Over 100 girl geeks joined networking and lightning talks from women working in engineering, product, and design at the sold-out Grammarly Girl Geek Dinner at Grammarly’s office in downtown San Francisco, California on August 29, 2023.
Grammarly women shared lightning talks about building GrammarlyGO, Grammarly’s new contextually aware generative AI communication assistant that allows you to instantly compose, rewrite, ideate, and reply. Grammarly is hiring!
Table of Contents
Welcome – Angie Chang – Founder at Girl Geek X – watch her talk or read her words
Fireside Chat – Heidi Williams – Director of Engineering at Grammarly with – Charlandra Rachal – Technical Sourcer at Grammarly – watch the fireside chat or read their words
Building GrammarlyGO From Zero To One – Jennifer van Dam – Senior Product Manager at Grammarly – watch her talk or read her words
Engineering GrammarlyGO – Bhavana Ramachandra – Machine Learning Engineer at Grammarly – watch her talk or read her words
Designing GrammarlyGO – Sarah Jacczak – Brand Designer at Grammarly – watch her talk or read her words
Like what you see here? Our mission-aligned Girl Geek X partners are hiring!
- See open jobs at Grammarly and check out open jobs at our trusted partner companies.
- More Grammarly Girl Geek Dinner photos from the event.
- Does your company want to sponsor a Girl Geek Dinner? Talk to us!
Transcript of Grammarly Girl Geek Dinner – Lightning Talks:
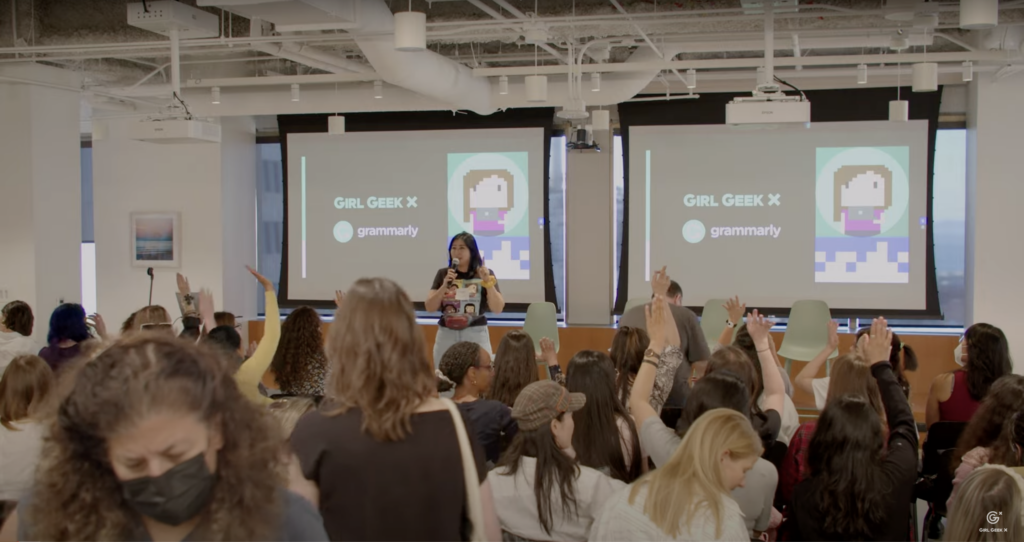
Angie Chang: Is [this] your first Girl Geek Dinner? Wow, that’s a lot. How many of you have been to more than five Girl Geek Dinners? Yay! So good to see everyone. My name’s Angie Chang, in case you didn’t know, and you could tell by the t-shirt, I am the Girl Geek X Founder, and started Girl Geek Dinners in the Bay Area 15 years ago, so we’ve been doing events like this at hot tech startups up and down from San Francisco to San Jose, and I’m in the East Bay, so I wish there was more events over there as well. Tell your employers they need to have one of these showing off their amazing women in tech and product.
Girl Geek X founder Angie Chang welcomes the sold-out crowd to Grammarly Girl Geek Dinner on August 29, 2023 in San Francsico! (Watch on YouTube)
Angie Chang: I want to say thank you so much to everyone at Grammarly for helping put this event together. They have been so amazing and supportive and they’re definitely hiring, so please talk to someone here that has Grammarly on their shirt. They’re very friendly, so I’m going to say thank you for coming and hopefully you’ve made a lot of good connections. I know I’ve seen a lot of people talking to each other and I hope you have LinkedIn with each other or Facebook or whatever people are using these days, and continue to stay in touch.
Angie Chang: A lot of us are in this industry working to keep women in tech and I think that involves all of us together, so thank you. Keep coming back to events! Keep giving each other job leads! Keep poking other girl geek to get in the car ride together to get to that event after work when we’re all tired! Thank you for coming! I hope you learn something, make a new friend, and have a good night!
Charlandra Rachal: Thanks, Angie. I’m super excited to kick things off and host this fireside chat with director Heidi Williams, who’s been very involved in building our generative AI features for enterprise. Heidi, welcome!
Heidi Williams: Hi. Thanks for having me! Great to see you all here. It’s awesome. Full crowd!
Charlandra Rachal: Yeah. For those who aren’t super familiar with Grammarly, can you give us a quick overview of our company and our product?
Heidi Williams: Sure. I like to make a joke that either people have never heard of Grammarly or they love it! I know I talked to a few folks already that love it, but for folks who aren’t familiar, we are an AI-enabled writing assistance that helps with your communication wherever you write, and I do mean everywhere. Our mission is to improve lives by improving communication. Earlier this year, we also launched our first generative AI product to help you with even more writing and communication assistance beyond just revision, but also getting into ideation and brainstorming and composition and comprehension. It’s been really fun to see the product evolve in the time that I’ve been here.
Charlandra Rachal: I hear that you just celebrated three years here, so woo woo! Three years! Can you tell us what brought you here and what really keeps you here?
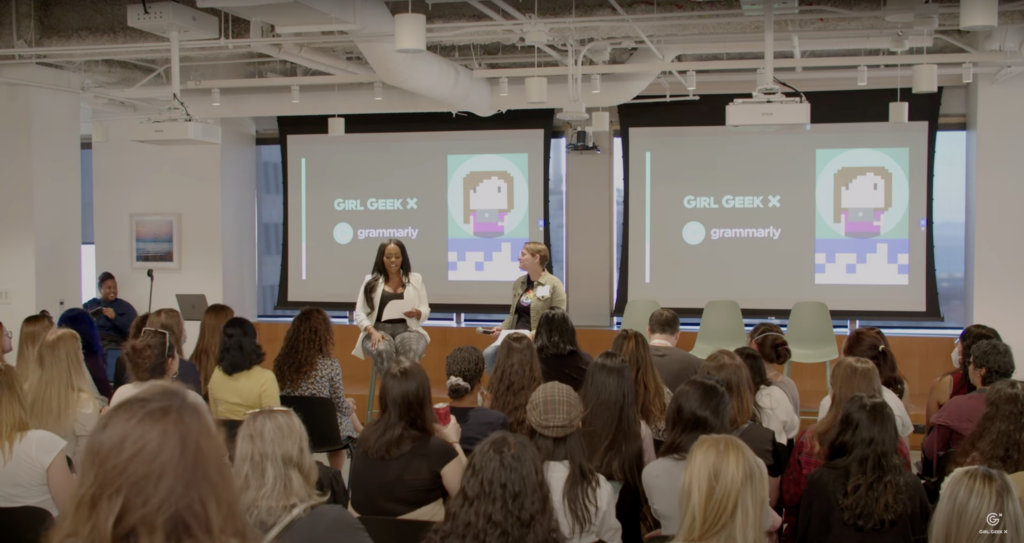
Grammarly Technical Sourcer Charlandra Rachal and Director of Engineering Heidi Williams welcome the audience at Grammarly Girl Geek Dinner. (Watch on YouTube)
Heidi Williams: When I was speaking about the mission, improving lives by improving communication, I do feel like I got to a point in my career, I’m a little farther along maybe than some of you, that I really wanted to work on something impactful and I feel like Grammarly more than any other place, it resonated with me that improving lives by improving communication is so real. It’s not a fake slogan because communication is what makes us uniquely human.
Heidi Williams: I was excited about the idea that we’re not just a platform to help you communicate more effectively, but also to help educate you along the way, especially thinking about things, like insensitive language or bias, there’s an opportunity to help educate people about the possible impact of their words that they may not even know is having a negative impact on someone, and so I got really inspired about the mission.
Heidi Williams: I’m also a word nerd, so that part was really fun as well. I think what keeps me here, is that everyone is so excited about the mission and the people. I think our values are amazing. We really live by our values, we hire and fire by them.
Heidi Williams: The last thing I’ll say is, we’re an amazing size company, where there’s still interesting problems to solve, but we’re small enough that people can really take the initiative if they see a problem that needs to be solved, or they want to advocate for something to change in some way, they’re really empowered to do that. I love being at that size company and our values really help us be successful doing that as well.
Charlandra Rachal: Nice. I like you mentioned initiative and impact. Do you have any sharing stories that you can share that where you seeing either yourself or someone else really make impact?
Heidi Williams: I have three examples if you’ll bear with me for a minute, but I see it all over the place, and it’s not just in the product. It’s about our organization, our culture. There’s an engineer on my team, her name is Lena, and she recognized on the product side that engineers were struggling with a certain pattern of ‘how do I reliably save settings about the individual, about their team and their organization about specific features. Then if I have all of these settings, how do I combine them and know which setting to apply at any time?’
Heidi Williams: She interviewed a bunch of engineers, realized it really was a problem for folks, and then proposed a new project called the Settings Registry, and advocated for it to be on our roadmap. It’s been exciting that she could spot an opportunity and a challenge for our developers and really advocate for that. That’s exciting!
Heidi Williams: The second one, I actually led an initiative where I noticed that I love our hiring process, but I noticed that we had one particular gap, which was that we didn’t necessarily have an interview where we asked people about their experiences. We ask about their knowledge, but we don’t ask, ‘what is the proudest thing that you ever built and tell me how it was designed and what did you learn?’
Heidi Williams: I noticed similarly that we weren’t necessarily getting the accept rates from underrepresented groups that I thought we should be getting, and advocated that this might give people an opportunity to talk about themselves, and for folks who aren’t used to bragging about themselves, that might not come out in a normal interview, but if you give them an opportunity to talk about themselves, then they can actually show off how good they are at stuff, which is exciting.
Heidi Williams: That pilot was successful, showed that we greatly increase the accept rates for folks from underrepresented groups to a really high degree, and now we’ve rolled that out as an interview across the engineering organization, so really proud of that.
Heidi Williams: The last one I’ll mention related to culture, Bhavana, who you’ll hear from later, identified an opportunity that folks were looking for mentorship inside of our women in tech group, and so she started a pilot with a few other folks to introduce an internal mentorship program for women in tech and we’re kicking that off in September.
Charlandra Rachal: I love that. Yes. I feel like the last two really spoke to me, especially being in recruiting so I love that a lot. Now, Grammarly continues to expand in its enterprise space. How do you drive value for Grammarly business with generative AI?
Heidi Williams: It was very exciting to see our generative AI product come out. A little bit of context: the part of the product that I’ve worked on is Grammarly Business, which is our B2B product for teams and organizations.
Heidi Williams: As we all know, communication is not a one person sport. There’s a team dynamic, there are team norms, there’s organizational knowledge that are part of the communication that you have at work. We looked at opportunities for how to incorporate organizational knowledge.
Heidi Williams: We have a feature called Knowledge Share that helps you define terms, definitions related links, key people, and then we can use that as part of the generative AI output to help you have something that knows something about your organization instead of maybe a more generic response.
Heidi Williams: We did things like that and then incorporated some of our Grammarly business features like style guides and brand tones, which help you speak with a consistent voice, and brand tones in particular, you can have a response from our generative AI product, and then choose ‘make it sound on brand to my company’.
Heidi Williams: That was a way that we could really make the information, both the information and the tone be tailored to your organization.
Charlandra Rachal: Nice. Well, I heard that there was some quick turnaround times. Can you tell us more about that?
Heidi Williams: It was definitely felt like this huge opportunity, this huge moment where a lot of folks are talking about generative AI and it’s an area (LLMs) we’ve been investigating for a long time and understanding what their capabilities and limitations were and whatnot, and so I think we really rallied as an engineering organization, and I think the way that we were able to turn things around quickly really came from our leadership approach, which is the idea that we really want to empower teams to make the best possible decisions on the ground.
Heidi Williams: The way to do that is to help with transparency and sharing context around ‘what are the business needs, what are the product needs, what are our customer needs, what problem are we solving for the user?’ Let me give you all of that information, all of that context. At the end of the day, if you need to choose, should this be a radio button or a dropdown or this should work this way or connect with that system, you can make that decision because you have all of that information. Really trying to be transparent and share context so that people are empowered to make decisions on the ground and not feel like they’re stuck with somebody else making decisions and kind of blocking them from things.
Charlandra Rachal: I hear you that you mentioned customer feedback. Do you have any feedback that you’re able to share with us?
Heidi Williams: Sure. You’ll hear more about it in one of the talks today. We did run a survey after launching GrammarlyGO and wanted to know how are people using the product and what’s working and what’s not working. Through that feedback, one of the themes that we heard was that ‘it didn’t sound like me’.
Heidi Williams: We started investigating – ‘how do you tailor the output to sound authentic to you?’ And it sounds, I see a lot of head nods, that resonates and what not. We invested in an area called My Voice and figuring out how to have your own voice profile and use that for all of the responses that are generated, so it’s more likely to sound like you than not and saves you an extra step for trying to even interpret what your own voice is. We can actually help you with that, so you’ll hear more about that when Jen talks about it.
Charlandra Rachal: Great. Well, I know this is one question that I know a lot of people probably want to ask but probably wouldn’t ask, but what would you say really sets us apart from our competitors?
Heidi Williams: Yeah, I was talking to someone ahead of time who asked this question, I’m like, oh, you’ll have to wait. <laughs> Great question. There’s one thing. First of all, I mentioned earlier, we work everywhere and that is one difference from some of the other products that are out there. We work in every writing surface, desktop web, and so we can be right in line for where you’re already doing your thinking, your writing your communication, so that’s certainly one.
Heidi Williams: The two I wanted to really call out, which I think are kind of reinforced by our engineering culture, is our important focus on security, trust and privacy, and also responsible AI. Because at the foundation of everything we do, we really want our customers and users to trust us with their writing and to feel like we can do things to make personalized experiences and what not, and so, what’s interesting to me, I feel like more than any engineering organization I’ve ever been at, because we are so mission-aligned, we recognize we have this huge responsibility to our users to be thoughtful about their data and their privacy, their security.
Heidi Williams: I feel like we care a lot about security maybe earlier than most engineering teams where at the very end before you ship security goes, ‘oh, not yet!’ And you’re like, ‘oh, I can’t’. The whole idea that engineers will advocate for, am I doing this right from the beginning, and wanting to make sure that’s so they’re proactive about asking for feedback about security and privacy. Or even there was a scenario where we had an idea about a feature and people are like, ‘That feels like it might invade privacy. Can we talk about that before we launch it?’
Heidi Williams: I really loved that people could bring that up and that we’re all trying to achieve the same thing, and so it’s a very fair question and let’s make sure we’re holding that to high regard.
Heidi Williams: Then on the responsible AI side, I think we’re so lucky to have an incredible team of linguists who can help us beyond what other competitors can do who don’t have a team of linguists where we can help sort of filter things like the inputs to generative AI to make sure that people are not asking for something harmful, but also that whatever they type in, they’re not getting harmful responses, which are either insensitive or inflammatory or traumatizing in some way.
Heidi Williams: I love the fact that we have the capabilities of being able to create these filters and create a safe environment for people to use these large language models, which have who-knows-what in them. Love that we are actually able to do that. We’ve also been able to build that not just through humans, but figuring out how to build automation and testing and all through the development process help you understand that you’re not going to create a feature that unintentionally create some sort of biased output or something like that, and so just tremendous examples over our long history in this of finding ways to make sure that we are building a product that is responsible and then also keeps everybody safe, secure, and all their information private as well.
Charlandra Rachal: Nice. Well that was fascinating, right, everybody? Alright, so we are going to dive deeper now to exactly how our generative AI features were built. As a heads up, we are going to ask for questions at the end and I’ll bring up all of the speakers including Heidi herself. For now, welcome Jennifer van Dam, who’s a senior product manager here!
Jennifer van Dam: Hey everyone. I’m Jennifer van Dam, product manager here at Grammarly. I’ve been here for three years and I worked on our features like emotional intelligence, tone detection, tone rewrites, inclusive language, and most recently I helped build out our generative AI product, GrammarlyGO, which I’ll be talking about today, so super excited to take you all through the journey.
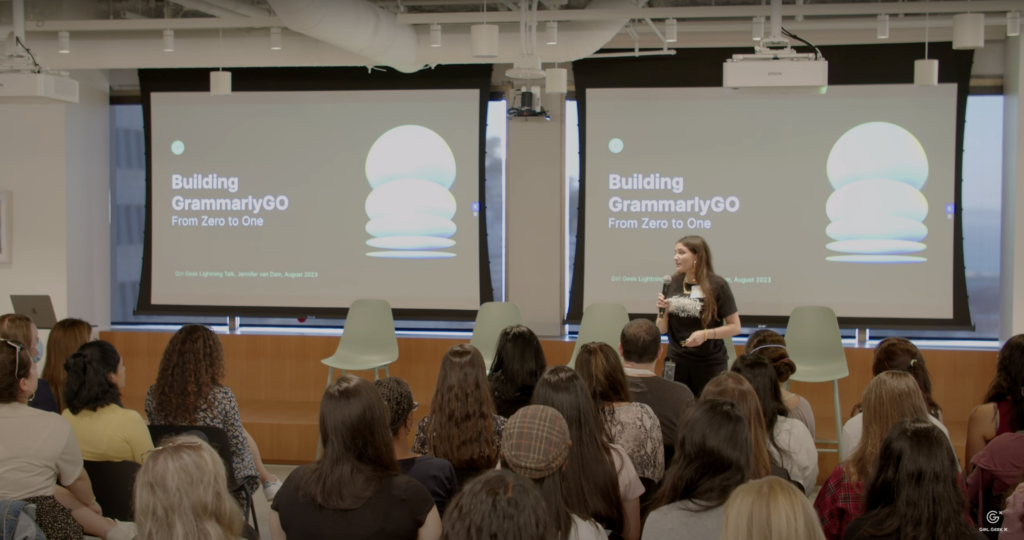
Grammarly Senior Product Manager Jennifer van Dam talks about building the generative AI product GrammarlyGO from zero to one. (Watch on YouTube)
Jennifer van Dam: First off, I want to give a huge shout out to my fellow girl geek PMs that helped build GrammarlyGO together with me. We were a team of three PMs leading multiple product efforts. Specifically, my product focus was on the UX and also on the zero to one stage, so figuring out the UX framework and the zero to one building process. That’s what I’ll dive in deeper today. First off, I wanted to start with a refresh of Grammarly before GrammarlyGO.
Jennifer van Dam: What Grammarly has been focused on for many, many years is helping make your communication more effective by proof writing and proof reading and editing your writing. Anywhere you write, let’s say you’re writing an email, a message, a Google doc, Grammarly will read the text that you have written already and make sure it’s correct and clear and delivered in a way that you want to come across. But, we have a big mission of improving lives by improving communication, so we fully were aware that this is a small part of communication that we want to help with, and we’ve had many dreams beyond proof writing and editing.
Jennifer van Dam: One big user problem we always heard, for example, was the ‘blank page problem’. For years, we’ve heard that our users really struggle with the inception stage of communication – the writing, getting those initial ideas on paper – and it was a huge productivity blocker. That’s just an example from user problems we’ve been hearing for years, and we always dreamt about solving it, and we were super excited with this recent technological leap that with generative AI – now we have the technology to solve all those user problems we always dreamt about.
Jennifer van Dam: That’s how we built GrammarlyGO. We went from proofreading and editing towards helping solve composition, brainstorming, and all these new use cases, which was really, really complex, because we went from a decade of in-depth expertise of rewriting, towards composition and brainstorming, and we had a pretty aggressive timeline as well. This was super, super challenging.
Jennifer van Dam: What made it really challenging? First of all, it was zero to one. We had no prior experience how this would land with our users and there was no data we could rely on, so we had to make a really risky decisions because we went from a proven product concept with product-market-fit towards a huge uncertainty and risk area, which it was really exciting, but super, super challenging. How can we predict how it will be received with the absence of data?
Jennifer van Dam: Essentially we really had to take on a beginner’s mindset to solve these new use cases and almost operate like a startup again to build this new product from scratch, but we’re also an established company – pretty big – and we have millions of users, 30 million daily active users that have a super high bar of our product. We were building zero to one moving fast, but also had a very high bar we wanted to meet for our users in terms of quality, responsible AI, and security that we wanted to deliver.
Jennifer van Dam: How do you solve such a huge, huge problem? What we did was, let’s just start with the earliest draft possible, and get it out – get it out to users. What we did is we created this really highly-engaged alpha community, and we built very early prototype, and we shifted and we asked for continuous feedback, and it was really, really engaged community that would give us feedback super fast and inform next iterations. We really focused on the core experience before we wanted to invest in any type of polish or any type of design polish, we made a commitment – let’s not focus on that. Let’s figure out the UX framework.
Jennifer van Dam: We had a big challenge. How do we create a UX where someone can brainstorm and compose something from scratch? What is intuitive? What will land with our users? To give you an example of the fidelity of prototypes, what we did is we started in grayscale, because we made a commitment to figure out the framework, before deeply investing into building something out, because we weren’t sure if this is the version to commit to.
Jennifer van Dam: This turned out to be a great idea because we did end up throwing away a couple of prototypes, and the third prototype was the one that we felt landed the most and that we committed to building out and refining, which was of course a huge process as well and took us a lot of time. Sarah will actually be giving a fascinating talk later about all the design and brand work that went into polishing this prototype, so I won’t go too deep into that.
Jennifer van Dam: What was really cool about this prototyping stage is, the user empathy led to innovation. We came up with things that we didn’t necessarily plan from the start. One thing we kept on hearing when we were asking for feedback on the UX and was it intuitive to compose and brainstorm?
Jennifer van Dam: A lot of the feedback we were getting was ‘it just doesn’t really sound like me’. And that made people drop off. They would compose an email or a document, but it didn’t sound like something they would write or want to use, so this was a huge risk of people dropping off and also, it wasn’t of the quality we wanted to meet. This led us to come up with the voice feature that Heidi talked about with before.
Jennifer van Dam: This is a classic example – it wasn’t on our roadmap from the start, but it really, being in tune with the user made us come up with this feature. I remember when we launched our first basic version of it, how excited everyone was, and that made us realize how important voices in generative AI – and it led us to much deeply invest in this area, so we’re keeping investing in this and also it helped actually become an important competitive differentiation.
Jennifer van Dam: To take it even further, we would also hear from users, okay, now it sounds like me, but in this situation it doesn’t sound how I want to sound, which was also a really hard problem. We heard this a lot in the email reply use case, and what we came up with is harmonizing your voice preference with your audience as well. Let’s say, I prefer to sound casual maybe 80% of the time, but I got this super formal email, it would be a little bit awkward if I replied casually there.
Jennifer van Dam: We also created a model that looks at the context of your communication and your audience, and harmonize that with your voice preference so it doesn’t diverge too much, but lands somewhere in the middle. This was an awesome, awesome project and Bhava is going to do a much deeper dive into replies after this.
Jennifer van Dam: Looking back, this was a huge product, and when I reflect back on what were the things that made it successful, I think first of all the team was really, really important when we started this project because building in a zero to one, very high ambiguity, it’s not for everyone. It can be quite chaotic.
Jennifer van Dam: We started with a very small team that was comfortable with iterations and ambiguity and okay throwing away work for the sake of learning. We intentionally kept this team very, very small until we resolved the main ambiguities, we started to scale up the team a little bit or slowly.
Jennifer van Dam: We were very intentional about the initial zero to one stage, and then scaling the team, and we had a lot of high alignment and energy because of this, because the people on the team were excited about these problems.
Jennifer van Dam: We also learned that prototypes are huge to align leadership, because it’s easy to get stuck in discussions, discussing strategy or design flows, but there’s nothing like proving it with real concepts and real user feedback and real prototypes. And then also our transparency principle really helped. We had a ton of cross-functional collaborators and of course it’s inevitable zero to one, there’s going to be all these changes and all these teams are relying on you.
Jennifer van Dam: We were super, super transparent with changes and reasoning and this really helped us creatively problem solve. In the case when there were changes, we would come together and this basically set up a place for innovation with cross-functional collaboration as well.
Jennifer van Dam: What’s next for Grammarly? At Grammarly, we believe that AI is here to augment your intelligence. That is really our product philosophy. We believe that AI is not here to take over your life or dictate you, but it’s here as a superpower, to help you communicate more effectively.
Jennifer van Dam: This is the product philosophy we’ve taken building GrammarlyGO, and this is our philosophy with all our next products and features that we’ll be launching. I can’t share too much about it, but I can share that this is the philosophy we take in building the next features that we’ll be releasing. Thank you. Alright, next up is Bhavana who’s going to be talking about the fascinating project called Quick Replies.
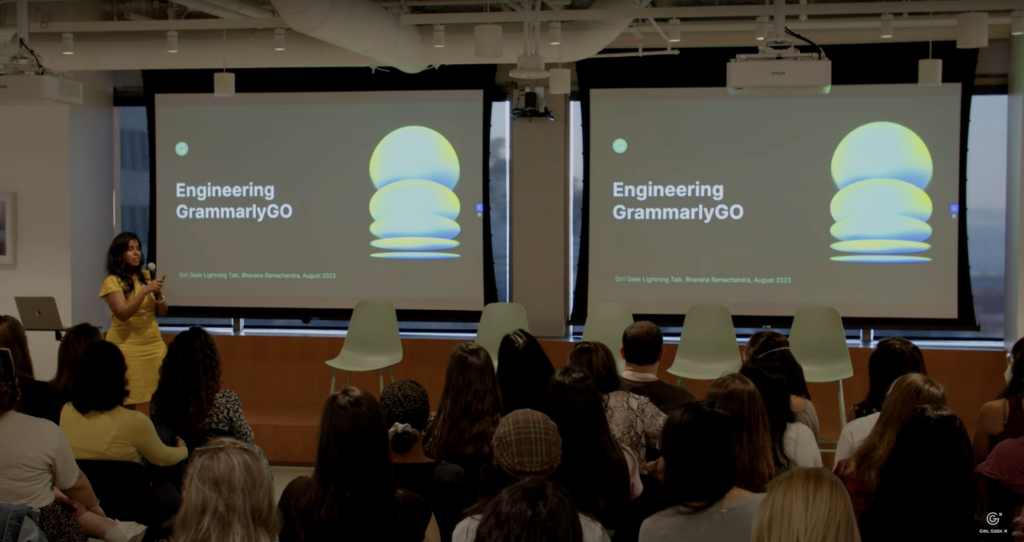
Grammarly Machine Learning Engineer Bhavana Ramachandra talks about engineering the generative AI product GrammarlyGO at Grammarly Girl Geek Dinner. (Watch on YouTube)
Bhavana Ramachandra: Thanks Jen for that awesome overview. Jen spoke about how Grammarly expanded into the user’s writing journey, and we’re going to take a small detour into one of the features that we built, which was Quick Reply, or replying quickly to emails. My name is Bhavana, I’m an ML engineer at Grammarly. I’ve been here for about three years. I was one of the many engineering geeks on this project. There are a couple of folks here in the audience today – Jenny’s here, Yichen is here, yeah, wanted to give a shout out to the team.
Bhavana Ramachandra: Today, I’ll be really talking about foundations in motion and with respect to the quick reply feature. Jen touched upon this previously. We have invested quite a bit in understanding what our users want in terms of their writing, and we were looking at expanding into this user journey, so really we built a lot of fundamental understanding over the years that helped us accelerate into the new product areas that we wanted to go into.
Bhavana Ramachandra: Another shout out is that, the team that worked on Quick Reply, all the point people, all the cross-functional people, were women. We have an analytical linguist, computational linguist, ML engineer, senior PM, and interestingly this has not been my first project when we were all women but yeah. Four foundations deriving from two projects that I have worked on coming into this one. One was Tone. Jen mentioned she has been working on Tone as well.
Bhavana Ramachandra: I’ve been here for three years. She’s been here for three years. Tone was our first project together, so Tone was one of them as well as Recap, which is our investment in 2022 to really go beyond the writing phase and to also to the reading phase to help users read faster so that we can help them write better. With the respect to tone, this was the first version of this. We also have tone rewrites, but this one helps users identify the top three tones in their text so that they can reflect on if that’s exactly how they want to sound.
Bhavana Ramachandra: Zooming into what was the fundamental understanding we built in each of these projects. The first one I’ll cover is Tone, and the three areas we invested is product definition, quality and our AI. For product definition, some of you might be thinking like, ‘Hey, this sounds like sentiment analysis and that is a pretty well solved problem’ but really our product team tries to think about what is the user value of sentiment. If you look at user text, honestly, eight out of 10 times users sound positive. That is not helpful to know.
Bhavana Ramachandra: What the product team did was actually define 50 tones over different aspects of your writing that’s actually helpful for you to know. Do you sound optimistic? Do you sound direct? Do you sound confident? Do you sound worried? Do you sound concerned? The product team really came up with a wide range of tones. In terms of quality, we iterated quite a bit over it and during this phase we actually came up with three levels of hierarchy.
Bhavana Ramachandra: When you have 50 tones, especially if you’re building models for 50 tones, it’s a bit hard – one to get data and to make sure you’re iterating over quality of all of ’em. The way we tackle this is, we define three levels. We have the tone at the really granular level, we have the sentiment at the highest level, but we also came up with tone groups that was maybe around, eight tone groups, and that helped us identify quality at different levels. Then, we really try to nail quality in terms of what is the user values.
Bhavana Ramachandra: Now as an ML engineer, I like to see quality always improving, but is it really worth it to invest in taking one tone from 90 to 92% or is it better for us to improve on a certain tone group that is really valuable to our users?
Bhavana Ramachandra: That’s the kind of trade-off that we had to make and then we really derive over time. I also want to mention our AI is one of our biggest tenants as Heidi mentioned. In this case, this feature was one of the first few pieces to pilot our sensitivity process. The REI manager today was during this process shaping up our formal sensitivity process. We’ve always done it and I think she was making that a very formal process.
Bhavana Ramachandra: Apart from that, we also wanted to make sure that any tone suggestions we make – because we have a varying level of quality, we did want to understand – what are the sensitive cases, and what is our risk of quality with respect to sensitivity. That’s something that we understood during this project as well.
Bhavana Ramachandra: The second one is Recap, which was our comprehension project that we worked on in 2022. Here we were going beyond the writing journey into the reading journey of the user. We invested a lot in understanding the user problem. We had many, many discussions about certain areas that surprised me that I’ll get into. There were also technical challenges because now we needed to again look at the context outside of the text that you’re writing. Where is it? Where are we getting this context from? And then we had a whole new set of ML problems, which is exciting for me.
Bhavana Ramachandra: For the user problem, I wanted to touch on two things. Delight versus value. We wanted to provide summaries and so we identified emails and we wanted to provide summaries as well as to to-do items. But does it really make sense in all use cases? For example, if you have a one line email, it doesn’t make sense to summarize that.
Bhavana Ramachandra: Or, if you have a social promotional email that says, sign up now, that sounds like a task, but all of us know that’s not really a to-do item for any of us. These are the kind of gotchas that we were like, ‘oh, we have a model, but is it actually useful in all cases’ or ‘how long should a summary for a really long email be’ versus ‘a one paragraph email’ be? These are the kind of things that we iterated over quite a bit. And also understanding the context and intent of the user.
Bhavana Ramachandra: Imagine you have an email, an announcement to your entire organization. If you’re a manager versus if you are an engineer versus if you are in design, you might have different takeaways from that email. Trying to understand a bit more of what is that context and what is the intent of the user.
Bhavana Ramachandra: We also solved a lot of technical challenges. Again, shutting out like our AI is one of our biggest pillars. Privacy is also our biggest pillar. We are very, very cautious about what is it that we are asking users to share with us and are we really providing value from it? Before this we didn’t look at the context of the user because we looked at suggestions of what they were writing. Now we wanted to provide value from that, so then we had to update our privacy policy and we also had to update our client side logic to derive this context.
Bhavana Ramachandra: Coming down to the ML problems itself, like I said, there were two things that we were trying to provide – summarization – as well as – task extraction – or to-do list, but because we were talking about delight versus value, context and intent, we also invested in a couple of different areas, including signature detection, intent understanding, and email taxonomy. Trying to understand what is the context of the user – that was more of email taxonomy and intent understanding, and signature detection really helped us. When you look at emails sometimes especially short emails, if the signature is longer than the email, then the model sometimes gets tripped up.
Bhavana Ramachandra: This is true for generative AI as well because yeah, for many different reasons, sometimes models are not perfect, so it helps to help them along the way, and signature detection was one of those areas.
Bhavana Ramachandra: In all of these areas, we spend time annotating our own data sets because email is a space where data sets are not as public, so this was one where we had to understand what data sets existed, what were the things that we were trying to build. As Heidi said, we have a big internal team of analytical linguists, and they help us identify the data, identify what our guidelines are, and go get us annotations that we can build models with, and these were all the areas that we collaborated with them on.
Bhavana Ramachandra: Putting all of that together, from the Tone project, we knew tone was something that our users cared about that we wanted to bring into this feature. You’ll see it says, ‘Jason sounds caring’, but that’s not like models don’t know to think about that. That’s something we have to prompt them to think about.
Bhavana Ramachandra: All of the tone taxonomy that I spoke about, the 50 tones, that made it into the prompt as well. In terms of the recap project, we really built a reply user – like, who are the users? You might get a hundred emails, but you probably reply to 10. What are these reply use cases is something that we had built an understanding that came into this project as well. That really helped us understand quality for launch.
Bhavana Ramachandra: As Jen said, we were not trying to polish, but we were trying to aim for user value. That meant, are we comfortable with the quality for launch? We know that we’re going to iterate over it, but for launch, does this look good? It’s something we try to understand.
Bhavana Ramachandra: Then, on the client side, a lot of the logic, that we built for the earlier project, got repackaged and reused for this one as well. We were using a new protocol, we were using, so it wasn’t just copy paste, but repackage. And then our AI as always, because it’s a generative AI output, we want to be sure that any output that we’re sharing with our users does not have bias, and some high risk scenarios. That’s something as well that we made sure this feature and the output of this feature goes through.
Bhavana Ramachandra: This was one of the few features we built for launch, but it did get a couple of different shoutouts. I know WSJ called out, we had a lot of users sending us, this was awesome. I specifically wanted to, we had a segment on NBC where Courtney Naples, who is our director of language research, spoke about this and the host in fact called out the feature and mentioned how the output of GrammarlyGO sounds like him, versus OpenAI does not. And yeah, that was a really nice moment for us to see. That’s it. Next off, we have Sarah who will be talking to us through all the explorations that the brand design team did for launch as well as our product.
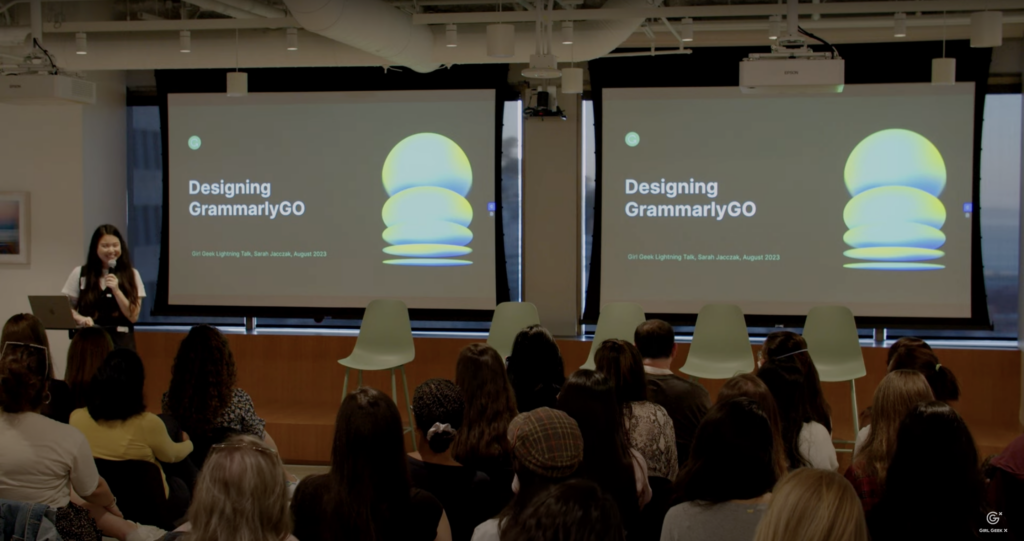
Grammarly Brand Designer Sarah Jacczak talks about designing the generative AI product GrammarlyGO at Grammarly Girl Geek Dinner. (Watch on YouTube)
Sarah Jacczak: Thanks so much, Bhavana. Hi everyone, my name is Sarah and I’m a brand designer at Grammarly. I’ve also worked here for three years and I’m so excited to share the brand design team’s work and show some of the behind the scenes process of the GrammarlyGO launch.
Sarah Jacczak: To start off, I want to intro the go-to-market design team. The team consisted of product brand designers, motion designers, content designers, brand writers, design researchers, and design operations. This was a complex launch and we were designing something completely new, and there were a lot of moving pieces and constant changes. On top of that, we needed to move fast. Having a team with a wide range of expertise, it allowed us to work quickly and collaboratively, and we were able to impact areas across product, brand, and marketing for this launch.
Sarah Jacczak: I want to give a special shout out to the brand and content designers and brand writers. I’ll be sharing some of their incredible work on the GrammarlyGO identity and campaign later on. To give a quick overview of the scope of work, the brand design team worked on in-product systems, a new brand identity that included a new logo and color palette, and a go-to-market campaign toolkit, which included guidance on how to design and write about Grammarly’s generative AI features.
Sarah Jacczak: To do this work, we had to consider how users will interact with this new experience and how we would differentiate Grammarly go from competitors. This required close collaboration with product and engineering teams as well.
Sarah Jacczak: When designing GrammarlyGO, one problem we identified early on was, we needed a way for users to access this new experience. We knew that users were familiar with clicking on the Grammarly icon to open the Assistant Panel and accept writing suggestions, but integrating GrammarlyGO features with this existing UI was not an option for the launch and it was something we would have to address in the future.
Sarah Jacczak: For the launch, we needed to keep these two experiences separate. and we decided to add a second entry point into the Grammarly widget, which would open the GrammarlyGO experience.
Sarah Jacczak: Here are some early explorations of the GrammarlyGO entry point. So on the left we tried two different button designs for the desktop app and browser extension, and we consider it a badge treatment on the desktop app, which has floating widget. The benefit here is that on desktop, the widget wouldn’t be much larger, so it wouldn’t interfere more with text fields.
Sarah Jacczak: However, the visual treatment, it felt kind of like a notification and because of its small size, we were worried it wouldn’t attract much attention. And so we moved on to another exploration. On the right is another exploration where we considered having multiple inline buttons with different icons, so there would be a new unique icon for composed reply and rewrite features, but when prototyping this design, we found that it was a little too cumbersome, and so we decided to simplify it down to one icon for all GrammarlyGO features. And this is what we launched with a single light bulb icon to open the GrammarlyGO assistant window.
Sarah Jacczak: Having one icon as the entry point gave us room to surface prompts that have unique icons. You can see on the example on the right, we have the improve it icon with the pencil, and this prompt appears when a user highlights their text and it gives them a quick and easy way to generate another version of their writing.
Sarah Jacczak: While we were designing how users would access GrammarlyGO, we’re also designing icons. We started exploring icons before we had a name, but we knew it needed to be unique and it would live next to the G icon. We explored a wide variety of approaches. Some were more literal and represented generative AI, like writing and pencils and sparkles and magic, and other explorations were more focused on abstract representations of speed and ideation. But yeah, we could have kept going and going, and this is not even all the explorations, but because of the tight timeline, we had to make a decision.
Sarah Jacczak: We went with the light bulb because we felt it was effective in conveying the new ideation capabilities of GrammarlyGO. We also saw an opportunity to design new product iconography for prompts. These icons would accompany the suggested prompts that appear based on a user’s writing.
Sarah Jacczak: Early testing showed that prompt writing is challenging, and so we prioritize these suggested prompts that are based on a user’s context, and we wanted to make this experience more visual and more delightful. Again, icon explorations range from abstract to literal, but we saw that these icons needed to convey meaning, and also support the prompt compi so we move forward with the literal direction.
Sarah Jacczak: Another discovery was that operating within the new limited color palette was challenging and it didn’t quite feel unified with the existing UI, so we looked to Grammarly’s tone detector iconography, and these emojis, they would appear in the same UI as the prompt icons, so it made sense to create a cohesive experience here.
Sarah Jacczak: We referenced the colors and styling of these emoji to create the foundation for the new prompt icons and here the prompt icons that we designed for launch. You can see they’re literal in that they depict the meaning of the prompt in a simple way, and keeping them simple also ensured that they could scale and be legible at small sizes. We also selected colors and subtle gradients that felt cohesive with the existing emoji icons. This resulted in an icon set that feels warm, friendly, and is hopefully fun to interact with.
Sarah Jacczak: We also needed to consider scalability. There would be hundreds of prompts and we wouldn’t be able to design an icon for each prompt, so we grouped them into categories. Each category has an icon, and within that category are prompts that share that icon. For example, any prompts about writing or composition, we’ll use the pen and paper icon and any prompts about ideation, we’ll use the light bulb and so on. We also identified which prompts we feel would be used frequently and created unique icons for those to add variety and more delight.
Sarah Jacczak: While some of the team was working on iconography and content design in the product, others were working on the identity and go-to-market campaign. Here are some of those explorations – a variety of logos were explored and taglines, as well as graphics for the campaign, and some visual explorations use gradient orbs while others focused on movement and transformation by using over layers of shapes and lines. And for the go-to-market campaign, we created a new tagline as well – ‘go beyond words’. It’s active and it conveys Grammarly’s ability to assist users beyond their writing.
Sarah Jacczak: We also designed a new logo that incorporates a bolder G with a circle forming the O as a nod to the classic Grammarly button. For the GrammarlyGO identity and campaign, the brand design team landed on a concept that uses overlapping shapes to convey transformation and the iterative process where one idea is built on the next. The softness of the gradients also speak to the human qualities, and they’re juxtaposed with hard edges to represent technology, and these overlapping shapes were further brought to life with animation.
Sarah Jacczak: The team also worked on a design toolkit, and this toolkit was shared across the company. The toolkit included logo, color palette, illustrations, photography, motion guidelines, and a library of product examples to be used across the campaign. A style and verbal direction guide was also created to ensure how we speak about GrammarlyGO is consistent. The brand writers provided headline examples based on themes. There was headlines about creativity, such as let your ideas take shape, headlines about productivity, such as ‘discover new ways to get things done’, and headlines about trust like ‘AI innovation with integrity at its center’.
Sarah Jacczak: This campaign was pretty large. We had a lot of requests and a lot of marketing channels to design for, but because the brand and brand writers and brand designers collaborated and built these systems and guidelines, we were able to move quickly and create consistency despite many people working on the campaign production.
Sarah Jacczak: Here are just a few examples of the work created for the campaign. The team created a series of demo videos and animated gifs that show product functionality, and these were used across marketing and PR. The team also worked on onboarding emails, landing pages, in product onboarding, blog posts, ads, and social assets,
Sarah Jacczak: To get a further sense of the scope of work, here’s some numbers from the naming and identity work. Over 500 names were considered, 188 Jira tickets were completed, over 105 taglines were explored, 45 videos explored, 39 product examples designed and animated, and over 230 logos were explored. And so, while these numbers don’t tell the full story, and we had challenges along the way, the team was able to overcome this and collaboratively design a new experience and produce a successful launch in a short amount of time. Thank you.
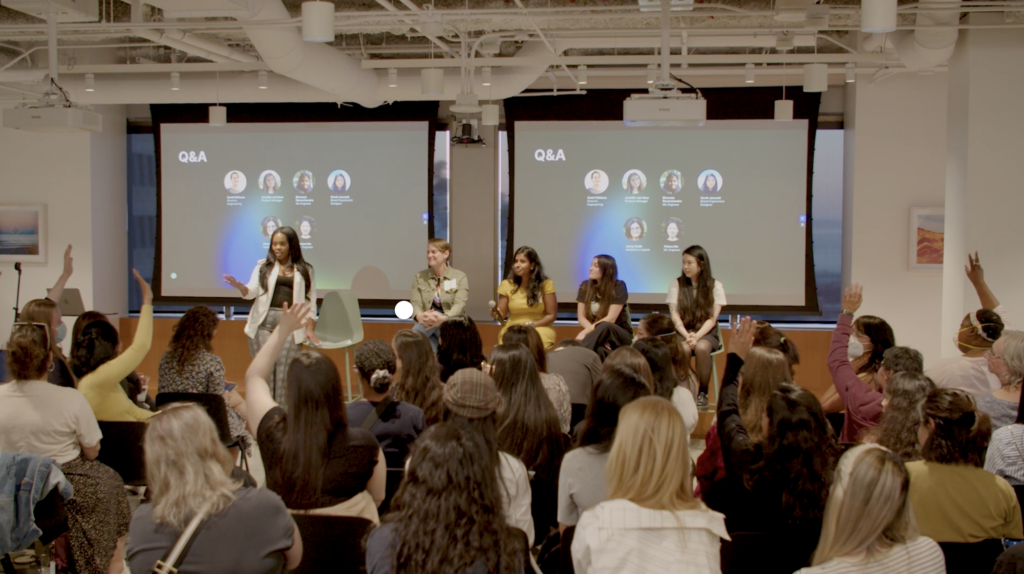
Charlandra Rachal: Thanks Sarah. To all the speakers that put together this incredible presentation, I learned a lot and I work here, so I hope you guys really enjoyed all of that. Let’s welcome back all of our speakers for Q&A. There is someone who is going to be in the audience with a mic, and I see it first hand already, so we will get a mic right over to you.
Audience Member: As mentioned, it was uncharted territory. I was curious how you went about ideating the first project. Was it based on existing user information you had? Was it academic papers? How’d you go about it?
Jennifer van Dam: That’s referencing my talk, so happy to talk more about it. What I mean with uncharted territory is the solution. We knew it was a problem – we’ve been hearing for years that since we started, we hear from our users, they struggle with these communication problems. What was the uncharted territory is the solution and delivering the product in a way that lends and resonate with our users.
Jennifer van Dam: The approach we decided to take is directly into the prototyping stage because we felt it was really important to connect the text and the product to the user. Let’s say, you want to compose an email, we can design and show you concepts, but we need that moment of you writing your text and seeing the output. That’s why we jumped right into the prototyping stage as our way to research the solutions and the design approach.
Charlandra Rachal: There was another hand right here…
Audience Member: Hello, my name is Kate, and probably question also to Jennifer because it was on one of your slides. When talking about prototyping, you were speaking about more empathy and I took a screenshot. Let me see how it looked like there.
Audience Member: ‘Deeper user empathy.’ Can you please elaborate a little bit more on that, how it worked? How did you do it while you were still prototyping, please?
Jennifer van Dam: Yeah, deep user empathy. What I really meant with that was to understand and dive into the types of things our users are trying to achieve. What are the types of use cases that, here’s a prototype, did you use it for rewrites? Did you use it for emails? What were those things?
Jennifer van Dam: We did so many sessions talking to people and getting their feedback to get empathy and then of course we had questions, but then the feedback we got was, ‘oh, but it didn’t sound like me’. This is what I mean by deep user empathy is really getting into the mindset of empathizing what is working and what is missing. That really helped us inform iterations and changes and new features or scrapping features.
Audience Member: Thank you. I would assume that the launch of ChatGPT definitely affected Grammarly. What would be the key learnings? What were the key learnings for you as product leaders from getting the LLMS viral and thanks for the presentations. My name is Maria.
Heidi Williams: One of the things which I think was interesting – we’ve been doing AI for a long time, we’ve used a lot of different technologies, whether it’s rule-based or machine learning, or all sorts of different technologies, exploring LLMs on our own.
Heidi Williams: The biggest thing about ChatGPT that ended up, was actually the discussion in the world about how to use AI as an augmentation tool. Before, you would have to convince people AI was okay and trusted and then all of a sudden overnight everyone’s like, ‘of course you trust it. Look at this’.
Heidi Williams: Now all of a sudden we don’t have to waste time talking about should you use AI? Now it’s about how can we be a trusted partner on how best to use AI and help you be more effective and help you succeed in your job or in your life. It has changed the conversation of ‘should I’ to ‘how should I’, and that’s been interesting and amazing that we can now focus on just solving real problems as opposed to convincing people they have a problem that AI can help with.
Audience Member: Thank you so much. First of all, want to say huge shout out for Girls Geek and Grammarly for putting together. Thank you. That’s a great event. I’m a huge fan of Grammarly. Go now. It finally sounds like Shakespeare in my emails and not like a broken machine. I want to ask you this question. I think Heidi and Bhavana, that’s questions for you. You said that one of the feedback was, ‘it doesn’t sound like me’.
Audience Member: Are you using LLMs to train data which your users are input? And if so, how do you also prevent some data security in terms of, for example, I’m putting something in my email as a product manager about revenue or about some specific of the product, which haven’t been on the market, and I’m always a little bit worried where this data is coming from. Yeah, I think it’s a good question. One is LLMS for make me sounds like it’s me. And the second one is the data privacy. Thank you.
Heidi Williams: I can talk about at least part of it and then if you have things to add as well. Because Grammarly has been around for so long, and that we are a trusted source, we were able to negotiate a really amazing contract with our LLM provider, which means that they don’t train on any data that we send to them. Not everybody could negotiate that, but because we’re Grammarly and we’ve been around so long and have such a big user base, we were able to do that.
Heidi Williams: I feel like that was a huge thing that is very differentiated from just using whatever’s on the market is that they’re not training on any of the data that we send. From that perspective of the data privacy, but if you want to talk more about the my voice and about how we do that and how do we then, if either of you want to add…
Bhavana Ramachandra: I’m going to pass to Jen.
Jennifer van Dam: Could you repeat the question?
Audience Member: Sure. How you make, if we’re not sending data to any LLMs, how you make it sound more like me, for example, GrammarlyGO, always suggesting me to be more assertive, which I think I’m already too much and then I’m like, no, no, no, let’s make it more positive. Yeah, how this happened, how it sounds more like me is like data being trained.
Jennifer van Dam: We look at context and communication patterns, so it doesn’t necessarily train on your data per se, but on the patterns of your communication and the context. That’s how we understand your voice profile across.
Bhavana Ramachandra: To add to that – we understand what your tones you prefer or you use are, but we don’t actually pass that on to LLMs. We had our tone detectors since 2019. We’ve been telling users how they sound for a bit now. We use that information to really update the writing rather than train the LLMs itself with your data.
Charlandra Rachal: I feel like I’ve been neglecting this side over here, so right here in the front.
Audience Member: More of a quick technical question. Do you list all of the tones that you detect for and measure somewhere publicly, or is that behind closed doors?
Jennifer van Dam: We have a homepage that lists a lot, but not all, of our tones. We feel it’s too competitive to reveal 50 plus. But yeah, you definitely can find information about a lot of our tones that we support with tone detection.
Bhavana Ramachandra: Maybe this is a challenge. Can you write enough with Grammarly to find all of them?
Audience Member: The tables have turned. I wanted to direct a question to the second speaker after Jennifer van Dam. Yes, you, um, why does my voice sound like this? Ah ha ha I see what you did there. The question I have more specifically was, when tone is being cited as a suggestion, when you write a sentence and it connotes that, ‘oh, your tone is serious and neutral’, and when you add a word or two and it changes the tone entirely, I’m curious, what quantitative scales do you use behind the scenes to make those on the spot judgements? You mentioned your team had a lot of linguists on it, and I was hoping you could expand on that because that has been an object of curiosity of mine for a while, possibly. Thank you.
Bhavana Ramachandra: Yeah, I’m just going to Jen about… Yeah, I think for, in terms of how do we decide, in fact, when we started looking at rewriting for tone, I think our initial exploration had just neutral and new tone versus in certain cases we were actually able to provide three levels, friend, friendlier, friendliest, but really depended on how much data we’d have. If you can actually, you can be neutral, but you can’t be more neutral. It really depended on the tone and how much data we have.
Bhavana Ramachandra: I think this is a part where our linguist really helped us really dive deep into this and look into each of the tone that we have, get data for each one of these tones. I think for our tone retype explorations, we started with the tones where we had most understanding and first started with two levels and then moved on to three.
Audience Member: Thank you. Hi, my name is Leanne and big fan of Grammarly. My question for whoever thinks that they’re best equipped to answer this is, could you tell us a bit more about how Grammarly is fighting bias, and what are some of those solutions that are currently in place? And maybe thinking about, in the future roadmap?
Bhavana Ramachandra: Yeah. Our AI is one of our biggest tenants, as Heidi said, and we’ve always invested in this area. The couple of things that we have done are actually very, very public. We have blog posts about how we look at pronouns or bias in gender bias in data, and how do we make sure our generative AI suggestions, how do we measure that and how do we prevent that as something that we do? And as Heidi said, this is part of the process.
Bhavana Ramachandra: This is not something that you think about at the end of the day, you plan for this. You plan to have a sensitivity analysis right from the get-go. The other part of this, we’ve published a couple of different papers this year. In fact, in the REI space in, I want to say ACL. Okay, thank you Dana. You’ll actually find a lot of public information. I don’t want to pretend that I know more than I do in this area. I am getting onboarded though. Definitely, we have blogs and papers out there that talk about what are the solutions we have implemented.
Heidi Williams: Maybe just one thing to add to that is part of it is actually cultivating a good data set because you could imagine that you just take, I think we’ve seen this with LLMs as well, you just take the words out there and you might see a bias of a gender bias around when you’re referring to a male, they might be more associated with certain words in the general public than a female. Then you would imagine that percentage wise, it might suggest like, oh, if you’re talking about a man, you must be referring to this, et cetera.
Heidi Williams: We’ve done a good job of cultivating our data sets to help ensure that the data sets themselves are not biased, and that’s a huge aspect of it is just making sure that we’re not having any gender weighting as one example, or it could be racial, whatever it is. There’s just making sure that you have a data set that’s representative and it’s not going to sort of skew things in one direction or another.
Bhavana Ramachandra: Do you want to add to that?
Jennifer van Dam: I wanted to add to that a little bit about how much we care about this investment. We also, besides of course all the deep investments in the modeling, we also have inclusive language suggestions for end users that help basically eliminate gender bias in your language while writing or talking to your coworkers or your team.
Jennifer van Dam:This is an area I also worked on and it’s a really great part of our product. For example, maybe you’re writing, ‘the businessmen are wearing suits’, we’ll underline ‘businessmen’ and we’ll ask if you’re writing to an audience that you want to be inclusive of everyone, maybe replace it with ‘business people’ rather than ‘businessmen’. We also tackled this from the end user standpoint and helping them communicate more inclusively and eliminate bias where they’d like.
Audience Member: <inaudible>
Charlandra Rachal: For those who didn’t hear, the question was, how much do we focus on educating, when people continue to make mistakes in their writing?
Jennifer van Dam: The inclusive language product, I encourage you all to check out because education was a huge part of the product UI and the way we wanted to position it. We always want to be educational rather than forcing you because at the end of the day, the user is in control. You have agency. That’s what we always believe in. We also realized we have to explain why are we saying ‘consider replacing businessmen with business people?’ How do we explain? Because some people don’t realize that it’s so ingrained, you just type it, you don’t really stand still.
Jennifer van Dam: Another example, like whitelist, blacklist, that suggestion, a lot of users didn’t understand why we were suggesting to replace blacklist with blocklist, so we actually focused our UI around education. Why is blacklist/whitelist is perpetuating certain stereotypes, so consider replacing it – and that was a real aha moment because when you say that in a training, it’s different than when in real life you’re writing a text and seeing it and applying it, so it’s actually been really powerful in educating people
Audience Member: To Jennifer and Bhavana. Earlier you mentioned deploying LLM models, initially, were you were skeptical how receptive the users would be and how they would perform.
Audience Member:Can you talk about your A/B testing strategies? Did you roll out to a part of your audience, I mean part of the user base first, and then started gradually increasing, rolling out the new features too, especially the generative AI features? Could you talk about your A/B testing strategy and how did you scale it to the whole user base? And after you employed gen-AI features and these new features that you earlier talked about, how did it impact the revenue subscription revenue and the user base?
Heidi Williams: Start?
Bhavana Ramachandra: I think Jen covered maybe some of the A/B tests. I’ll let her, I can maybe speak to the launch plans, the alpha testing. I can cover that a bit. Especially for projects like Tone where we did iterate over quality quite a bit, we would try to identify one. We had internal annotations. Every time we improve our quality, we do more internal annotations to understand how much of a bump is it? And once we have a fair understanding, we run experiments with Gen AI, we had to take a slightly different process.
Bhavana Ramachandra:As Jen said, we had more alpha testing with users, really deep conversations in terms of understanding what is a useful generative AI LLM feature because we’ve had rewrite features in terms of generative AI for the longest time, but what’s a useful composed feature? What’s a useful quick reply feature? All of that was not really A/B testing. We were building understanding in this case. That was a lot more alpha testing.
Bhavana Ramachandra: Then for the launch plan itself, we have 30 million users, we have five different surfaces, we have extension, we have desktop app, we have an editor, we have our website, and we’re in many different countries, so this to me firstly was the most impressive one, because we had to do a geo launch across many different clients that all have different release cycles, so all of them had to be in sync because we wanted feature parity. We started with certain countries to make sure, one, we can handle the traffic. Two, all the features are looking or performing as they should.
Jennifer van Dam: The difference with how we approach modeling quality, it depends on the maturity of the track. In the zero to one stage we do a lot of offline quality evaluations and make sure it meets our quality bar and the metrics. We don’t necessarily test out multiple models yet, but in the iteration stage we do. One example where we’ve A/B tested our improve it rewrite, which in one click will improve your text, and there was a lot of experimentation we did there with tone behind it and conciseness and what lands the best with improving my text with one click. Typically, we focus a lot on offline quality evaluation of our models, and then in the iteration stages we do a lot of A/B modeling.
Audience Member: After new features, did you see any bump in the overall user base?
Heidi Williams: I can’t talk about specific numbers, but I think obviously there was a lot of excitement and interest in this area. I think we did see that there was new interest, and then also just seeing interest and engagement from our existing users using the product maybe in a different pattern than they had been before as well. It definitely feels like there have been changes, but I can’t speak about specific numbers.
Audience Member: What’s your tech stack typically for the whole GrammarlyGO is hosted on?
Heidi Williams: The tech stack? A lot of different parts of it… it’s hard to answer with a really quick answer. Our particular LLMM provider is Azure OpenAI. And then there’s a variety of tech stacks above that – different things that we’re using for the linguistic side of things, and then there’s Java, there’s Closure, there’s all sorts of different technology stacks and then we run on AWS otherwise.
Charlandra Rachal: Thank you. Then I only have time. Oh, oh, oh. I was coming for you. I know you had your hand out. If you still wanted to answer, we would definitely break the mic over your way. The last I have one time for one more question. Alright, Nancy
Audience Member: Behind.
Charlandra Rachal: She’s coming. Yeah, she’s coming.
Audience Member: Oh hi. Alright. I’ve used Grammarly for a really long time and this may be more of a product manager question because I’m also, I can write circles around everybody, so I don’t really need GrammarlyGO. I’m actually wondering, I’m thinking about, the roadmap further down for advanced writers, people like me who write. What’s coming?
Audience Member: Because I will say now, I use Claude a lot just to be like, Hey Claude, this is what I wrote. What do you think? And then Claude will say, that’s really good or not or whatever. I’m just wondering it’s GrammarlyGO moving in that direction for people who don’t really need help getting stuff on paper or on screen.
Bhavana Ramachandra: These are the comprehension projects that I was talking about. They’re all about trying to understand what the user is reading or what the user has written. For example, tone is something, even if it’s not correction, if it’s not editorial, you still might want to understand how your tone is coming across, especially in cross-cultural communication.
Bhavana Ramachandra: That’s something that’s helpful and in general as well, especially for long writing, we’ve gotten a lot of feedback about, I think this is one area that we were investing in – how we, so we show top three tones and let’s say people use Grammarly to write books or their fictional books, and does it make sense to show top three tones? Then they want a different – so this is the kind of evolution of the features that we see.
Bhavana Ramachandra: Comprehension is one area. In our generative AI in GrammarlyGO, if you actually open it up in a document, we provide a lot of prompts around understanding the gaps in your document, identifying what are your main points. All of these are just comprehension. This is just not how to improve your writing. Rather like this is what’s there in your document. You can review it based on a couple of different dimensions.
Audience Member: I should use Claude and Grammarly. Yes.
Bhavana Ramachandra: That’s the answer.
Charlandra Rachal: Yes. Alright, I wanted to say thank you so much to all of the speakers here and all you wonderful guests. I’m going to give a shameless plug if you didn’t already see, I’m in recruiting and we are hiring! Definitely talk to us, talk to me. I know we’re going to send a link out as well.
Charlandra Rachal: I believe there are more refreshments in the back and everyone is welcome to kind of hang out, chat, network. If you have more questions, I feel like we got through a lot of them without telling all of our secrets, but feel free to pull them aside and ask more questions. I hope you have a great night. Thanks again for coming out.
Like what you see here? Our mission-aligned Girl Geek X partners are hiring!
- See open jobs at Grammarly and check out open jobs at our trusted partner companies.
- More Grammarly Girl Geek Dinner photos from the event.
- Does your company want to sponsor a Girl Geek Dinner? Talk to us!
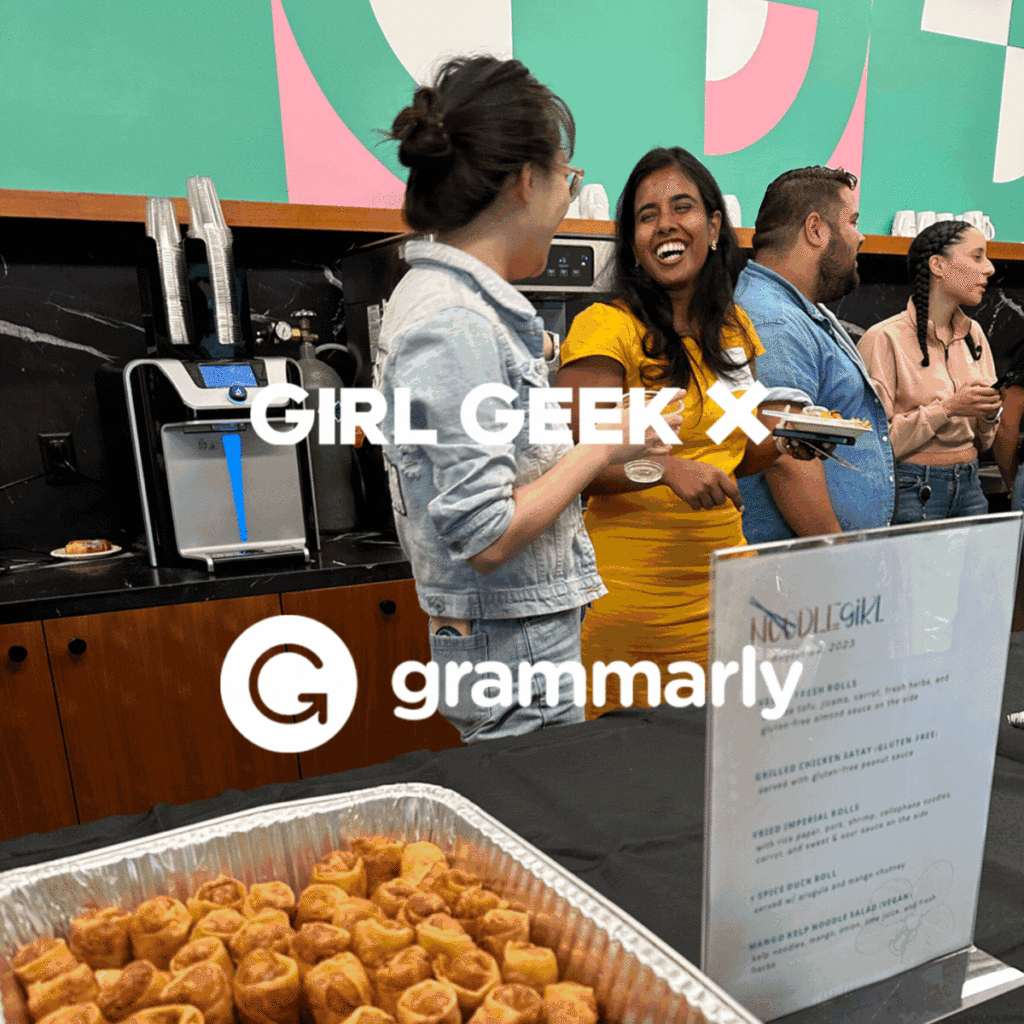