we are hiring. Yeah, it’s a really great team, really fun, really ambitious. And I’m so honored to work there and we’re looking for all kind of builders, engineers, researchers, products. And thank you very much.
Julie Choi: Thank you so much, Laura. Okay, that was wonderful. Let me just go to the next talk. Our next speaker is Amy Zhang, and Amy comes to us from, she’s currently a research scientist at Meta AI research, and a postdoc I think, you’re not a postdoc anymore, are you?
Amy Zhang: It’s kind of like a part-time thing.
Julie Choi: It’s like, never ending, huh? But you’re on your way to your assistant professorship at UT Austin, amazing, in Spring 2023. And Amy’s work focuses on improving generalization and reinforcement learning through bridging theory and practice. And her work, she was on the board most recently of women in machine learning for the past two years. And she got her PhD at McGill University and the Mila Institute and prior to that obtained her M.Eng. in EECS and dual Bachelors of Science degrees in math and EECS at MIT. Let’s welcome Amy Zhang to the stage. Thank you.
Amy Zhang: Thank you Julie for the really kind introduction and for planning this amazing event. It’s so nice to see people in real life. This is my first large in-person talk in over two years so please bear with me.
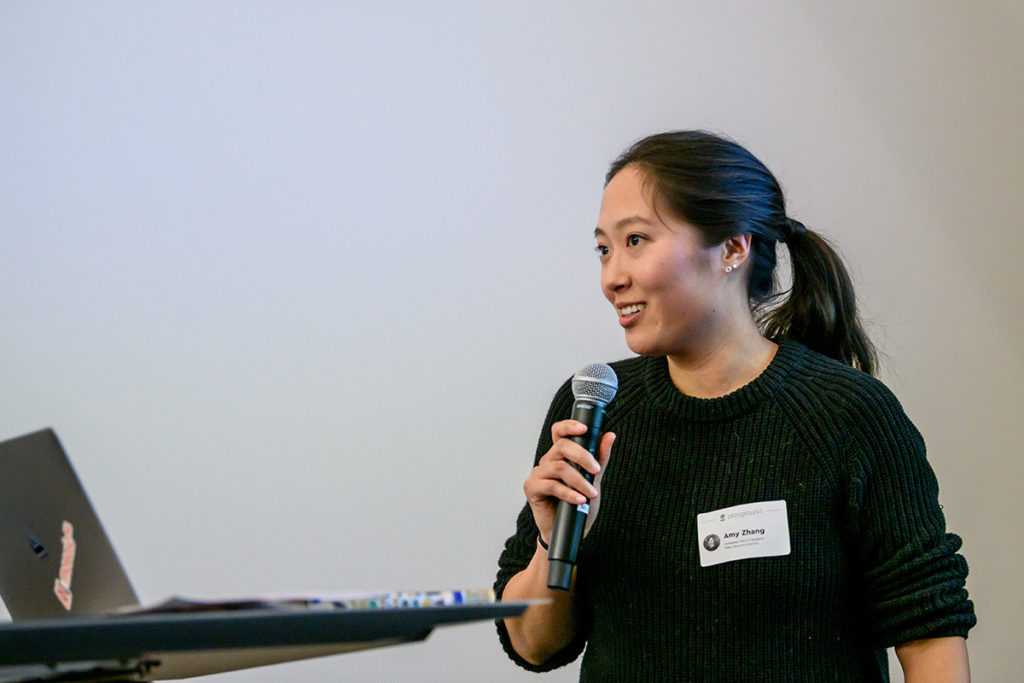
Meta AI Research Scientist Amy Zhang speaks about her career journey in reinforcement learning, from academia to industry, at MosaicML Girl Geek Dinner. (Watch on YouTube)
Amy Zhang: Today I’ll be talking about my research which is in reinforcement learning, but I first wanted to just give a little bit of introduction of myself. To me, I feel like I’ve had a fairly meandering journey through academia and industry and research in general. I wanted to give a little bit of introduction of what I’ve done so that if any of you feel like you’re going through something similar, please reach out and I’m happy to chat and give more details. Like Julie said, I did my undergrad at MIT, a year after I finished my masters, I started a PhD at UCSD. It did not go well, through no one’s fault, really. I just felt really isolated and so after about a year, I quit my PhD, meandered through a couple of startups, and then eventually found my way to Facebook, which is now Meta.
Amy Zhang: At Facebook, I initially started on the core data science team. I was a data scientist, but I was working on computer vision and deep learning. This was 2015, still fairly early days in terms of deep learning and everyone is really excited about the gains that it had shown for computer vision at the time. I was working on population density, so we were taking satellite images and doing building detection to find houses, to figure out mostly in third world countries, what the population density was so that we could provide internet to people and figure out what was the best way to provide that internet.
Amy Zhang: After about a year of that, I ended up joining the Facebook AI research team FAIR, as a research engineer. And after about a year of that, I happened to meet the person who became my PhD advisor, Joelle Pineau, who is now director of FAIR, and I got to do my PhD with her at McGill University while still staying in FAIR. Fast forward a few more years and I defended my PhD remotely in the middle of the pandemic last year and am now back in the Bay Area as a research scientist at FAIR and like I said, was part-time postdoc-ing at UC Berkeley.
Amy Zhang: After spending all this time in industry, and having a really great time in industry, and getting all of these amazing opportunities to do my PhD while in industry, with all of the nice resources that that provides, I decided to go back into academia. And, last year I was on the faculty market, on the academic faculty market, and I accepted a position at UT Austin. I will be starting there next year as an assistant professor.
Amy Zhang: With that, I’m just going to jump straight into my research. And this is going to be, again like I said, a very whirlwind, high level overview. I’m passionate about reinforcement learning. I love the idea of agents that can interact with the world, with us, and it can grow and learn from that interaction.
Amy Zhang: Okay, thinking a little bit about what reinforcement learning (RL) can do. I’m personally really excited about the idea of applying reinforcement learning to solve real world problems. To me, this is personalized household robots, having a robot that will do your dishes, clean the house, make your bed, learned autonomous driving so you can just drive in a car without having to actually drive and pay attention to the road, and personalize healthcare, so having like a robo-doctor who knows everything about you and can personalize healthcare and give recommendations for you specifically.
Amy Zhang: Unfortunately we are not there yet, as I think maybe all of us can tell. Deep reinforcement learning has had a lot of successes in the last two years. Maybe some of these things are familiar to you. AlphaGo, where we have an RL agent that was able to beat the best human experts. OpenAI with playing video games, which I’m not very familiar with, but like Dota and StarCraft. These are the things that have been hitting the news in terms of what Deep RL is capable of. But there are still a lot of disappointing failures and I think none of these videos are going to show, but imagine this robot trying to kick this ball and then just falling flat on its face. That’s what that video is. And in the other little cheetah looking thing is supposed to be like tripping and falling. Anyway, this is where we are currently with RL.
Amy Zhang: Why do we still see this discrepancy? How are we getting these amazing gains but still seeing these failures? And what we’re really seeing is that Deep RL works really well in these single task settings, in simulation, when you have access to tons and tons of training data, but it works less well in visually complex and natural settings. Basically we’re not seeing the same type of generalization performance that we’ve been getting out of deep learning in computer vision and natural language processing. My research agenda is mainly about how can we achieve RL in the real world? How can we solve these problems? And, to me, it seems that abstraction is one key to generalization. And I use this type of idea to develop algorithms that have theory-backed guarantees.
Amy Zhang: I’m going to not really talk a little bit about this math, but I’m particularly interested in being able to train reinforcement learning agents that can solve tasks from pixels. Imagine that you have this household robot or this autonomous driving car that is receiving information about the world through a camera, through RGB input, and that’s a big part of how we also perceive the world. There are things in this image that are relevant for the autonomous driver here and there are things that are not. And we want to figure out how can we determine from just a reward signal, what things are relevant versus irrelevant.
Amy Zhang: I’m just going to, as part of this project, we developed this representation learning method using this idea by simulation, and showed that in this type of simulation driving task, which is done in this platform called Carla, so we have just this figure eight simulation environment where this car is just driving along this highway, and there’s lots of other cars in the road and basically, it’s designed to try and drive as quickly as possible. Using the break as little as possible and maximizing throttle while also not hitting anything. And we find that our method which can basically ignore these kind of irrelevant details and figure out what things are irrelevant does much better compared to a lot of existing methods.
Amy Zhang: One really cool thing is that when we look at the representation that we actually learn here, and we look at what kind of observations get mapped to be close together in this representation space, so what information is actually getting captured by this representation, we see that… this is the agent’s point of view. We see that, in these three examples, you’re always on this straight road where you have an obstacle on the right hand side. It doesn’t matter what the obstacle is, but the representation just captures that something is there, which means that you can’t turn right. This is just kind of an example of what our algorithm can do. And unfortunately I’m going to skip over this, because these were just some videos showing what our agent can do.
Amy Zhang: I just wanted to end on talking about some open problems that I’m particularly excited about. I’m particularly excited about compositionality. How can we solve combinatorially difficult problems. And these correspond to a lot of real world tasks that we should care about. When we think about really simple versions of problems like this, you can have a block stacking task. You can have any number of blocks or any combination of blocks and so you can always have new environments that you give to your agent to try and solve.
Amy Zhang: Similarly, moving more towards actual real world problems that we care about. Again, going back to the dishwasher example or an agent that is trying to move boxes around in a warehouse. These are all settings where the exact environment, the exact state that the agent has to deal with, is constantly different. The objects that you want to place in your dishwasher on a day to day basis is always going to look different. How can we get agents that can actually generalize to all of these new states?
Amy Zhang: I think one really exciting direction to go when trying to solve this type of problem is to think about factorization. How can we break down a problem into smaller, easier building blocks? So if we understand how one block, the dynamics of one block moves, so creating a stack of two blocks, right? Babies play around with this sort of thing and then as they get older, they automatically can extrapolate to building like gigantic towers and castles. So how can we take that idea and give it to reinforcement learning? So this is something that I’m particularly excited by.
Amy Zhang: I just wanted to end talking about my sort of wider research agenda. Now that I’m starting as faculty, I have to start recruiting a group. If any of you are interested in doing a PhD at UT Austin or know anybody who is, please send them my way. But when I think about what my research group does, I’m particularly interested in these three applications of reinforcement learning.
Amy Zhang: The first is in robotics, trying to solve manipulation tasks. Going back to that block stacking example, trying to solve locomotion and navigation tasks. How can we build an autonomous driving system purely from first principles, like end to end machine learning? Reinforcement learning has to be a part of that.
Amy Zhang: Natural language processing, so using RL for text generation, being able to extract knowledge from text, when you build an interactive agent, how do you give it information about the world? We learn from textbooks, we don’t want an agent just deployed in the real world with no basic information. Healthcare, how do we build RL agents that can help out with diagnosis and treatment and tackle a lot of the problems that we have there? That’s basically it, very grandiose. I probably won’t make much progress on a lot of these fronts, but this is the dream. And thanks for listening.
Julie Choi: Thank you so much. I think this is very grandiose and amazing that you’re working on it. Amazing. Thank you, Amy, okay.
Julie Choi: We’re going to just clean some of this up. Okay. And we’re going to open up our next talk, which I’m extremely excited about. Let me introduce our next speaker. Our next speaker journeyed probably the furthest to join us tonight… all the way from the east coast, North Carolina, to be here today to deliver this talk. I want to thank you. Thank you.
Julie Choi: Tiffany Williams is a Staff Software Engineer at Atomwise working on AI-powered drug discovery. Prior to Atomwise, Tiffany was a Staff Software Engineer at Project Ronin where she was developing cancer intelligence software. Tiffany earned her PhD in cancer biology from Stanford University and her Bachelor’s in biology from the University of Maryland. Let’s give a warm welcome to Tiffany Williams.
Tiffany Williams: All right. Hello, everyone. I’ll admit, I have some notes here so I can stay on track, but I’m really glad to be here this evening. I was searching through my inbox and realized that I attended my first Girl Geek Dinner in April of 2015. I was fresh out of grad school and a coding boot camp. Eager to form connections, acquired some cool swag, and to be honest, eat some free food and have some drinks.
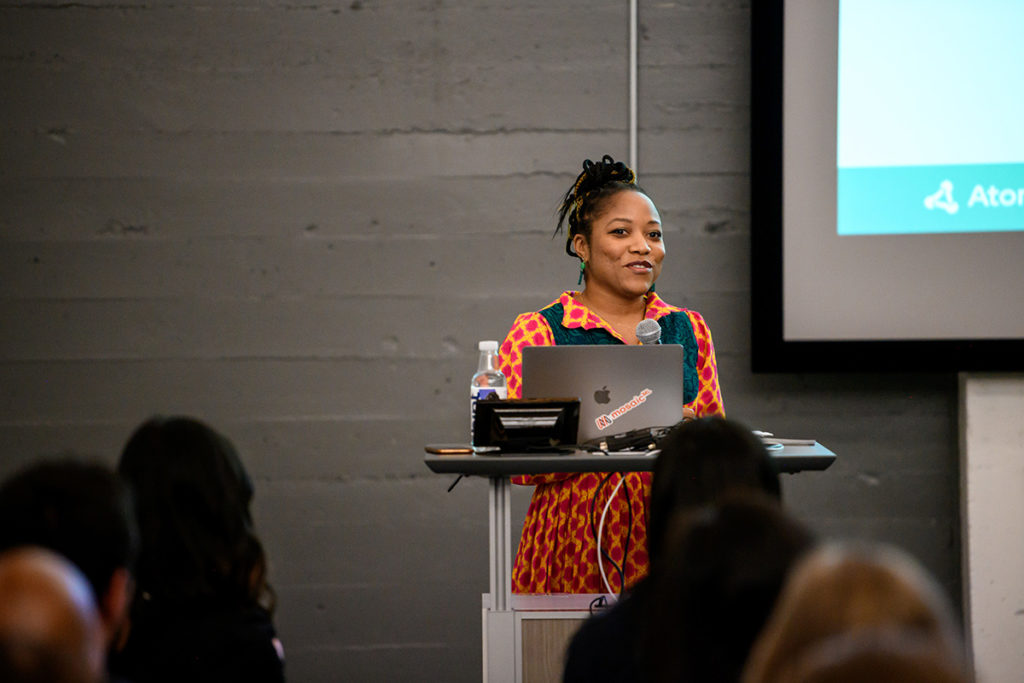
Atomwise Staff Software Engineer Tiffany Williams discusses the drug discovery process with AtomNet at MosaicML Girl Geek Dinner. (Watch on YouTube)
Tiffany Williams: I went to grad school to study cancer biology. My research was from the perspective of a molecular biologist exploring the role of a protein as a target for therapy, and skin cancer. And my interests have generally been at that sweet spot, leveraging data, and technology to improve human health. Coming from a biology research background, in my current role as a software engineer at Atomwise, I’m able to look further down that drug discovery process. I’m happy to share with you all today, what that looks like.
Tiffany Williams: Now, I’ll start by giving a very brief primer on the current state of the drug discovery process, as well as a very brief primer on biochemistry. From there, I’ll talk about what we’re doing at Atomwise to make a significant impact in human health, and some interesting challenges ahead of us. I do want to give a disclaimer that I am merely scratching the surface of what could be discussed in drug design, drug development, and even applying ML on top of that. But what I hope you all take away from my time with you all this evening is just general excitement about the possibility of improving human health.
Tiffany Williams: Beyond that, I hope you feel empowered to do even more digging into the drug discovery process, and maybe you’ll feel empowered to even… to find opportunities to make an impact in that space. For me personally, I’m coming from the east coast, but I have a personal attachment to improving the drug discovery process. I’m on the east coast serving as a caregiver now – my mom has endometrial cancer and it’s at a point where there’s only one treatment option. If any of you have ever been in, unfortunately, in a predicament like that, it sucks.
Tiffany Williams: There’s a lot of data out there, technology’s improving, and it would be great if we can leverage that to improve health, right? This diagram depicts the drug discovery process from the basic research to identify a potential drug target all the way to FDA approval. On the right, I’ve noted the average number of years of the different stages in the drug discovery process. In the middle, what it shows are like basically in these different stages, there are certain types of experiments that are done that basically kind of knock out the potential candidates in that step.
Tiffany Williams: Initially you might have like a candidate pool of over like 10,000 drugs, but in an early stage, which is known as like kid identification, computer simulation is used to predict potential drugs ability to bind to a target of interest. These subsequent steps will test for other characteristics like potential toxicity, or efficacy in cell cultures, and animal models. Eventually, hopefully, we end up with a few candidates that reach the human clinical trial phase to verify safety, and any other side effects along with a few other things.
Tiffany Williams: What I hope that you take away from this slide really is that the current basic research to FDA approval drug process takes a really long time. It takes on average, it’s estimated to take about 15 years, and it’s also really expensive for each drug that goes to market, it’s estimated that 2.6 billion is spent.It’s the case that not all of these trials have a happy ending. For every drug that makes it to the market, millions may have been screened, and discarded. We have to improve this process, right? But in order to appreciate how this process can be improved, let me first give that very, very, very brief biochem primer. And I’ll focus specifically on protein interactions.
Tiffany Williams: Up to this point, and then even later in this talk, I’m going to be using some words interchangeably, and I just want to make sure I bring some clarity to what I’m actually talking about. When I say like ligand, or ligand, I’m specifically referring to any molecule that combined to a receiving protein, and that receiving protein is also known as a receptor. And in this presentation, when I use the word or phrase drug candidate, I’m actually referring to that ligand and the receptor would be that the drug target.
Tiffany Williams: One model for how proteins interact is this lock and key model. And the gist of this is that the ligand and the receptor have these somewhat complimentary sites. And the ligand combined to this complimentary site or active site, and basically alter the shape, and or activity of the receptor. And one more thing is that this binding is also referred to as docking. If we know our bodies are made up of proteins, and they have a diversity of functions within the body, but in a disease state, a normal process can become dysregulated. This image on the far right, is taken from a classic cancer biology paper called The Hallmarks of Cancer. The premise of this is that there about 10 biological capabilities that cells take on as they like morph into this more cancerous state. And I’m not going to go over all of these, but I want to highlight that two of these biological capabilities would be like cell growth and like cell motility.
Tiffany Williams: Those are normal functions within the body. But I guess within a diseased state you’ll have overgrowth, or you may have cells that are primarily concentrated in one area, develop the capability to invade into other tissues or metastasize. I say all this to say that like in small molecule drug discovery, what we want to do is actually figure out what sort of structure is needed for a ligand to bind to this problematic protein or proteins, and counteract that like not so great behavior. If this is where Atomwise comes in, right? Or it does, if you don’t know. We want to know how can we efficiently explore this space of all potential chemical compounds to better identify small molecule drugs faster.
Tiffany Williams: Atomwise actually developed AtomNet the first deep convolution neural network for structure based drug design, so that we can actually make better predictions for potential drug candidates earlier in the drug discovery process and faster. I’m highlighting this paper, again, just trying to give high level overviews. Feel free to check this paper out, but what I want you to take away from this is that the AtomNet technology is currently being used in real drug research, in cancer, neurological diseases, various spaces, on the right is, it’s actually a GIF, but since it’s a PDF, it’s not showing up as a GIF, but basically this GIF would show the AtomNet model. It would simulate the AtomNet model, predicting candidate treatments for Ebola. And this prediction that AtomNet made is actually has led to candidate molecules that are now being studied in animal models.
Tiffany Williams: One, despite everything I’ve said up till now, you actually don’t need a background in wet lab research, or chemistry to appreciate what’s happening here. The power of convolute or the power of convolution neural networks, or CNN, is that it allows us to take these complex concepts as a combination of smaller bits of information. And I think if you’re familiar with CNN, or even if you don’t like one area that is really popular, has been computer vision.
Tiffany Williams: I’ll briefly go over like an example of image recognition, and then kind of like try to tie it into how AtomNet works. An image is essentially represented as a 2D grid with three channels, you have red, green, and blue. And this network learns images of objects or faces, for example, by first learning a set of basic features in an image like edges. Then, from there, by combining those edges, the model can then learn to identify different parts of that object. In the case of a face that might be ears, eyes, nose, et cetera. With AtomNet that it’s working in a similar fashion, that receptor ligand pair is represented in a 3D grid, and the channels are essentially the elements that make up protein like carbon oxygen, nitrogen, et cetera. In the case of AtomNet, that the learning of edges is actually the learning of the types of, or predicting the types of bonds between those elements.
Tiffany Williams: Then from there, the compliment to the ear eye detection would be actually identifying more complex molecular bonds. You could say, essentially, that this network is like learning organic chemistry 101. This is powerful because we can then train these models to make predictions about different aspects of the drug discovery process. Like what ligands, or what type of structures are most likely to bind to a certain target? At what strength? What are the additional modifications that we can make to a potential drug candidate to strengthen that bond? Beyond that, it’s not enough just for the ligand to bind to the receptor, it also has to be like biologically relevant in that, let’s say, if we’re looking for something that is treating some neurological disease. We need that ligand to be small enough to cross the blood brain barrier. Or we may need to take into account toxicity or other effects that may happen in the body. Metabolism. We don’t want that small molecule to become quickly metabolized in the body before it has the opportunity to have the intended effect.
Tiffany Williams: These are exciting times, and I’m really, really passionate about the work that we’re doing at Atomwise and any work that is being done at the intersection of health and technology. I wanted to briefly go over some of the projects that my team is currently working on. I work on the drug discovery team at Atomwise, it’s within the engineering team. I think we’re working on some pretty interesting issues. One of my, my teammates Shinji, he recently has been working on bringing best engineering practices, and improving performance of some of our ML tools. Adrian, my teammate, is working on optimizing algorithms to explore a three trillion chemical space. He is also been working on, or has been able to create simulating mocular… mocular, I’m combining words… molecular docking on GPUs.
Tiffany Williams: Then another thing that we’re working on that I actually have more of a hand in, is building a research platform to better enable drug discovery. Oh, I didn’t mention the third person Shabbir, who’s our manager, and he has his hands in a bit of everything. What I hope you take away from this is that I think we’re at an exciting time in today to like really leverage data, and technology to make a major impact in human health. I think there are a lot of challenges, interesting challenges in drug discovery. I hope that you may have been convinced that you actually don’t need a background in chemistry to contribute. There are a lot of transferable skills. If you just know software engineering, or you know ML, or if you’re in product or marketing, there is a place for you in this space.
Tiffany Williams: Finally, really important takeaway is that we are hiring. My team alone, please, if you have any front end experience, if you have backend experience, or if you have a background in computational chemistry, those are some of the positions that are open right now on my team, but then outside of my team, we also are hiring. Definitely check out our careers page. If you have any more questions or are interested in chatting, feel free to reach out to me. I have my LinkedIn handle as well as my Twitter handle, posted here. Then finally, I think I mentioned that I had some references to share. Again, I’m only scratching the surface. There’s so much information out there. I wanted to highlight two Medium articles that were written by former Atom, which is what we call people that work at Atomwise, machine learning for drug discovery, in a nutshell, I highly recommend starting there if you want to do a deeper dive. That’s it.
Julie Choi: Thank you so much, Tiffany. Yes. I think drug discovery is an incredible application domain for deep learning. Really appreciate your talk. Okay, let me introduce our next speaker. Our next speaker is Shelby Heinecke. Shelby is a senior research scientist. Again, I did not touch. Okay.
Julie Choi: Shelby is a Senior Research Scientist at Salesforce Research, developing new AI methods for research and products. Her work spans from theory-driven, robust ML algorithms to practical methods, and toolkits for addressing robustness in applied NLP and recommendation systems. She has a PhD in Mathematics from the University of Illinois at Chicago, and a Master’s as well in Math from Northwestern and her bachelor’s is from MIT. Let’s welcome Shelby.
Shelby Heinecke: Thanks so much. Awesome. I have to give a thank you to Julie for including me in this event, inviting me. This is my first Girl Geek, and not my last Girl Geek event. I’m super excited to be here. Yeah, let me get started.
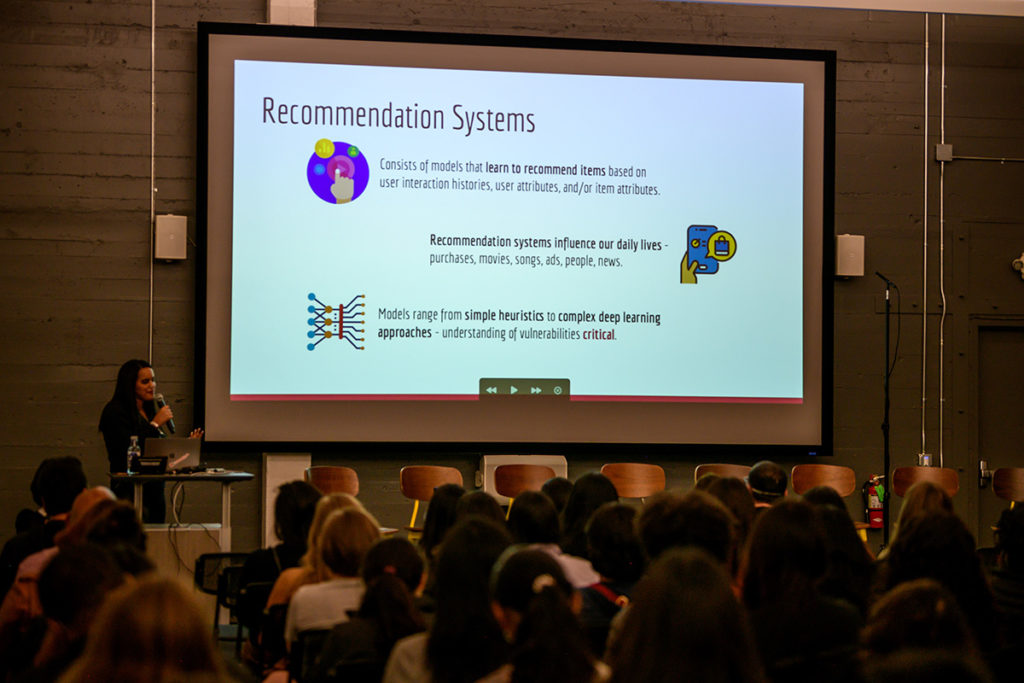
Salesforce Research Senior Research Scientist Shelby Heinecke speaks about how to evaluate recommendation system robustness with RGRecSys at MosaicML Girl Geek Dinner. (Watch on YouTube)
Shelby Heinecke: Today I’m going to be talking about evaluating recommendation system robustness, but first I feel kind of like the new kid on the block. Let me just give a little bit of background about myself. I moved here to the Bay Area about a year and a half ago in the middle of the pandemic. Super excited to be here in person to meet people.
Shelby Heinecke: Currently I’m a Senior Research Scientist at Salesforce Research. As Julie mentioned, I work on both research and product. It’s pretty awesome to develop prototypes, and see them in production. Before I was here at Salesforce in the Bay Area, I was doing my PhD in math in Chicago. There, I focused on creating new ML algorithms that were robust. I worked on problems in the space of network resilience. Before that I got my master’s in math, kind of focusing on pure math at Northwestern. Originally I hail from MIT Math, focusing on pure math there. That’s my background. Today’s talk recommendation systems and robustness.
Shelby Heinecke: Let’s get started. A crash course and recommendation systems. So, what is a recommendation system? Well, it consists of models that learn to recommend items based on user interaction histories, user attributes, and or item attributes. Let me give you an example, say we want to build a recommender system that recommends movies to users. Well, what kind of data can we use? We’re going to use the users, previous movies. They viewed we’re going to use the ratings that they rated those movies.
Shelby Heinecke: We’re probably going to take a look at the user’s age. We’re going to look at the user’s location. We’re also going to take into account item attributes. The movie attributes, like the movie title, the movie genre, we’re going to take all that. That’s all of our data, and we’re going to train models. The models can build the recommendation system. As you can imagine, recommendation systems influence our daily lives. We’re all exposed to recommendation systems every day. Just think about purchases. Think about movies.
Shelby Heinecke: Think about songs you’re recommended. Think about the ads that you see every day, people, news, information. We are at the mercy of these recommendation systems and a lot of our decisions are highly influenced by what the recommendation systems decide to show us. Let’s think about the models that we see in recommendation systems. Models can range from simple heuristic approaches, like a rules based approach or co-sign similarity approach to complex deep learning approaches, think neural collaborative filtering, or even now we’re seeing transformer based approaches coming to light.
Shelby Heinecke: With the vast range of models available and how greatly they impact our daily lives, understanding the vulnerabilities of these models of each of these types of models is super critical. Let me get started about recommendation model robustness. As we all know, machine learning models are trained on data, and ultimately deployed to production. And in that process, there are some hidden sources of vulnerabilities that I want to bring to light.
Shelby Heinecke: One of the big issues is that training data may not reflect the real world data. In many cases, we’re training on data that’s been highly curated. That’s been cleaned up. And yeah, and so because of that, when we train a model on that very clean, highly curated data, it’s not going to necessarily perform well when it’s exposed to the messy data of the real world. Real world data has noise. Real world data is just can be unpredictable. As a result, sometimes we train model, we train a recommendation model, but we see poor performance and production. That’s definitely one type of vulnerability we need to watch out for.
Audience Member: Woo! So true.
Shelby Heinecke: What? Okay. Another type of vulnerability. I love the enthusiasm, okay? Another type of vulnerability. As you can imagine, recommendation systems are closely tied to revenue for a lot of different parties for companies, for sellers. There’s an issue that participants may intentionally manipulate the model. Think about creating fake accounts, trying to do things, to get your item higher on the list for customers, things like that. That is a reality and that’s something we have to take into account.
Shelby Heinecke: The last thing I want to bring to light is poor performance on subpopulations. This is a well known issue across all of ML, but I just wanted to bring it to light, to recommendation too, that when we train models and we test on the evaluation set, usually the basic evaluation methods think precision recall F1. We’re computing that on the entire test set, so we’re averaging overall users. And in that average, sometimes we’re hiding…
Shelby Heinecke: Sometimes there’s poor performance on subpopulations that are hidden. For example, a subpopulation that you may care about could be new users, or maybe a users of a certain gender. That’s something that we just need to keep an eye on. We don’t want poor performance on key subpopulations I’ve told you about all these different types of vulnerability.
Shelby Heinecke: What is a robust model? Well, a robust model you can think of it intuitively as a model that will retain great performance in light of all of these potential perturbations, or in light of all of these scenarios. The question is how can we assess the robustness of models? That is where one of our contributions comes in.
Shelby Heinecke: I want to introduce one of our open source repos called RGRecSys, and it stands for robustness gym for recommendation systems. Our library kind of automates stress testing for recommendation models. By stress testing, I mean, you can pick a model, you can pick a data set, and you can stress test it in the sense that you can manipulate the data set.
Shelby Heinecke: You can add in attacks, you can add in noise and so on. I’ll actually go into more detail about that. And you can see in a really simple way how your model, the robustness of your model. As I mentioned, RGRecSys, is a software, is a software toolkit. It’s on GitHub. I’ll talk about that in a second and it’s going to help you assess the robustness of your models.
Shelby Heinecke: One thing that we contribute is that RGRecSys provides a holistic evaluation of recommendation models across five dimensions of robustness. When I say I told you about various types of vulnerabilities, there’s various types of robustness. Our library helps you to quickly and easily test all these different types of robustness for your model. Using our API, you just simply select a model for testing, and then you specify the robustness test that you’re interested in trying along with the robustness test parameters. What I’ll do is I’ll go over the types of tests that are in the library.
Shelby Heinecke: Let’s talk about the different tests that we have in our library. First is around subpopulations and this kind of goes back to what I mentioned in the previous slides. This will allow you the test. What is the model performance on subgroup A versus subgroup B? And this is going to be very useful because, as I mentioned, most of the time, we’re just computing precision recall these usual metrics on the test set, but this gives you an easy way to test performance on specific subgroups. This could be useful, for example, if you want to test you want to test performance on gender A versus gender B, or new users versus old versus like users that have been in the system for longer time. Just some examples there. That is one type of test you can run in our library. The second type of test is around sparsity. If you think about recommendation systems and you think about the items available like a movie recommender, or a purchasing recommender there’s millions of items, and each user really only interacts with a handful of those items.
Shelby Heinecke: Each user is only clicking, only purchasing, only viewing a handful. This is a source of data sparsity. Data sparsity is a huge problem in recommendation systems. It will be good to test the degree to which your model is sensitive to sparse data. That’s one thing you can test with our library. The third test is around transformations. There are a lot of ways that data can be perturbed when you’re training a recommendation system model. For example, maybe you’re gathering data about your users and maybe that data is erroneous in some ways. And because of that, you might want to test if a recommendation model will be robust, if user features, for example, are perturbed. The fourth test that you can test is around attacks. As I mentioned, there’s a lot of reasons why people would try to attack a recommendation system it’s tied to revenue ultimately.
Shelby Heinecke: What you can do with our library is implement some attacks and test how your model performance would change under those manipulations. And finally distribution shift. What we see is that the training data that you train on is often different from the data that you’ll see in production. It’s super important to be able to know, get a sense of how was my model going to perform if it’s exposed to data from a slightly different distribution? You can go ahead and test that with our library., I definitely encourage you to check out our library on GitHub, and feel free to check out the paper for way more details about the capabilities. And with that, thank you so much for listening. It was great to share it. Feel free to reach out.
Julie Choi: Thank you, Shelby. That was great. It’s very important to be robust when we’re doing model deployment. Okay. Angela Jiang, thank you so much for joining us tonight.
Julie Choi: Angela is currently on the product team at OpenAI. Previously, she was a product manager at Determined AI building, deep learning training, software and hardware, deep learning… I think Determined AI was recently acquired by HPE. And Angela graduated with a PhD in machine learning systems from the CS department at Carnegie Mellon University. And we actually have built some of our own speed up methods on your research. It’s an honor to have you here today.
Angela Jiang: Thank you so much, Julie, for inviting me, for the introduction, as well as Sarah, and Angie for organizing the event and bringing us all together too. Yeah. Like Julie mentioned, I’m Angela. I work on the product team at OpenAI.
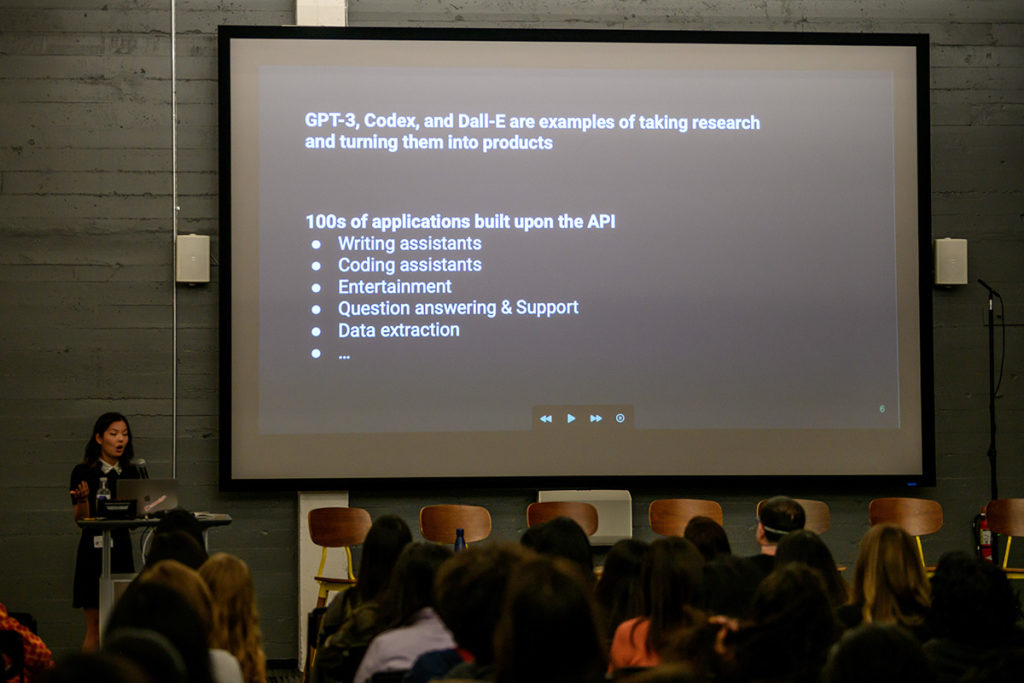
OpenAI Product Manager Angela Jiang speaks about turning generative models from research into products at MosaicML Girl Geek Dinner. (Watch on YouTube)
Angela Jiang: I work on our Applied team where we really focus on essentially bringing all of the awesome research coming out of the org and turning them into products that are hopefully useful, safe, and easy to use.
Angela Jiang: Most of my day, I’m thinking about how to turn generative models from research into products. I thought I’d make the talk about that as well. This might as well be a list of things that keep me up at night, think about it that way. But in particular, what I really wanted to highlight is just some of the observations I’ve had about things that make these generative models unique and tend to have large implications on how we actually deploy them into the market.
Angela Jiang: To start, I want to share a little bit about what OpenAI’s products are. OpenAI does a lot of AI research. In particular, we do a lot of generative models. Over the last two years, we’ve really started to work to bring those generative models into real products. Three examples here are GPT-3, that does text generation; Codex, that does code generation; and most recently, DALL-E, which does image generation. These were all made by DALL-E.
Angela Jiang: To get a little bit more concrete, what these products are is that we expose these models like GPT-3 and Codex via our APIs. As a user, you can go to the OpenAI website, sign up, and then hit these endpoints and start using these models. And here’s an example of how you might use the GPT-3 model. Here we have an example of your input in the gray box. It might say something like, “Convert my shorthand into a firsthand account of the meeting and have some meeting notes.” And you submit this to the model, and then the model will do its very best to return you an output that completes this text. And in this case, the response is essentially a summarization of your notes.
Angela Jiang: Codex works very similarly, except it’s also specialized for code. And in this case, our input is not only text, but it’s also some code, some JavaScript code, and some hints that we want to transform it into Python. And the output is some Python code that does that. DALL-E is very, very new. It’s been out for around a month, so it’s not available in the API or anything yet. But it has a very similar interaction mode where you have, again, an input, which is a description of the image that you want. And then as the output, it provides some images that relate to that description, which in this case is a cute tropical fish.
Angela Jiang: I think GPT-3, Codex, and DALL-E are all really good examples of taking research and seeing that there’s a really big market need for the kinds of capabilities that they expose. Then working as an Applied team to actually transform that research into a productionized product by essentially figuring out where that user value is, designing the product so that we can deliver that user value in a way where users are set up for success, making sure it’s deployed responsibly, et cetera, et cetera.
Angela Jiang: To date, there’s hundreds of applications that have been submitted for production review that is built upon this API. Those are applications like writing assistants like CopyAI if you’ve heard of that, coding assistants like GitHub’s Copilot is built on top of Codex, as well as a lot of other applications like chatbots for video games, question answering bots, etc. This is pretty good validation that these models are not only really exciting research, but are also solving problems in the market.
Angela Jiang: Now, when we think about bringing these to market and turning them into real products, I think there are two interesting properties of these generative models that really change who uses them as well as how we actually deploy them. I’ll talk about how these models are stochastic and how they’re very general. With typical products, you might expect that the results are very predictable and deterministic. For instance, if you’re coding up a payments API, every time you use that payments API, you’d expect it to react in a similar way. This is not how our models work at all.
Angela Jiang: Our models are probabilistic. Every time you submit a prompt, you could get a different response back. If you tweak that prompt ever slightly, then you could get a very different response back. That really changes how you interact with a product and what kind of applications can be built on top of it. Another difference is I think that typical software products that I think about are typically focused on solving a need or a problem very directly, whereas, in these generative models, they’re very, very general. They’re capable of doing a lot of things all simultaneously.
Angela Jiang: For instance, you would think that GPT-3 would be able to summarize legal texts and also simultaneously write poems or maybe even code. I think this is super exciting from a science perspective as we get more and more powerful models, closer and closer to something that’s super general. But from a product perspective, this comes with some challenges because we have to now take one model and one product and have it serve many, many use cases simultaneously.
Angela Jiang: For the rest of this talk, I’ll talk about, again, more detail about how these two properties affect the way that we deploy our products, and I’ll give some examples of each. Okay, starting with stochasticity. Right. Our models, again, are probabilistic, which means that every time you use it, you might get a different result. The result might not be what you want or maybe the result is what you want every third time you try the model.
Angela Jiang: It might be surprising that you can actually build real applications on top of this kind of behavior. What we actually find is that for a lot of tasks, especially very simple tasks, we can have very concrete and reproducible behavior for even tasks where the outputs are imperfect or are only correct some of the time, these results are actually still very useful for many applications in the right context.
Angela Jiang: We find that these models work really well, for instance, in creating productivity tools where there’s a human in the loop. A really good example of this is Codex Copilot. Some of you may have used it before, but this is a screenshot of it in action, where it’s a Visual Studio Code extension and it just gives you auto-complete code suggestions that the user can then tab to accept or reject.
Angela Jiang: This is great for a product like Codex because you have actually an expert there that’s telling you, is this completion good or not? And generating more. What we actually see is that most of the suggestions from Copilot are rejected by the user, but still, developers tell us that this is an integral part of their deployment pipeline or their development pipeline I should say. It does not need to be perfect every time we generate something. Those are cases where you don’t need a perfect result every time. What you’re looking for is just inspiration or getting over writer’s block. In some of the applications built on top of these APIs, you actually don’t have a correct answer to shoot for.
Angela Jiang: Applications that are doing art or creative tasks or entertainment, that’s a case where having variety really is helpful actually. Here I have a DALL-E prompt, which is a bright oil painting of a robot painting a flower. And here are different generations from DALL-E. And in this case, it’s really helpful actually to have probabilistic nature because, for one, I want to have different options. And it’s also really cool to have generations that you’ve never seen before or other people haven’t seen before.
Angela Jiang: I think it’s even counterintuitive that, in this case, we’ve actually learned that these AI systems seem to be doing really, really well and surprising us in creative tasks as opposed to rote tasks, which you might have expected the opposite earlier. Those are some examples where the properties of these generative models affect what applications are built on top, but it also affects how we deploy these models. Going into a little bit more detail, one property is that these products are really, really hard to evaluate.
Angela Jiang: This makes being a Product Manager, among other things, quite challenging because we really need to know how our products perform so we can position them in the correct way to the users, know what to deploy, and tell users how to use them correctly. For example, we have these text models, GPT-3, and they have different capabilities, right? One capability is they might be able to complete text, and another capability is they might be able to edit existing text. There’s some overlap there on which one you should use.
Angela Jiang: It’s really important that we understand exactly how this model performs on different tasks so that we can direct users to use the right tool at the right time. We try really hard to figure out creative ways to evaluate these at scale, given that we often need a human in the loop to be telling us if a generation is good or not.
Angela Jiang: We do things like large-scale application-specific A/B testing. Like we see if we use one model or the other, then which model gets more engagement for this writing assistant.
Angela Jiang: Next, I’ll just give a couple of examples of how generality also comes into the picture here. Like I mentioned, these models are capable of a lot of things simultaneously because the way that they’re trained is that they’re trained for a very long time, for months, on a lot of data. And the data spans the internet, books, code, so many different things.
Angela Jiang: By the time we get this model as the Applied team, we’re really still not sure what kind of capabilities it’s picked up at that time. But like I mentioned, it’s really important that we understand that quickly. This is also really exciting because, at any given time, we can always discover a new capability of the model, and it’s often discovered after training.
Angela Jiang: An example of this is that the original GPT-3 model was built with text for text. And it was really a discovery after the fact that it also happened to be kind of good at code as well. And these signs of life and discovery is what ultimately sparked this idea of a Codex series that specialized in code.
Angela Jiang: As a Product Manager, it’s really critical that we keep on discovering and probing these models to really understand what’s the frontier of what they can do. And again, we are still figuring out what is the best way to go about this. But something counterintuitive that I realized after I started is that traditional user research that we’re used to doesn’t actually work really well for this use case because you might expect that you would go to your users and ask, how is this model? What can you do with it?
Angela Jiang: What we find is that our users, by definition, are working on tasks that the model was already really capable at. Their focus is not on the new capabilities of the model and pushing that frontier oftentimes. What’s worked better for us is working with creatives or domain experts to really hack and probe at the model and see what the limits of it are.
Angela Jiang: That’s the exciting part of generality, that there’s always a new capability around the corner to discover. But there’s also some risk to it because there’s not only all these capabilities in the model, but the model can also generate things that are not useful for you, and it can also generate things that you really don’t want to generate.
Angela Jiang: For instance, GPT-3, when prompted in the right way, can generate things like hate speech, spam, violence, things you don’t want your users to be generating. This is also a big part of our goals as a research and applied organization is to figure out how to deploy these models in a way that doesn’t have toxicity in them.
Angela Jiang: There’s a lot of different approaches for doing this, spanning from policy research to product mitigations to research mitigations, but I’ll highlight two things here. One thing that’s worked really well for us is actually fine-tuning these models after the fact with a human in the loop, telling you exactly what kind of content is good content.
Angela Jiang: What we found by doing this is that we have a much better time at having the model follow the user’s intentions. And we have a much less toxic and more truthful model as a result of it. At this point, every time we deploy a model, we also fine-tune the language model in this way. We also do a lot of research and provide free tooling to help the users understand what the models are generating at scale so they can understand if there’s anything that they need to intervene in.
Angela Jiang: Okay. Finally, even though I think it’s very, very cool that these models can do a lot of different things at once, sometimes it really just can’t serve multiple use cases simultaneously well. Different use cases often will just require, for instance, different ways of completion.A chatbot for children is going to want a very different personality than a chatbot for support.
Angela Jiang: You also just have different product requirements in terms of accuracy or latency or even price. Copilot is a really good example of something that needs an interactive latency so that it can continue to be useful in real time. But contrast that with something like SQL query generation, which doesn’t need a fast latency, but actually just wants the most accurate response that you can give it. What we’ve found is that the combination of two things have allowed us to provide this flexibility to serve the use cases that we need to serve.
Angela Jiang: One is that we don’t just expose the best model that we have or the most accurate model we have I should say, we expose models with different capability and latency trade-offs for the users to choose from. And then we also offer fine-tuning as a first-class product so that you can take a model and then fine-tune it so that it has your personalized tone or your personalized data bank.
Angela Jiang: These are, hopefully, examples that give you a flavor of what it’s like to deploy generative models. This is also just the tip of the iceberg. If any of this stuff is interesting, please feel free to come and chat with me. I should also mention that we are hiring. We are. Thank you so much.
Julie Choi: Thank you so much, Angela. After DALL-E was launched, the productivity went a little down. We were so distracted by the DALL-E. I don’t know whether to thank or curse your team for that.
Julie Choi: Thank you, Banu. It is just a joy to introduce our next speaker. She’s a friend of mine and a former colleague, really an all-star. It’s so wonderful to have you here, Banu. Thank you.
Julie Choi: Banu Nagasundaram is a machine learning product leader at Amazon Web Services where she owns the go-to-market strategy and execution for AWS Panorama, an edge computer vision appliance and service. Prior to AWS, Banu has spent over a decade in technology, building AI and high-performance computing products for data centers and low-power processors for mobile computing. Banu holds a Master’s in EECS from the University of Florida and an MBA from UC Berkeley’s Haas School of Business. Let’s all welcome Banu.
Banu Nagasundaram: Thank you, Julie and team for having me here. I’m super excited to be here. One difference from the other speakers is this is not my area of expertise, the title of the talk. It is something I’m trying to do better at that I wanted to share with you.
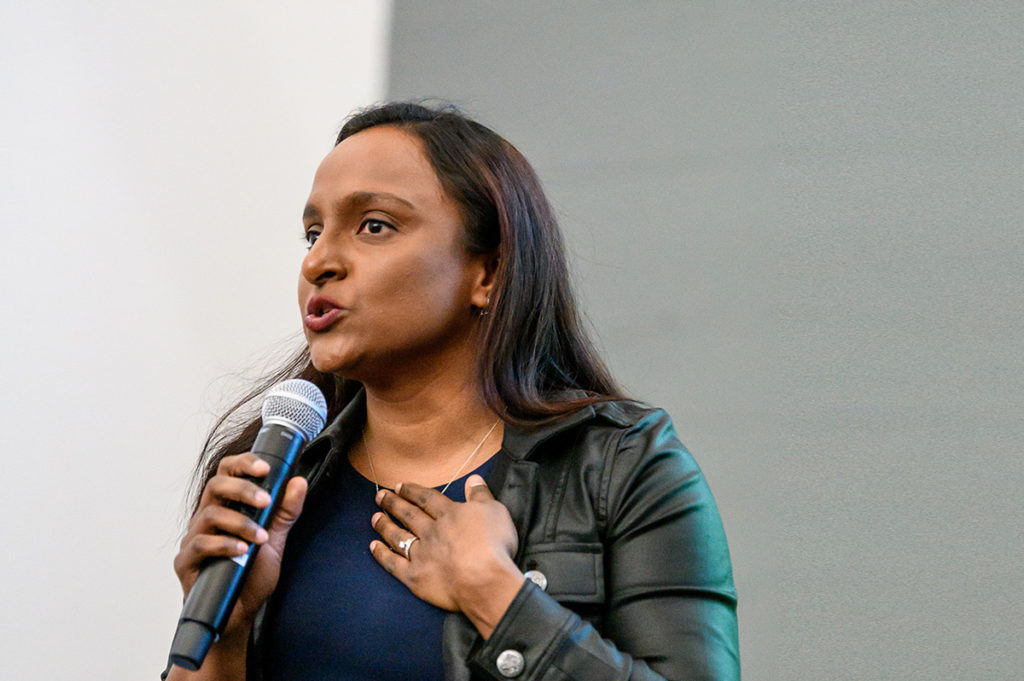
AWS Product Manager Banu Nagasundaram speaks about seeking the bigger picture as a ML product leader at MosaicML Girl Geek Dinner. (Watch on YouTube)
Banu Nagasundaram: I’m trying to seek the bigger picture at work. I’m a Product Manager and I’m trying to see why companies do what they do and learn more in that process. What I want you to take away from this is how you can also seek the big picture in your roles that you do either as engineering or product leaders.
Banu Nagasundaram: With that, I wanted to share a little bit about the companies that I work with on a daily basis. These are concerns who use machine learning and AI and work with AWS to implement the services in production. This is different from the research that we spoke about.
Banu Nagasundaram: These companies are looking at getting value out of these systems that they put in place, of course, based on the research, but taking into production. What I implore you to think about is put on a hat of a CTO or a CIO in each of these companies and think about how and why you would make the investment decisions in machine learning and AI.
Banu Nagasundaram: For example, I work with healthcare and life sciences team. I learned a lot from the drug discovery talk earlier from Tiffany here, but I do work with healthcare and life sciences team to understand how they can take the vast amounts of health data that they have to translate into patient information that they can use to serve patients better.
Banu Nagasundaram: They use multiple services to personalize, to extract value from the text data, a lot of unstructured data that they have. That’s one category of customers.
Banu Nagasundaram: The second type of customers that I work with include industrial and manufacturing. The key component that they’re trying to improve is productivity and also optimizing their manufacturing throughput.
Banu Nagasundaram: The questions that they ask and they seek to improve include automating visual inspection. How can I improve the product quality across my manufacturing sites? I have thousands of sites in the US. I scale globally. How can I implement this process not only in one site but uniformly across those thousands of sites to achieve something like predictive maintenance on the tools, improve uptime of their equipment, etc?
Banu Nagasundaram: Third set of customers we work with include financial services. They are looking at data to improve or reduce the risk in the decisions that they make. They’re trying to target customer segments better so they can understand underserved populations but lower the risk in making those products and offerings that they want to do.
Banu Nagasundaram: They also look into fraud detection and many applications around financial services. Retail, this is one I work closely with because I work in the computer vision team right now. And retail is trying to use the insights from computer vision products to see how they can reduce stockouts, which is basically when you go to the store, is the product available? Can they sell it to you? How can they manage inventory? Can they keep track of the count or the number of people entering the store?
Banu Nagasundaram: You may have heard about Amazon Go, for example, a store with just a walkout experience. A lot of retail companies are working with us to understand how they can use computer vision to build experiences like that.
Banu Nagasundaram: And it doesn’t stop at a retail store. Think about the operations officer, a centralized person who’s sitting and trying to analyze which region should I invest more on? Which region should I improve security? Should it be in North Carolina? Should it be in California? Or should it be in a whole different country? They’re trying to collect and gather insights across their stores regionally, nationally, internationally to make those decisions.
Banu Nagasundaram: Then there’s media and entertainment too, which we touched upon a little bit around recommendation systems and personalization. Here we work with customers who are looking to improve monetization, who are looking to create differentiation in the marketplace, in the very highly competitive media and content marketplace, through those recommendation systems and personalization that they have.
Banu Nagasundaram: Across all of these customers, the core task as a product manager that I work on is understanding their requirements and then translating it into product features so that they are served better. But what I learned in the process is that it’s so much more in decision-making than just understanding about features or product requirements.
Banu Nagasundaram: It’s about what enables these CTOs to make those decisions is value creation. How can they use all of these AI ML systems to realize value from the systems that they put in place? One simple way to think about what value creation is, is that it’s an aggregation of data, analytics, and IT that brings the machine learning together. But there’s a second part to it, which includes people and processes.
Banu Nagasundaram: What I mean by that is all of this analytics and machine learning and data can help them understand something, but they still have to lean on people to analyze the data that they gather, make improvements in the process in order to recognize and create value. And that’s the workflow for decision-making across all of these companies.
Banu Nagasundaram: We can look at this as two buckets, one in data analytics and IT, and the second one as people and processes. For the computer vision product that I own, I wanted to talk to you about the value chain for the first part, which is the data analytics and IT portion.
Banu Nagasundaram: This might look a complex set of boxes. It is. Once I finish the pitch here, if this excites you, I’m hiring too, growing my team. Hopefully, I do a good job in explaining this value chain.
Banu Nagasundaram: I started my career in the bottom left, by the way, in silicon processor design. Pretty much in the bottom row, trying to understand how silicon design is then aggregated into components and how distributors sell those components to OEMs who are equipment manufacturers, and how from those equipment manufacturers, the equipments reach the consumers, which is through those equipment distributors.
Banu Nagasundaram: My product is currently both an appliance and a service. I do start from the silicon side, working with partners, I can give examples like Nvidia on the silicon side, Lenovo on the OEM side. And then once you have these equipment distributors selling these devices or equipment I should say to infrastructure providers, one of the examples of infrastructure providers is cloud service providers, but it can also be on-prem equipment providers.
Banu Nagasundaram: You then go to the infrastructure providers, which is the second row from the bottom, and these infrastructure providers then build the tools and frameworks either themselves or through the partner ecosystem. Those tools and frameworks essentially put in place to make efficient use of that underlying infrastructure. It’s a motivation for these infrastructure providers to offer the best tool and frameworks so that you can gain that value out of that underlying infrastructure.
Banu Nagasundaram: Then comes the ML services. You have the overall MLOps flow is what would fit in this bucket. Once you have the tools and the frameworks, how can all of these pieces get grouped together to build a robust system that can scale in production? This goes from data annotation, labeling, training to predictions, to model monitoring. “How can you maintain this when it is in production” is a key question for these decision-makers.
Banu Nagasundaram: Then comes the AI services that are built on top of these ML services. AI services can either be services offered by CSPs or it can be services offered by startups or companies who are trying to build the microservices or services on a specific use case. This is where the customer use case comes into play, where you have that specific use case. In case of computer vision, you can think of services like Rekognition or Lookout for Vision. Those are two examples that AWS offers for computer vision services.
Banu Nagasundaram: Then comes the final layer. This is the layer that the customers that I refer to, the CTOs, work closely with. The independent software vendors or ISVs are companies that build these software solutions, aggregating everything that I spoke to you about, but building the software components of it. But the software component by itself is not going to function in a customer’s premise. For the customer to realize value, the solution, the software solution for their use case has to integrate with their existing system. That’s where system integrators come into play.
Banu Nagasundaram: For example, Deloitte. You can think of Deloitte, Accenture, et cetera are system integrators who bring this whole puzzle together for customers, build that reference architecture for that solution. And they’re like, okay, so now we have this architecture in place. Then they bring in the value-added resellers, who are companies like Convergent or Stanley, for example, who take this entire system that’s put in place and roll it into individual sites.
Banu Nagasundaram: This is where it reaches the scale of thousands of sites. Once a solution is put together, proven in a pilot or a production pilot or a proof of concept, when you go to production, across globally, across cities, across countries, the value-added resellers roll the system into place in the customer’s site. But it doesn’t end there. There need to be managed service providers who can offer service contracts to maintain the system in place once it is on a customer’s premise.
Banu Nagasundaram: All of these building blocks in the value chain is what makes ML, machine learning, in production, intangible for a customer to realize value. It is a big journey. And this is the team I’m building who will work with individual partners across this value chain. And if this is something that excites you, we can talk after. In that value chain, we started with value creation. What is it that companies are looking for? We saw the value chain, but the value realization, let’s say the companies went through this process, put this whole system in place.
Banu Nagasundaram: What is it that actually helps them realize that value? When we think about data analytics in IT, many of the ML practitioners that I talk to, talk to me about the output, the visualization, the dashboards, the histograms for decision making, but it doesn’t stop there. That is not sufficient for these companies. There has to be people in those companies who take this output and actually work to achieve an outcome. The outcome is increase in productivity, increasing in throughput. It’s like, if I can know my demand better as a retail store, or if I can forecast the demand better, I can do so much better in my business.
Banu Nagasundaram: That is an outcome that I’m looking for from this data output that I’m getting from the machine learning models. But is that outcome sufficient? No, the outcome is like one place, one time, you are able to visualize that outcome, but you have to scale that outcome globally in order to achieve the impact. The impact that businesses look for is that you have to either increase revenue, reduce costs, reduce their risk, improve their sustainability, or create a competitive advantage. This is what the ML journey in production looks like. While I walked you through incrementally from the beginning on what it would take customers to get there, customers don’t work through individual steps, reach there and see what’s the next step.
Banu Nagasundaram: They actually have to make assumptions along the way and then understand what the impact might be and this is an AWS term that I’m going to throw and work backwards, which is understand what is it that you want and then build out what is it that you can, what you need to do towards achieving that end goal. That’s the big picture that I want to leave you with. In this whole ecosystem of having machine learning in production, can you think big on behalf of the customer, can you seek the big picture that the customer is looking for? If you’re working on a feature, what is the end state of that feature? What is the end state of that business? Who is actually your end customer? Your end customer may not be the team that you’re immediately working with and who is the decision maker for that overall flow?
Banu Nagasundaram: One of the simple frameworks, this might sound silly. It’s super simple, is to ask five whys, which is in a customer discovery or any feature that you’re building. Just ask, why is that outcome important? Why is that output important? How is it going to help? Why is it going to be something that helps the customer? Why is it needed tomorrow? All of these questions is just going to help drive a little bit more clarity into the bigger picture and motivations for the customer on how they make investment decisions and choices in your particular products and features that you’re building. And that’s it. I want to leave you with one fair warning. If you try that five why’s with your partner, that’s on your own.
Julie Choi: All right. Thank you. Banu, that was great. Thank you so much. It is my honor to introduce Lamya Alaoui, a dear friend of mine and I’m so thankful to you, Lamya, for agreeing to give the closing talk of the night. Lamya is currently Chief People Officer at Hala Systems, and she has been committed throughout her career to supporting organizations as they shift behaviors to align their talent strategies with their business objectives. Her corporate background includes over 15 years of experience in talent acquisition and management, where she has had the opportunity to build teams for companies such as Bertelsmann, Orange, Groupon, Google and Microsoft. Her work experiences span North America, Europe, Asia, Middle East, and North Africa. Let us welcome Lamya Alaoui.
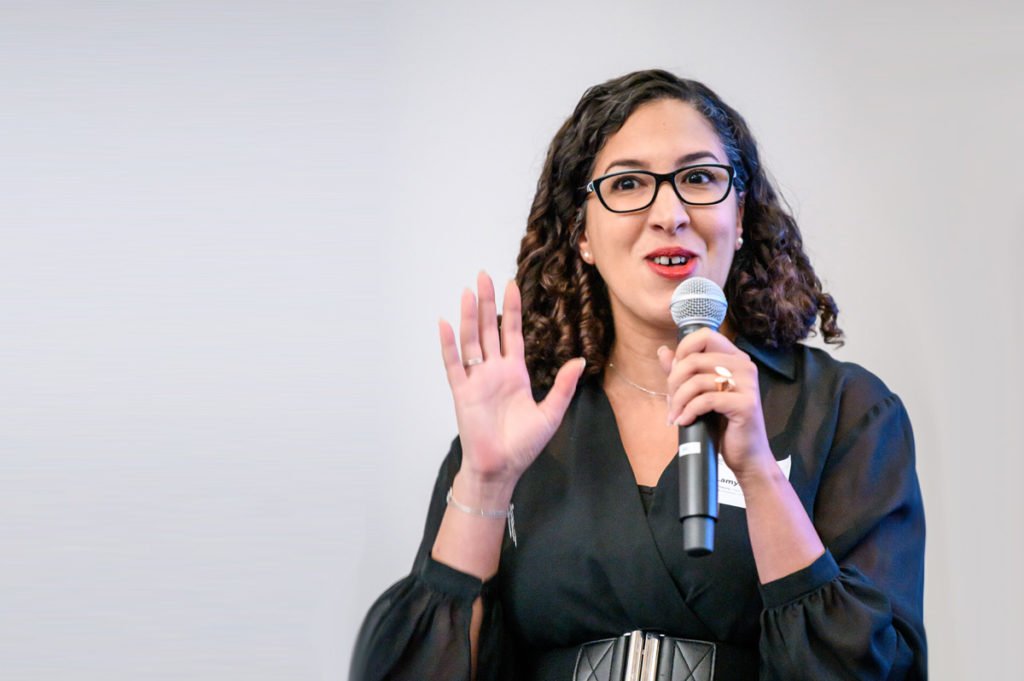
Hala Systems Director of People Ops Lamya Alaoui talks about 10 lessons learned from building high performance diverse teams at MosaicML Girl Geek Dinner. (Watch on YouTube)
Lamya Alaoui: Thank you so much, Julie. Hi everyone. Thank you. I don’t see Sarah here, but I want to thank her personally because she has been so patient and Angie as well for inviting me here. How is everyone feeling today? This is the non-tech talk. This is the people talk. A quick background before we get into it. At Hala System we develop early warning systems in war zones. Not the type of things that you broadcast usually when you are in this type of settings, but we’re hiring as well, especially for our AI team. And Julie announced my promotion that no one else knows about, even in the company so, thank you. That stays here, please, in this room, until next all hands, on Wednesday. With that being said, a little bit of background about me. I’m Moroccan.
Lamya Alaoui: I moved to the US about 10 years ago. I will ask a lot of grace because my brain is wired in French. Sometimes there will be French words that will come out from my mouth so please be graceful about it if you can. With that being said, this is one of my favorite things because as a Moroccan who’s half Muslim, half Jewish, went to Catholic school, I thought I got it covered, but I was the lady the first time I landed in Germany because I was not aware that you’re not supposed to kiss people to greet them. But this is one of my favorite pictures to show and talks or even in workshops, because I’m pretty sure it happened to all of us one way or another. We show up and we think that we got it right. It’s what we’re familiar with and actually it’s not what the other person is expected.
Lamya Alaoui: Throughout my career, I have built teams in very, very different countries, different cultures. At Hala we have over 17 nationalities. Altogether we speak 25 languages. It makes it interesting for the meetings, believe me, where you have side conversational Slack in a whole different language, but you still need to deliver officially in English. The translation is super weird sometimes, but those are anecdotes for another time. That leads me to one of the lessons that I learned very early on. It seems obvious that when you’re building teams and you want them to perform, one might assume you want to know your mission and your values, but it doesn’t happen as often as one might think. The mission is basically your GPS. You want people to rally behind a common goal.
Lamya Alaoui: You want them to believe in that and it’s also what’s in it for them when they’re working for a mission. That means that it needs to be aligned with their own values or their belief system. SVlues start very early on. We all have seen or worked for companies where the values are stated. How many of you when you joined the company saw values listed on the website or have been talked to? How many of you were explained to what are the associated behaviors and expectations when it comes to those values? Oh, we have less people. This is one of the first lessons that I always recommend to people to kind of follow. I learned the hard way, by the way, is that know your mission, state your values. Values should come from the leadership of course, but also account for it in the hiring process, which we will get a little bit into it, but be very clear about what are your expectations are when it comes to values.
Lamya Alaoui: If I’m thinking about transparency, for me, it means having information that I need to do my job, but some other people, they think that they need transparency. They need to have access to everything. We all have someone like that in our companies. Don’t we? Setting those clear expectations and associated behaviors are very important. And mission is like literally your North Star. This is why it’s really important. The second one is seek first to understand. This is from Stephen Covey. There is a second part to it which is, then to be understood. When you are building diverse teams, we all come from different backgrounds, we have different understandings, often we speak different languages. We can also come from different cultures, high context or low context and if we don’t have mutual understanding we cannot succeed because then everyone is convinced that they’re right, that their approach is again the one that everyone needs to follow, but that kind of hinders teamwork, which is actually essential to high performing teams.
Lamya Alaoui: Listen. We all think that we listen pretty well, but we actually don’t because as human beings we have been trained, for the last few centuries, to listen to answer. We’re almost never listening for the sake of just listening and there is amazing research about that, that I will be more than glad to share. In working in very diverse teams or diverse teams in general, listen, help, create understanding, respect, which is a very important when it comes to having high performance teams as well. How many of you would say that they listen really, really, really well. Okay. We have four people in the whole room. That gives you something to think about. That’s another, and I’m a good listener, but still a lot of work to do with that.
Lamya Alaoui: Acknowledge that you will face cultural differences. When you join a new team, we all want to get along. Again, its human nature. We want to belong somewhere. We’re super excited. It’s a new job. We just went through the hiring process. We landed the job and then you show up in that meeting or you’re meeting your team and sometimes you see people that you have nothing in common with, and you still try to ignore that, which is again, human nature. Beautiful thing. Just as a manager, as a team member, be prepared that yes, we will have some challenges. We’re not seeing things the same way. One of the best examples that I always give here is like, I’m from what would qualify as a high context culture, which is there is no explicit direct messaging.
Lamya Alaoui: Basically I can be in my office and say, hey, I have a lot of work and I’m hungry. My expectation is that my coworker will just get it and go get me some food. Now, imagine if I’m having someone from a low context culture. In their head, they’re like, I mean, you’re going to go get your food when you’re done. That creates a fracture that it’s not even intentional because there will be resentment from my end. Why didn’t he get it? Having those conversations upfront is quite helpful because then people know again, what to expect. Assess the degree of interactions. When teams are being built, most of the time what happens is no one is thinking about how often the team members will have to work together, at what intensity.
Lamya Alaoui: Before starting to build the teams or adding members to your teams, just make sure that, hey, how often do they have to have, I don’t know, sprints. If it’s once a month, eh, you might want to have people that are pretty much aligned having somehow the same thought process and we’ll get to that in a little bit. If they’re not interacting a lot, you have a lot more room to assemble your team. Always, there are three degrees of interactions. We have low interdependency, which is people are doing their things on their own and sometimes it’s like going in a [inaudible]. Medium, which is a hybrid and then high interdependency, which the output from someone becomes the input for someone else.
Lamya Alaoui: Therefore, things need to be structured in a very certain way in making sure that people get along and understand, again, what’s expected from them. Communicate, communicate, and communicate and when you think you are done, communicate some more. One of the things about communication is that we all think that it happened. Again, the question, oh, disclosure, I ask a lot of questions. I mean, I used to be a recruiter. It comes with the territory basically. How many of you thought in a meeting that you were crystal clear and then two days later you discover that you were not.
Lamya Alaoui: When it comes to communication in teams, again, thank you, Slack, everyone in meetings, or usually where everyone is doing a lot of things at the same time. You’re talking, you think that you got your point across. Always ask a question at the end. What do you think? What are your takeaways? Just to make sure that people are on the same page and teams, high performing one’s, one of the best practices is having action items assigned, even if it’s an informal meeting or if a conversation happened on Slack, on, I don’t know, any other platforms. Even sometimes now, oh, we have also Asana now people add us to Asana and they think that, hey, we’re good.
Lamya Alaoui: No, make sure that the person actually went in, is understanding what you’re waiting for or expecting. Diversity and leadership. Clearly icons still have a long way to go in terms of diversity, but again, high performance teams when it comes to diversity need to see that diversity reflected in the leadership and in the management. Research shows that usually entry level management, there are sometimes good numbers, but as soon as we go to director, VP and above underrepresented groups tend to become less visible or they’re non-existent and C-suites and VP, EVP, SVP type of roles.
Lamya Alaoui: It’s also very important that the leadership reflects the diversity that the company is striving for. Again, an obvious one, hire the right people. This one is very dear to my heart because as I said, I’m a recruiter. Again, we’re only humans. We tend to hire people who are like us, who think like us, who share our values. Anyone wants to venture what happens when you hire people who are like you and your team and you’re building a team of clones, basically from a cognitive perspective. Anyone? Yes.
Shika: You wouldn’t get to learn something different from what you already know.
Lamya Alaoui: Yes. Anyone? Thank you so much. What’s your name?
Shika: Shika.
Lamya Alaoui: Shika. Thank you, Shika for volunteering. When you build a team of clones and this is a tech talk after all, how do you think that you can innovate? How do you think you can perform if everyone is thinking the same way and not challenging the ideas that are being discussed? Chances are very, very, very slim.
Lamya Alaoui: When you’re hiring the right people for your teams you have to look at three things. The first one is the values, because that’s, again, what will be the foundation of the team that you’re building. The second one is what’s missing from your team. An ideal team has five type of people. You have a theorist, you have a strategist, you have someone who is an analyst, you have a manager and you have an implementer. When we hire our teams usually we hire in desperation because you know, you got to deliver on that project.
Lamya Alaoui: No one is thinking about the composition of the team in itself. In tech, my experience showed we have a lot of theorists and strategists and a lot of analysts, sometimes zero implementer and zero managers. Things don’t get done somehow. Always look at what’s missing and finally, the third part is people who are willing to learn, who have a curious mindset and are eager to grow, because this is how actually innovation happens. People who are not afraid basically of questioning the status quo.
Lamya Alaoui: The other lesson is constantly scan for misunderstandings and ways to clarify. Again, here I will go to the low context, high context type of thing so, let me give you an example. I think it might be easier to make my point that way and I will ask a question at the end. In high context cultures or in some cultures when you ask questions, no, is not an acceptable answer. You have to say yes and some others you have to say no three times before saying yes.
Lamya Alaoui: Imagine when you have people from different cultures in the same room and someone is asking a close question that requires a yes or a no. What are the chances, based on what I said, that you will get an accurate answer to your question? 50%, 60%, 20%, a hundred percent. For this always ask open ended questions to give people space to answer without having to break their own boundaries and to make sure that you are getting clarity in the answers. How many of you were in meetings where you felt that you were misunderstood when you were speaking? What did you do? Anyone wants to volunteer again? When you felt that you were misunderstood? Yes.
Angela: Have you summarized in a written notification? I gave action items to the people I wanted clarification from that helped with the misunderstandings.
Lamya Alaoui: Thank you, Angela. One of the things that is really helpful and again, best practice for high performing teams, always summarize and put things in writing and this setting English is the working language. We do have a lot of people, I know in my team, I have a lot of non-native speakers. I’m not a native speaker so to make sure that the message gets across and that it’s clear it’s always in writing, always, which we tend not to do because everyone is working long hours or you have back to back meetings. Then at the end of the day you just want to finally get to do your work and you never summarize in writing what happened in those meetings.
Lamya Alaoui: Finally, the structure. Build a structure that is around diverse teams. When we’re usually building teams we have, we’re looking at what now is referred to as visible diversity, which can be ethnicity, religion, gender and many other things, but we’re never looking at the cognitive one that also has a heavy part, especially in tech. Those are recommendation that I’ve always lived by every time that I join a new company. We do a reintroduction and relaunch of the core values. We redefine them. We create, and this is like a company work, it’s not just someone in their corner doing it, but it’s a collaborative work setting what are the core values, what are the expected behaviors and the ones associated with each one of the values, and then it’s communicated throughout the whole company. When it comes to performance review it needs to be designed with underrepresented communities in mind.
Lamya Alaoui: Performance reviews, if you look at the history of how it was designed, it was designed for a very specific community or population. It doesn’t fit other people. Just leave it at that. Work on creating a performance review management system that is more inclusive. Yeah. Targeted networking. Again, this ties to the diversity and leadership. One of the things, again, hiring in despair. When those roles are open, people tend to hire fast from networks that they know because they don’t have networks that are already established. This is something that is quite helpful. I don’t think there are any people in the HR realm tonight, but all of you can be ambassadors for something like this to be established in your companies. And again, clear expectations around the engagement and the roles. What are the pathways to promotions? Again, if you look at internal promotions or internal applications, woman tend for, this is just an example, women tend not to apply to internal openings when it comes to higher positions in the company.
Lamya Alaoui: Sometimes just because they don’t check all the boxes so the work starts before that, where we actually need to make sure that the job descriptions or the job openings are reflecting and are being thoughtful about underrepresented groups. Finally, this is one of my favorite things, Kaizen. There is always room for improvement. Performance and diversity are a very long journey. Each company is at a different stage or different phase. Just ask questions, be kind because not a lot of people think about it when it comes to those things and be as curious as possible because at the end of the day the only way to know other people is to ask questions. Thank you so much everyone.
Julie Choi: I want to thank all of our speakers tonight and for all of you, for being such an incredible audience. We have people who have been watching these talks outside in the overflow and I’m told that, I think it might be better if we just all go out and just mingle. Get some fresh air after all this time is for us to connect and network. Why don’t we continue the conversation outside? Thank you everybody.
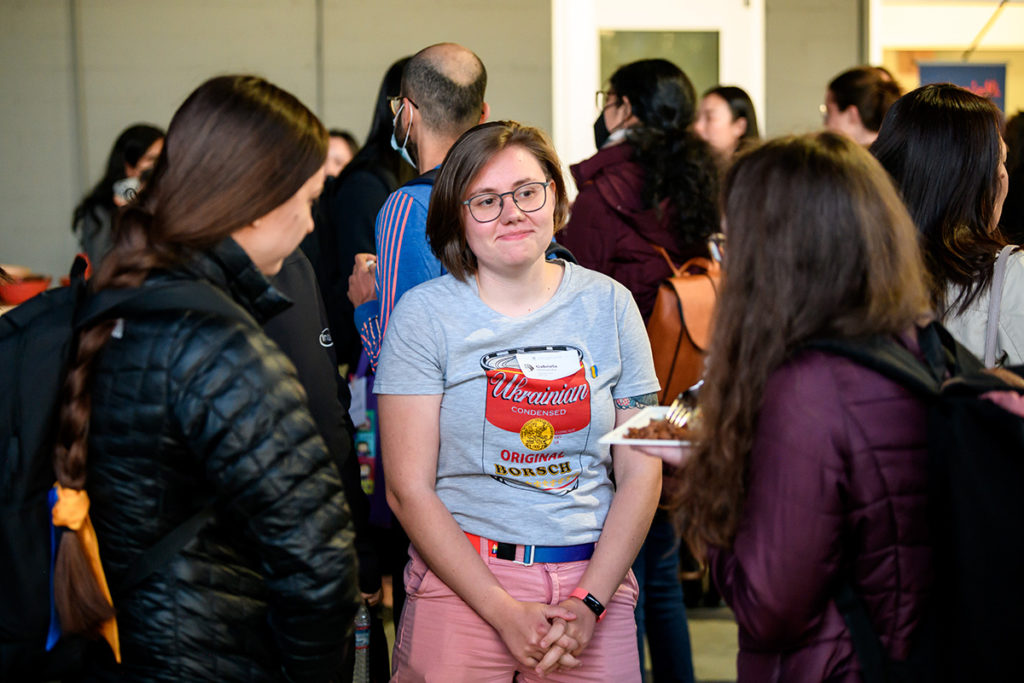
This site reliability engineer discusses Ukranian borscht or machine learning, or both, at MosaicML Girl Geek Dinner.
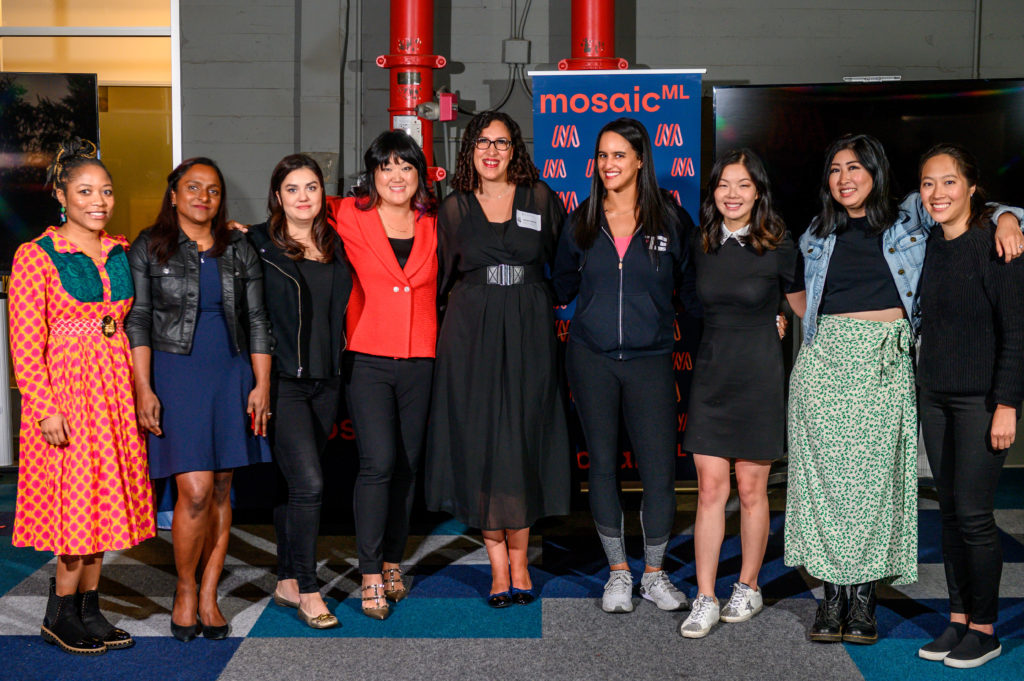
MosaicML Girl Geek Dinner speakers after the event: Tiffany Williams, Banu Nagasundaram, Laura Florescu, Julie Choi, Lamya Alaoui, Shelby Heinecke, Angela Jiang, Angie Chang, and Amy Zhang.
Like what you see here? Our mission-aligned Girl Geek X partners are hiring!
- See open jobs at MosaicML and check out open jobs at our trusted partner companies.
- Find more MosaicML Girl Geek Dinner photos from the event – please tag yourselves!
- Does your company want to sponsor a Girl Geek Dinner? Talk to us!