VIDEO
At ELEVATE, AI trailblazers Julie Shin Choi (Cerebras Systems SVP & Chief Marketing Officer), Savita Kini (Cisco Director of Product, Speech & Video AI), Gabriela de Queiroz (Microsoft Director of AI), and Lamya Alaoui (Hala Systems Chief People Officer) discuss everything from their career journeys, to staying up-to-date on the latest in AI research, AI ethics & more.
In this ELEVATE session, AI trailblazers Julie Shin Choi (Cerebras Systems SVP & Chief Marketing Officer), Savita Kini (Cisco Director of Product, Speech & Video AI), Gabriela de Queiroz (Microsoft Director of AI), and Lamya Alaoui (Hala Systems Chief People Officer) discuss everything from their careers to staying up-to-date on the latest in AI research, AI ethics & more.
Like what you see here? Our mission-aligned Girl Geek X partners are hiring!
- Check out open jobs at our trusted partner companies!
- Watch more ELEVATE 2024 videos from the event, or just the “Best Of 2024” Videos!
- Does your company want to sponsor a Girl Geek Dinner or Virtual Conference? Talk to us!
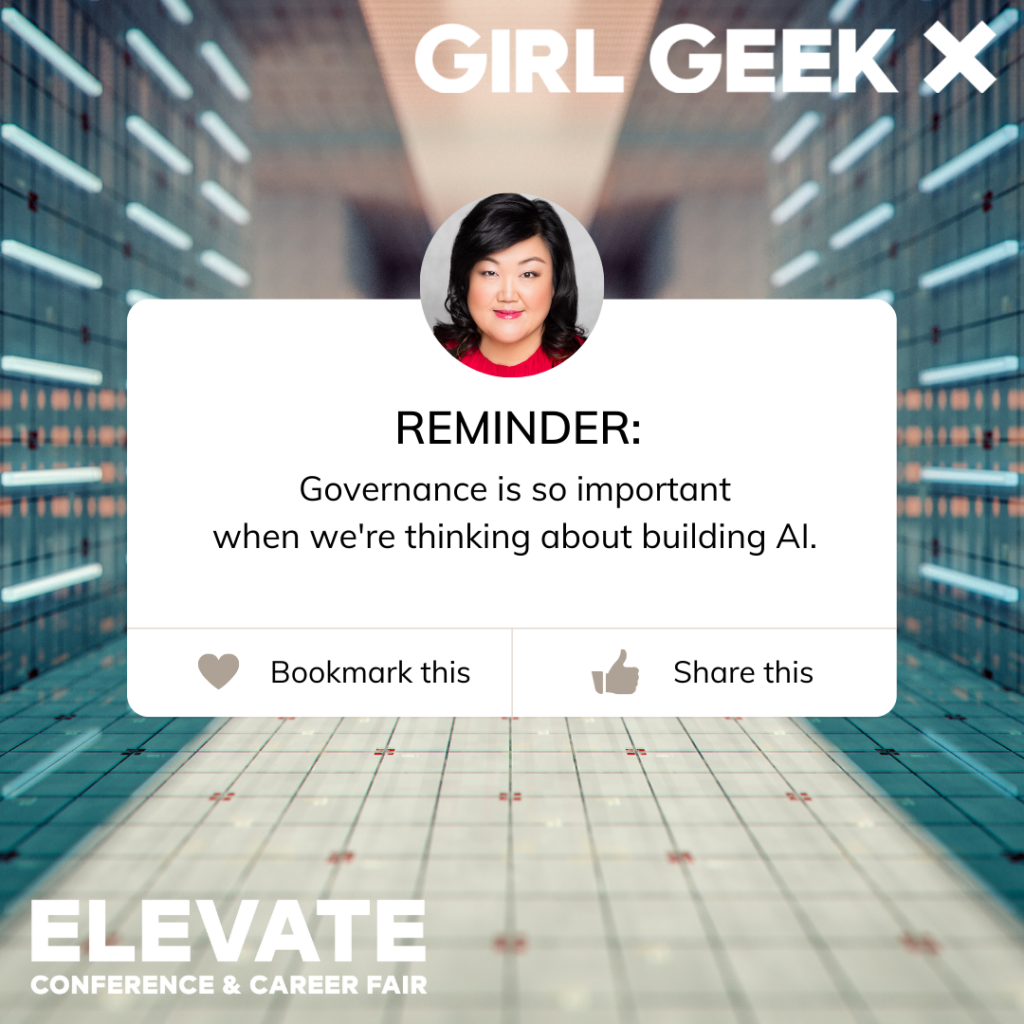
Transcript of ELEVATE Session:
Lamya Alaoui:
Thank you so much, Sukrutha. We’re going to do it family style where I would hand the mic to each one of our panelists to get acquainted with our audience, and from there we will have a discussion about AI and their experiences doing this for as long as they have done it. I’ll start with Gabriela, would you mind just quick intro, less than 30 seconds and then you can hand it to somebody else?
Gabriela de Queiroz:
Absolutely. Pleasure to be here. My name is Gabriela de Queiroz. I’m a Director of AI at Microsoft. More specifically, I work with startups, doing a lot of engagement with them, talking to them about Microsoft product and anything that it’s around AI. And I’m so excited to be here today. We’ll be talking more about AI and the fun stuff and I’ll pass to Julie.
Julie Shin Choi:
Hi everyone. I’m Julie Choi, and I am the CMO at Cerebras Systems. We are the makers of the largest AI chip in the world. And I’m excited to be here today. Many thanks to Girl Geek X and Elevate for bringing us together.
Savita Kini:
Hi, I’m Savita. I’m Director for Speech and Video Technologies here at Cisco. Many thanks to Girl Geek and ELEVATE for inviting us to this panel. Very excited to be here. Look forward to sharing my experience, how I got into this gigs.
Lamya Alaoui:
Okay, thank you everyone. My first question is for the three of you, we’re going to go through a journey and explore different aspects, but the first question is, can you share a pivotal moment in your career that convinced you of the potential of AI and also how do you stay updated? Savita, I will start with you.
Savita Kini:
Yeah, thank you. There is not one pivotal moment. I’ve been in the enterprise tech space for now over 20 years. That started my journey in networking, moved into solutions healthcare. I also did field roles in Singapore, so I had a global experience. When I moved back here to the Bay Area, I saw all these different forums with cloud and big data, and slowly I started seeing AI come up in some of these sessions.
I had done my undergrad in double E, where I actually had done a research paper in neural, networks, that’s 20 plus years ago. It was so tiny and so simple. And now with all the big data available I could that the trend was something to this new technology because data was available.
I wouldn’t say it was one pivotal moment, it was more like I saw that, “Oh, this is the bigger trend. It’s not the big data, it’s not the cloud. It’s really what we do once you have the data.” To me it was more about looking at what application AI will bring, let’s say, in the IoT space or in collaboration.
I would say it was more a journey of discovery to see what’s the new inflection point. It was probably around 2018, 2019 when I felt that that’s where the new trend is going. In terms of how do I get myself updated, I use podcasts, LinkedIn, a lot of reading, newsletters.
There’s so much information available there, I think there’s a overload of information. It’s more about picking who you listen to, who feels authentic to you and how it applies to your work and what you are doing because it can be too much of an information overload. Maybe Julie, you want to take up?
Julie Shin Choi:
Oh, sure, yeah. The pivotal moment in my career that convinced me of the potential of AI was back in 2015. I was hired to do product marketing at Hewlett Packard Enterprise, and my specialty was developer marketing, so developer platforms, up to that point, it was APIs, web services for mobile app development, social apps, Firefox, that kind of thing.
But in 2015 there was a clear shift, it was a movement towards machine learning. As many of you may remember, and maybe not, but 2015 was the first year that Google repositioned itself from being a search company to a machine learning company, and 2015 was probably one of the first years where Nvidia started calling itself a deep learning company.
As I was just working on marketing this platform at HPE, lo and behold, what it was, was it was a machine learning platform as a service with over 70 APIs for functions like speech-to-text, object character recognition, sentiment analysis, emotion detection, all of these little things, these machine learning functions.
What I was really impacted by was, how excited developers were, and I remember launching this thing called Haven OnDemand, which was quickly killed about nine months later, but we got to launch it, and even Jim Cramer, Mad Money, CNN, he picked it up, he’s like, “Wow, HPE launched this machine learning thing.” This was back in 2015, and I knew there was just something very special happening.
As far as how I stay up to date, luckily since I’ve been working in the space for about eight years or nine years now, a lot of my best friends work in the domain, so I just call and we chat and catch up.
Gabriela de Queiroz:
Awesome. I love the whole history, Julie, how things are evolving. For me it was similar to both a few, there was not a single pivotal moment that convinced me. It was a gradual move throughout my career journey, so began with statistics and then moved toward ML, machine learning and AI.
This transition was more like a natural progression where I was one step building on the last, moving from the more foundation principle of statistics to a more dynamic and adaptable approach of ML, and then now finally to this more broader scope of AI.
What convinced me about AI was not just the technological advancement, but the realization that these tools can be used to solve real problems, problems that before we could not solve. Examples would be improving healthcare diagnostics to enabling more efficient energy use or creating more personalized learning experience, for example.
It’s been this cumulative process where informed by my experience and the gradual impact of AI technologies in various domains. For me, it’s a realization that AI is not just a tool, but this transformative force capable of driving significant advancements and solutions for the future. I feel like it’s very painful to be up-to-date in everything that is happening because there is so much. I don’t remember any other time in my professional life where I’m playing this catch up game every day, and because I work with startups and I’m working in AI, I need to know everything that is happening.
Also, it’s a struggle, how do you balance your full-time job with everything else that you need to know and you are in the meetings all day? Anyway. It’s very chaotic. It’s very hard. What I try to do, I try to leverage a combination of online sources, resources, and in-person events. I follow some people on LinkedIn, Twitter.
I subscribe to some newsletters on Substack where we have people that compile weekly summaries. I go there and then I have a weekly summary, and then for the topics that I’m more interested in, I go and click. The other thing is, I would say the advantage of being in San Francisco is that we have a lot of events, so I take advantage of attending those local events and learning from people as well, which is an amazing resource.
Lamya Alaoui:
Thank you so much. I want to go back because there’s clearly a pattern here when it comes to the amount of information available out there. Savita said the problem is like, “Who do I actually trust to listen to?” There’s also a lot of research.
Savita, my question is for you, in your opinion, what is the next frontier in terms of AI research? Because you also have a background in research. What are the challenges that in our industry we need to overcome to get there? Because there is an insane amount of resources out there.
Savita Kini:
Absolutely. I think most of everybody has been overwhelmed with ChatGPT and the models on the LLM site coming out every day. I’m a multilingual person and I can see Gabriela is multilingual, most of us in this panel are multilingual, english is not our mother tongue. And I feel that this planet has billions of people and so many different languages and they’re not part of this new AI ecosystem.
The next frontier, I’m following people in India who are building up multilingual datasets, speech, as well as transcribed for Indian languages. One of their datasets and their models, they just announced a couple of days ago.
I feel that the next frontier of research, LLM is just the beginning, but really speaking, it’s all about how do we make this technology inclusive and accessible. My dad and mom should be able to communicate in their own language with voice assistance. I don’t think we are there.
Just even a year ago, neither Siri nor Google understood me, even now, my daughter laughs when Siri is not reacting to what I said and she didn’t understand. I think the next frontier is really about multilingual speech-to-speech translation without the transcription, because some of the transcription is also not accurate, to be honest.
When I see some of the English transcription because of our accents, it’s not accurate. I think to me, when we build these massive, much more inclusive speech-to-text, text-to-speech and LLM ecosystems and multilingual languages is when we have really started including everybody. And to me, that’s the real hard next frontier. It’s hard. I don’t think it’s happening.
Gabriela de Queiroz:
Yeah. I’m so glad that you touched on this point because I feel your pain every day on that, either to talk to AI assistant or to reply an email. I’ve been in the US for over a decade and almost every email before I send, I go and check and I would say ChatGPT has been done a tremendous amount of helping me on checking on those emails. Even some features inside Outlook, for example, they have been amazing, but I still think that we are a little bit far from where we want to be in terms of language and the multi-language piece.
Lamya Alaoui:
Okay. I would love for us to explore a little bit more on the societal impact. And my next question is for you Gabriela, because there is definitely, I’m also multilingual person and working on project where there is a necessity to use different languages that are not even on the map for some of them.
Gabriela, my question for you is, in your opinion, what measures can be implemented to ensure that the technology is accessible and beneficial to all segments of society? You touched upon real life applications, but I would love to hear from you regarding what measures can we implement?
Gabriela de Queiroz:
Yeah. That’s a great point. That’s an ongoing problem, especially around technology and not even just AI, I think technology, it is a big problem, how do we make sure that people are aware, they know that those things exist?
Especially around AI, one of the things that struck me in the beginning of the whole AI discussion around discrimination bias, that the majority of those discussions were happening here, especially in the US and Silicon Valley and where the majority of the people that were going to be affected the most were not the people here, are probably the people in the underserved and underrepresented communities.
How do we make sure that they are aware? We need to have a lot of efforts, and one of the efforts that I think is very successful from my experience is creating communities, because that’s how you can reach communities that, they don’t have maybe internet connection, they don’t have access to the resource that we have.
Through communities, through education, making sure that we have open education and open resources, so everybody… And translated to their native language, which is very important. That’s the only way that we can make this more accessible to people.
Lamya Alaoui:
Thank you. My last question on the societal impact is for Julie, and I think everyone in this room is keeping in mind because we work in the AI field about ethics, because we cannot speak about AI without speaking about ethics of it.
Julie, how do you think we should address some of the ethical implication of AI, because there is the inclusivity part, but there is also sometimes the use or dual use of AI? So what are your thoughts on that aspect?
Julie Shin Choi:
Yeah. Governance is just so important when we’re thinking about building AI and really filling the table of people that are involved in everything from data curation, so from the very beginning, from data, preparation, which data? What’s the data looking like? Just from that point all the way to, how are we training the model? How are we interpreting this data and training that model? Like that GPT-3.5, 4 or 5, et cetera, the data to training.
We need governance, we need to make sure that there’s checkpoints along the way. Don’t just wait until you’ve spent a billion dollars to train a model and then find out, “Oops, it’s racist.” You know what I’m saying? We got to do better. We can’t just be rushing to the end, rushing to the convergence.
I do understand, businesses these days are in an arms race to release the next state-of-the-art model, but because we rush and we don’t really take time to fill the table of participants thoughtfully, sometimes we get these models that are just not fair. It starts with setting up your team really thoughtfully, making sure it’s representative of different viewpoints and different backgrounds, different genders, everything.
Then, working together with checkpoints all throughout the way from data to training, to deployment to evaluation, and then just being really rigorous about it. Don’t just rush to tell me it’s state-of-the-art. No way. You do that, then these models are not going to be as scalable for safe global use.
Lamya Alaoui:
Thank you, Julie. I want to be mindful of the time. I want to go back to something that Gabriela said earlier, because you talked about the applications in healthcare. And we’re thinking about the future and how fast it’s evolving. Can you share a little bit of how can current AI technologies support in solving global challenges, maybe climate change, healthcare crisis and so on? Because you’re exposed to startups and I think that talking about healthcare is quite important when it comes to AI.
Gabriela de Queiroz:
Yeah. No, that’s a great point. One of the things that I’ve seen in startups as well, they’re trying to solve specific niches. In terms of healthcare is, let’s just start with the doctor or even making an appointment. Let’s think about that as a patient, how do you go about finding an appointment?
Here, in the US for example, it takes forever for you to get to appointment, for you to get a diagnostic. It takes months because first, you go to your general practitioner and then they go and say, “Oh, maybe you should see this specialized person or this other specialty.” By the end, you get something, it’s like three months, four months, six months, seven months. How can we make this in a shorter waiting period? I see that AI can help a lot with that. There is some startups doing interesting stuff.
The other piece is on the doctor’s side, the doctor, when they are attending you, they need to take notes. They are not paying attention to you fully because they have to be taking notes at the same time because they are obligated to do so.
There is a whole also burnout of doctors because they cannot do both works, talking to the patient, adding everything to the computer, making sure that they complete everything in a correct way. There is also an AI way of making sure… I can go more into details, but I think I’m just saying, what are the things that we are trying to solve that’s a pain point nowadays? So that’s another example that I see some startups trying to find a way to make them more efficient for both sides, patient and doctors.
Lamya Alaoui:
Thank you so much, Gabriela. My next question is for Savita. Again, I cannot bypass your research background, where do you see the field in the next five years? And what role will it play in our daily lives?
Savita Kini:
I already touched upon the multilingual data part of it, the speech. I’m in speech and video, so I live and breathe that every day. Even my voice is difficult for some of our models just because I speak fast and women’s voices are different from male voices in terms of the frequency spectrum. It’s much more higher frequencies in our voice. And then you need to have diverse data sets. I think over the last year, Google and Facebook have realized that they didn’t have enough difference in their datasets in terms of diversity of skin tones, ethnicities, gender, age, so there is a lot of research now coming out, for example, with the monk skin tone scale instead of six skin tones. Imagine trying to put a global population of humans in just six skin tones, can you do that?
There is so much perception and subjectivity in how you put people in different boxes, especially when there is so much difference in camera technology and the display technology and so on. I think now last year there was new research that came out with 10 scale skin tones from one of the researchers at Harvard. I would say that the fallout from Gemini that happened last week with Google, all of these points to me one thing, which is, how do we build models that are A, really authentically diverse, not because somebody is saying it, but actually having governance systems and checks that can actually verify?
I think explainable AI is going to be a much bigger deal than it is today. I think it’s almost like the early internet. A lot of people, at least in the Wall Street, they’re comparing Nvidia with Cisco. Cisco had this internet boom and then bust, and is Nvidia going to see the same thing?
I don’t think that will be the issue, for example, I’m not still seeing LLM use cases as much in my daily life other than say, meeting summarization. That’s probably the only thing I’m using. Voice assistance for multilingual speech video technology that is really inclusive, how we transform some of the movie industry, just the online media and entertainment, so independent producers can participate using AI. I see some of those areas emerging in the next four to five years, I would say. I think Gabriela sees more of it in the startup ecosystem, so I’m still so focused internally, I’m just watching two areas.
Lamya Alaoui:
Okay. Thank you so much. We have about five minutes left, and I do have two last questions, one for Julie and one for all of you. Julie, because you were talking about the importance of having a team, a team that is designed and put together in a thoughtful way, keeping in mind a lot of aspects when it comes to cognitive diversity, background diversity and all of that, how important do you think is the interdisciplinary collaboration? I’m thinking about it from an industry perspective in advance in AI, and can you maybe share a story of what a successful collaboration in this space looks like?
Julie Shin Choi:
Yeah. We are really interested in domain specific AI at Cerebras, and this requires a collaboration between the ML experts who work at my company, but really collaborating in an interdisciplinary way with domain experts across certain industries. We announced, Mayo Clinic, for example, is one partnership where the ML hardware and software and algorithm’s teams at Cerebras partner very closely with the doctors at Mayo Clinic, which is the number one hospital in the US, and we’re working together in that way to build these amazing generative AI models that are attacking some of the most intense diseases like rheumatoid arthritis, for example.
Lamya Alaoui:
Thank you so much. My last question, because today it’s about elevating others and lifting up careers, what advice can you give to our audience in terms of either they’re just starting on the AI side of things or in the tech world, or to advance their careers based on your own experiences?
Gabriela de Queiroz:
Yeah. No, that’s a great question, which I do love answering. First of all, you don’t need to know everything. You are going to be overwhelmed because there is a lot of buzzwords, so try not to get overwhelmed by that. Think about what is the minimum that you need to know. Even when we talk LLMs, not a lot of companies are doing that.
I talk to people, I say, “Oh yes, should I know everything that is happening? Everything that is trending?” Sure, it’s good to know, but a lot of the work that you are going to be doing, or the jobs that are open are still for, let’s say data analysis or data science, they still need people working with data, they still need people to do more classical machine learning models.
Try to think about what is the bulk of the problems that companies are trying to solve and then focus on that instead of trying to know everything.
I always joke that the company, of course, they will try to hire the unicorn, the person that knows everything, because it’s so better for them to hire someone with all this set of skills, but it’s impossible. There is no unicorn. They need to hire a team, not only one person. Try to focus on the things that matter the most, that are being asked the most on all the job listing. And one thing that I want to share is, also if you are learning, if you are learning something, try to find the source of, maybe you like regular books, maybe you like video, maybe you like tutorials, try to find one way and then go for it.
Like what you see here? Our mission-aligned Girl Geek X partners are hiring!
- Check out open jobs at our trusted partner companies!
- Watch more ELEVATE 2024 videos from the event, or just the “Best Of 2024” Videos!
- Does your company want to sponsor a Girl Geek Dinner or Virtual Conference? Talk to us!