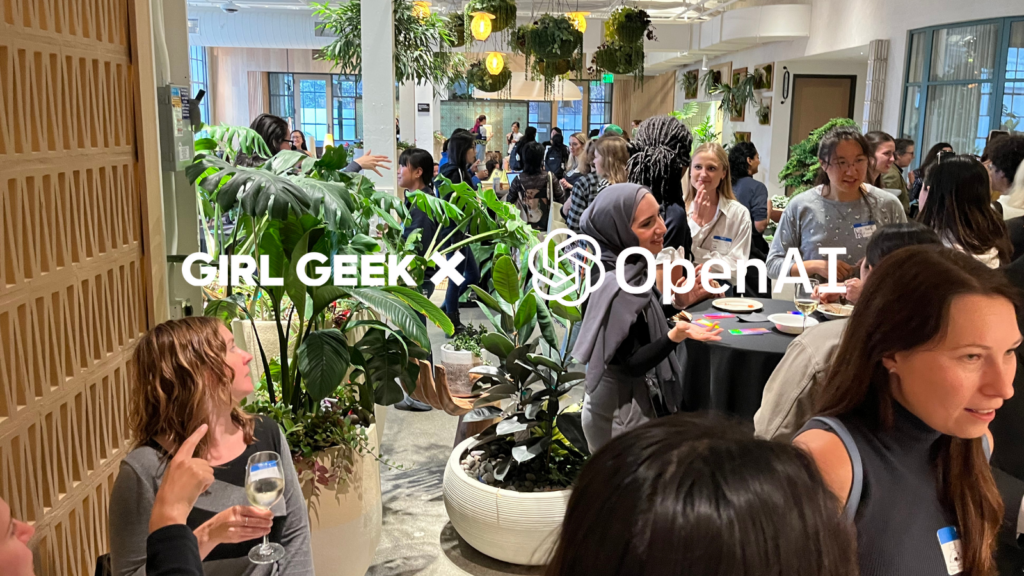
Over 120 girl geeks joined networking and talks at the sold-out OpenAI Girl Geek Dinner on September 14, 2022 in San Francisco’s Mission district.
Hear lightning talks from OpenAI women working in AI with music and deep learning, sharing the power of trying and trying again, how to make language models useful, and much more at the OpenAI Girl Geek Dinner video on YouTube!
OpenAI Residency applications are open! OpenAI is looking for engineers and researchers who are interested in applying their skills to AI and machine learning. Please apply for OpenAI jobs here!
If you have an unconventional educational background, we encourage you to apply to OpenAI Residency (applications are open through September 30, 2022).
Table of Contents
- Welcome – Elena Chatziathanasiadou, Talent Programs Lead at OpenAI, Recruiting & People – watch her talk or read her words
- Multimodal Research: MuseNet & Jukebox – Christine McLeavey, Member of Technical Staff at OpenAI, Multimodal – watch her talk or read her words
- If At First You Don’t Succeed, Try Try Again – Alethea Power, Member of Technical Staff at OpenAI – watch them talk or read their words
- Making Language Models Useful – Tyna Eloundou, Member of Policy Staff at OpenAI, Policy Research – watch her talk or read her words
Like what you see here? Our mission-aligned Girl Geek X partners are hiring!
- See open jobs at OpenAI and check out open jobs at our trusted partner companies.
- Find more OpenAI Girl Geek Dinner photos from the event – please tag yourselves!
- Does your company want to sponsor a Girl Geek Dinner? Talk to us!
Transcript of OpenAI Girl Geek Dinner – Lightning Talks:
Angie Chang: Hello. Thank you everyone for coming tonight. My name’s Angie Chang and I’m one of the founders of Girl Geek X. We started over a decade ago as, Bay Area Girl Geek Dinners, and we’re still going strong. Thank you to OpenAI for hosting us for a second time. We’re really excited to see the new office and invite a bunch of Girl Geeks over to hear these lightning talks on AI and policy and all these things that we’re so excited to learn about tonight!
Sukrutha Bhadouria: Hi. I know you all were still chatting when Angie introduced herself, but she’s Angie and Girl Geek X is basically her brainchild. It started off with Angie looking to bring women together, I’m doing your pitch, Angie for you because I have a louder voice. Some people, they ask me if I swallowed a mic as a child because I’m so loud and I don’t need a mic.
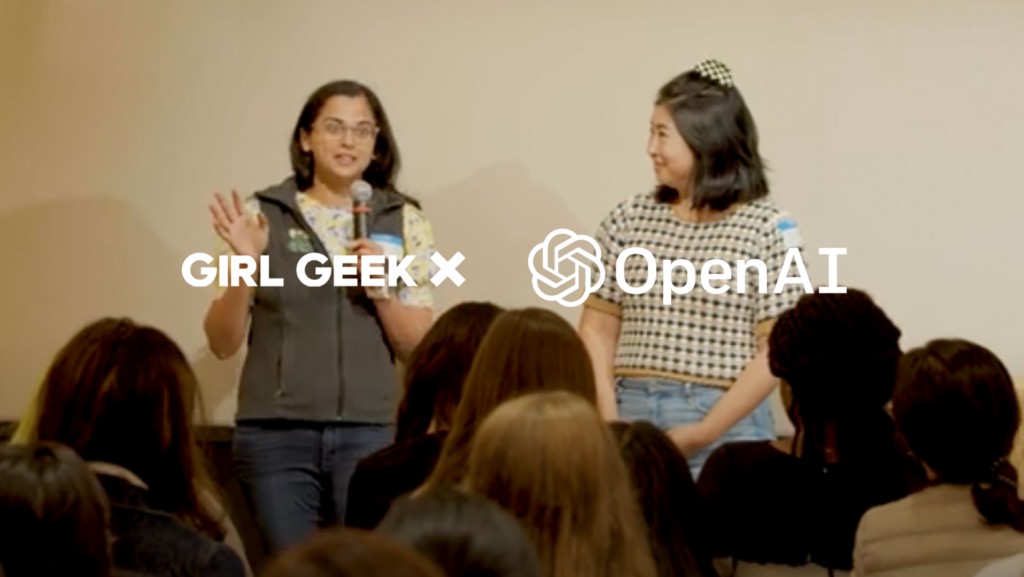
Sukrutha Bhadouria: Anyway, I’m Sukrutha, so Angie started Girl Geek and it was back then called Bay Area Girl Geek Dinners, this was over 10 years ago. And when I had just moved to the Bay Area, looking for ways to meet new people and I found out about Bay Area Girl Geek Dinners dot com at that time, and I tried really hard to meet with Angie, but she was a busy bee doing all sorts of cool things, trying to change the world. And this was way before ERGs existed, right? So people didn’t have a way to connect with the community until they went to meetups.
Sukrutha Bhadouria: And Girl Geek Dinners, at that time, was the one way you could also get an insight into what these sponsoring companies worked on, what life was like. And so it also allowed people to get an opportunity to speak and a lot of the speakers at Girl Geek Dinners were first time speakers. They were too afraid to sign up for conferences. If you go to our website (girlgeek.io), you’ll see all these amazing stats on how since Angie started, there’s been a real shift in the environment in how people are more willing to speak at conferences, due to some of the chances they’ve gotten as a result of speaking at an event sponsored by their company. This organization exists.
Sukrutha Bhadouria: I joined Angie and we tried to change the world together. I’m happy to report that I think we actually did. We rebranded to Girl Geek X, and that’s when the organization hit 10 years. It was a sizable number of people working on it, it was Angie and me and it was just the two of us. And then Angie had this idea to really evolving into a company and so that’s when she started to bring on contractors, more people such as somebody who could take video of our events to make us look a little bit more professional and somebody else to do our website besides me. And we started to do podcasts.
Sukrutha Bhadouria: We started to do virtual annual conferences and we really, really, really were always consistently sold out for our in-person events that would happen at various companies that we partnered with through the Bay Area. Then COVID hit and the good thing is that we had already started to have a global presence through the virtual conferences that we had and we’ve now had four? Five, yeah.
Sukrutha Bhadouria: We used to be carpooling all around the Bay Area together to these events after work and now we are moms. So it’s amazing. We would look up and see amazing people working at these sponsoring companies speak and we’d be like, “Wow, look at them managing their mom life and parent life and coming to these events.” But I just think that it’s now become such a common thing that it’s not as isolated anymore. And I’m hopeful that, you all can come back again and again, because this in person event has really made me really happy.
Sukrutha Bhadouria: I’ve been holed up in my home office today, which is basically a room which also has my… What’s it called? A bike that stays in one place, stationary bike, so it has too many things going on in the room, but I wanted to give a big thanks to OpenAI for hosting us for the second time, for sponsoring for the second time. And I hope that we can keep doing this. So please do get your companies to sponsor and encourage them to do it in person. That’s all I will say. I know I said a lot more than I had planned, but thank you again, and Angie.
Angie Chang: Thank you Sukrutha, for the intro. I guess I should talk up Sukrutha a little more. When I first met her, she was a software engineer in test, and now she is at Salesforce as a Senior Director of Engineering there, so I’m very proud of her. And over the years we… She mentioned we have a podcast, we have annual virtual conferences!
Angie Chang: We’ll be launching a career fair virtually as well, to be announced. And I don’t want to say too much. We have an amazing line up of speakers tonight and we’re going to invite up first, Elena, who is our host for the night from OpenAI.
Elena Chatziathanasiadou: Hi everyone, I’m Elena. I work here and I’m on the recruiting team, I’m leading the Residency program right now. I’m very excited that you’re all here and have joined us together. Really want to thank Angie and Girl Geek X. We’re very excited to deepen our partnership together and to be back in the office here all together, in the new space and to experience this tonight.
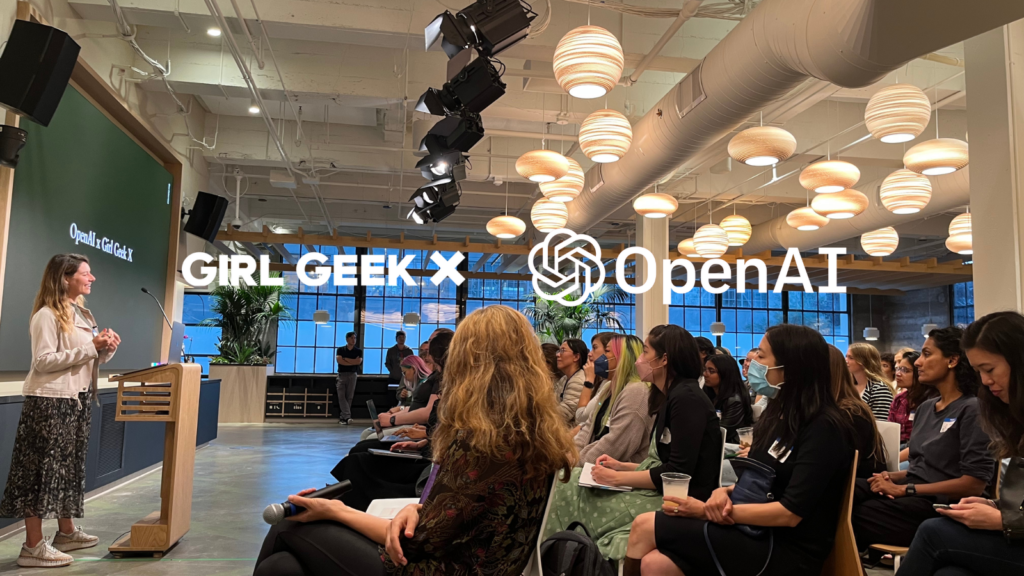
Elena Chatziathanasiadou: We’re very excited about having you here and in terms of what we’ll see tonight, we’ll have a series of lightning talks and then that will be followed by Q&A and then we’ll get some dessert in the area that we were before and then we’ll wrap up at 8:30. But before we get started, I did want to take a moment to make a quick plug and share that…
Elena Chatziathanasiadou: We’re actively hiring for our Residency program and that includes both research and engineering roles and the goal of it is really to help develop AI talent. The program, it offers a pathway to a full-time role at OpenAI for folks that are currently not focusing on AI and are already researchers or engineers in a different field.
Elena Chatziathanasiadou: We’re really excited to hear from you. If you do have an interest in making this career switch, come talk to me after. And we’ll also have full time recruiting team members and positions that we’re hiring for across research product and engineering that we can tell you more about. Please come find us and learn more about the interview process, but also what the program offers.
Elena Chatziathanasiadou: With that I wanted to introduce our first speaker, Christine, who’s currently managing our multimodal team and previously worked on music generation research, created MuseNet and was collaborating on Jukebox. And before that was a classical pianist who transitioned into a researcher as well. I’ll hand it over to Christine. Thank you so much.
Christine McLeavey: Thank you. So yes, it’s really an honor to be here tonight. Thank you all for being here. And this Residency program is near and dear to my own heart, because I first joined OpenAI through, what was then the Scholars Program and the Fellows Program and those are the programs which have since evolved into this Residency program. I’ll put a plug in for anyone who’s considering it.
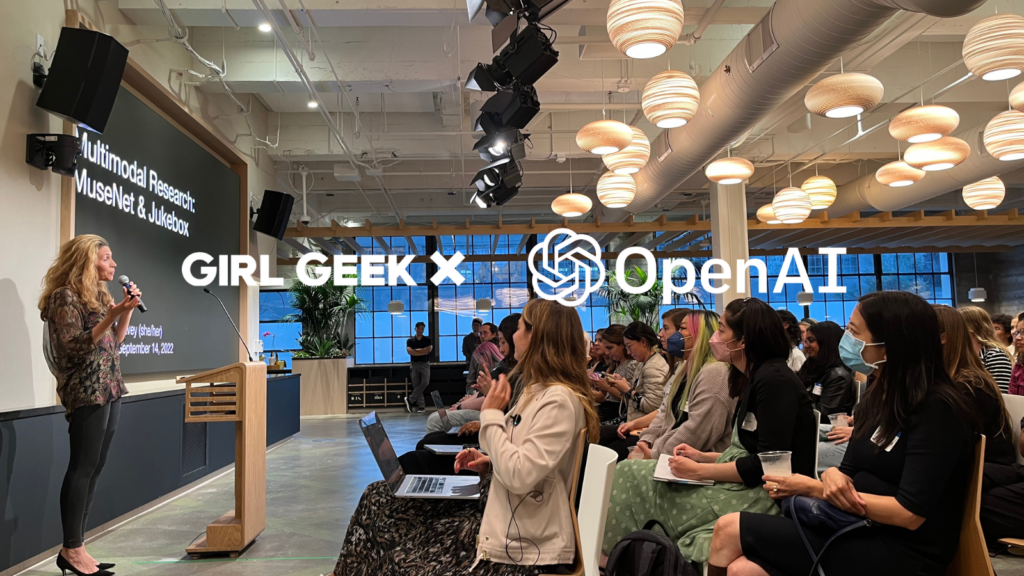
Christine McLeavey: I want to talk this evening about my own path through OpenAI, but especially about the two music models that I worked on during the time here. I thought I’d start by just going ahead and playing an example of each of the models. The first one, this is the one I worked on when I was doing the Scholars and Fellows program. This is MuseNet, which works in the MIDI domain, so this is the model trying to generate in the style of jazz. Okay, I’ll cut that off and then after I joined full time, I was lucky enough to collaborate with some amazing researchers here to work on a model that was instead working in the raw audio domain. The fun of that is you get to imitate human voices. This is trying to do the style of Elvis with lyrics by Heewoo. Okay.
Christine McLeavey: Elena mentioned before being at OpenAI, I was actually working as a pianist, I had done some math and physics in college, but obviously it had been a long time and so I think I took a good year of self studying before I applied to anything. And I thought I would just give a shout out to three of the online programs that I particularly liked at that point. They’re all amazing. But then I was lucky enough to join the first cohort of scholars that we had here. And at that point I was just trying to do this process of learning about all these different models. And I had this feeling that instead of just copying a model or copying what someone else has done, let me just try to translate it into a field that I know well, which was music. And so what became MuseNet was really my attempt to take all of the stuff I was learning and then apply it to the music domain instead.
Christine McLeavey: MIDI format is this really nice representation of music. I think of it as the way that a composer thinks of music, so it’ll do things like it tells you what notes it plays when, the timing of it, the volume of it, things like that, which instrument is supposed to play. But it loses all the actual detail of when a human takes it and performs it. You don’t get a person’s voice, you don’t get the sound of a great cellist, anything like that.
Christine McLeavey: The nice thing is it’s what you trade in expressivity, you get in this nice really meaningful representation. It does sound pretty terrible when you try to render materials. As a musician, just thinking about the structure of music, this was a nice simplification for a scholars project. What I did is I took a bunch of MIDI files and I tried to pull them out and turned them into a sort of language to make them look as much the sort of thing that you could get in your own net to predict as possible.
Christine McLeavey: I did things like I would always tell the model which composer or which band was going to be first and then things like what tempo was going to be when notes would turn on and off, and a wait token, which would tell the model how long to wait, things like that. And then what you end up doing is you translate that tokenization into just a dictionary of numbers and the model sees something like this. Which I think that this is the first page of a Chopin bellade or something.
Christine McLeavey: What the model is faced with is this task of given the very first number, what number do you think is going to come next? And then given the first two numbers, what number is going to come next? And when you first look at the first thing and when the model first sees it’s like how do you do this? What does that even mean? It feels like an impossible task. But what happens is the model sees many, many, many examples of this.
Christine McLeavey: And over time it starts to pick up on, ah, if I see 4,006 somehow I tend to see 586 more often after that or something. It starts to pick up on these patterns, which we know because we know the tokenization was like, oh, if a piano plays the note G, then probably soon after it’s going to turn off the note G or something. It has real musical meaning to us. But the model is just seeing these numbers like that. The nice thing is the model gets really good at this job and then you can turn it into a generator just by sampling based on, I thinks there’s like a 20% chance this token’s going to come next, so 20% of the time take that.
Christine McLeavey: The other really fun thing you can do is you can then study the sort of mathematical representation you’ve gotten for these tokens. So I was always giving it the composer or band token in the beginning and now you can look at the vectors or the sort of embedding that it learns through these composers.
Christine McLeavey: And as a musician it’s really fun because I would clearly think that Da Vinci and Ravel, for all these French guys are related and the model just picked up on the same thing, which is cool. But the other really fun thing is that you can mix and match those [inaudible]. So here is the start of one of my very favorite Chopin, Nocturnes. So I actually just gave the model the first six notes of that and this is what the model thought, if instead it was being written by [inaudible] It was a bunch of VPs. It goes on for a while, but I’ll cut it off there. And that was MuseNet.
Christine McLeavey: And then I ended up joining full time after that and I was lucky enough to collaborate with Prafulla and Heewoo on taking music generation over to the raw audio domain. And so in a way this is a much harder problem because now whereas in MIDI world you have just nice tokens which are meaningful in a musical way, raw audio is just literally 22,000 or 44,000 times per second.
Christine McLeavey: You’re recording how loud the sound is at that moment in time and the nice thing about it is it gives you all this expressive freedom, right? Literally any sound you can imagine you can represent as a sound wave, just audio recording to that. The trouble is there are just so many ways for those waves to go wrong or those patterns to go wrong. If you mess up on the short scale, it’s just like crazy hissing noise. If you mess up on long scale, your piece sadly starts getting out of tune or the rhythm drifts or so many ways it can go wrong, it’s really an unforgiving sort of medium. And the problem is now in order to get a minute of music, it’s no longer maybe 3000 tokens you have to do, it’s maybe a million numbers that you have to get correct.
Christine McLeavey: We approached this by looking at ways that we could compress the music to make it more tractable because at that point a transformer could maybe deal well with the context of 4,000 tokens or something. We used an auto encoder to do three different layers or levels of compression and the sort of least compressed on the bottom. The nice thing about that is it’s very easy to translate it back to the regular raw audio. If you put some original song in and then back out, you don’t notice any loss at all. Whereas if you put it through the most compressed version, the nice thing is now it’s super compressed, like 3000 tokens might get you half a minute of music or something. But if you go through this simple just trying to reconstruct the raw audio, it sounds really bad. You can sort of tell that someone’s singing but you’ve lost most of the detail.
Christine McLeavey: The nice thing about it is when you work in that top layer of tokens, now this looks a lot like the MuseNet problem or even just a lot language problem where you’re just predicting tokens. So we train a transformer on that. We sort of added in the same which person was singing, which band was playing, and then we also added in where you can write the lyrics in, so the model conditions on the lyrics and then generates these tokens. And then I won’t get into the details, but we had to train extra transformers to do this upsampling process so that you could get back to raw audio without totally losing all the detail.
Christine McLeavey: The fun thing is you can do things like ask it to generate in the style of Sinatra singing Hot Tub Christmas and I have to put in a book, these were lyrics by at, that point, GPT-2. All right. It’s a Christmas classic now. And then last I wanted to wrap up by talking a little bit about the multimodal team, which is the team that I’m really excited to be managing these days. It’s this really, really great group of people. Unfortunately, our current projects are all internal and I can’t talk about them, although stay tuned, we’ll be publishing them to the blog when we can. You might recognize Clip, which was work done by Alec and Jong Wook both on our team. This is, I guess, nearly two years ago already, but made a really big impact on the image work at that point. And then just to put in a plug for the team, we’re about a group of 10 at this point and we will be hosting a resident in 2023.
Christine McLeavey: Please reach out if anyone’s interested to talk more. And then we’re doing all sorts of projects in the sort of image, audio and video domains both on the sort of understanding side and generation side. And we end up working really closely with algorithms, which is the other team that tends to do a lot of awesome multimodal projects. But then also anytime we get close to things that we’re looking at putting out tech customers, we end up working with applied through that and then also obviously scaling because at OpenAI we believe deeply in this, get a good pattern and then scale it up and it becomes awesome. So thank you so much for your attention.
Elena Chatziathanasiadou: Thank you so much, Christine. That was awesome. So now next we’ll have Alethea. Alethea has spent the last couple of years at OpenAI working on getting neural networks to do math. Before that, they built large infrastructure health system, studied math and philosophy and spent lots of time singing karaoke. Welcome, Alethea.
Alethea Power: Thank you. So this talk is called If At First You Don’t Succeed, Try Try Again. It’s been a wild few years. I decided I wanted to give an uplifting and encouraging talk. It’s a short talk so it doesn’t get too deep into technical details, but if you’re interested in it, please find me afterwards. I will talk your ear off about it.
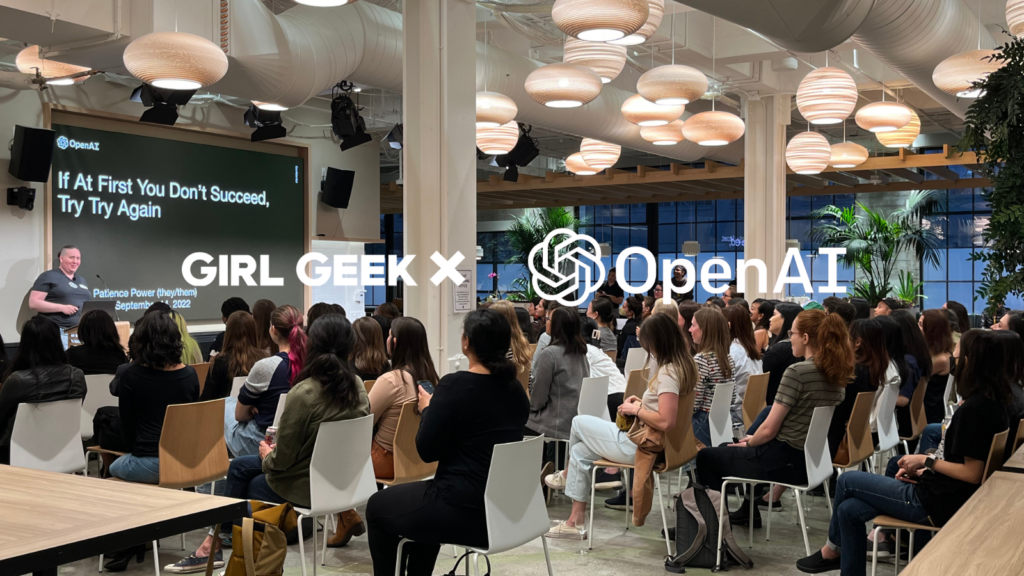
Alethea Power: Okay, my name is Alethea Power and yes, Patience is actually my middle name, which will be very relevant for this talk. Okay, so about 10 years ago I was a software engineer and site reliability engineer and my dream was to get into artificial intelligence, but I didn’t know how to do it. I didn’t have a degree in AI, I didn’t have any background in AI, I didn’t have any idea how to break in. So I thought, ah, I probably need to take some time off to study this before I can get into the field.
Alethea Power: I started saving up some money so that I could take time off to study. But by the time I had enough money saved up, I realized I needed to handle my gender issues. So I took that time off to go through a gender transition instead of studying AI. Eventually though I was finally ready to try and break into AI in some form or fashion and that was about the time that OpenAI hosted their last Girl Geek Dinner, that was in 2019. And I came to that talk and I met one of the recruiters who stunned me by telling me I didn’t need to have a degree in AI and I didn’t need to have a background in AI to be able to work here.
Alethea Power: She introduced me to the Scholars Program, the same program that Christine went through, which today is called the Residency Program. And I applied to that and I got in and I had the best mentor in the entire program, Christine. I’m second generation scholar up here. But there were in addition to the obstacles before, there were obstacles after joining the program as well, about three weeks after I joined, there was a pandemic, you may have heard about it. But despite spending a lot of time fearing that I might die or people I love might die for some reason or another, health or political, Christine was very kind and understanding and supportive and she helped me get to the point where I had learned a ton about artificial intelligence and managed to do a great project and I ended up applying full-time and I got three offers here. Thank you. I wasn’t trying to brag, but thank you. This is more to encourage you.
Alethea Power: I ended up taking a job on a team that was trying to teach neural networks to reason and do math. And what I want to talk about here is about a year after I joined that team, I released my first research paper called Grokking: Generalization Beyond Overfitting on Small Datasets. I’m going to give you a very basic introduction to what all that jargon means. And like I said, if you want more technical details, come talk to me afterwards. So first I need to explain how training neural networks works. If you have a background in ML, this is going to be very basic 101. If you don’t, it’s going to be exciting.
Alethea Power: Okay, so usually when we’re trying to train a neural network, we’ve got some amount of data that captures a pattern that we want that neural network to recreate in the future. And often if we’re doing what’s called supervised training, we’ll break that data up into training data and evaluation data. And you can think of this, the training data is sort of what we actually teach the neural network, what it learns from. This is like classroom education and evaluation data is basically like pop quizzes to see how much the neural network learned. And neural networks have this nice property where you can pop quiz them. They don’t learn anything from the pop quiz, they just tell you how they did and then five minutes later you can pop quiz them again and the questions are all new again, they have no memory of them. Throughout the course of training, we measure the performance of the neural network on both the training data, the classroom instruction and the evaluation data, the pop quizzes.
Alethea Power: And there’s two main ways we measure this. One is called loss. I won’t go into details right now about what loss is, but the short version is it’s a differentiable function calculus derivatives that we use to actually figure out how to modify the network, so it learns, when loss goes down. The network is learning. Accuracy is exactly what you would think of being like a test score, so 0% accuracy means you got every question wrong. A hundred percent accuracy means you got every question right. This is what a very successful neural network training looks like. You can see, oh, the x axis here on both of these graphs is steps of training. You can see that as we train this neural network along the loss on both the training and evaluation go down. It’s learning what it’s supposed to learn from and it’s able to generalize that to the pop quizzes.
Alethea Power: It’s doing well on the tests as well and then this is what it’s actually scoring. So by the end of this training it gets up to 90% accuracy, so it’s got an A. Sometimes though, if you train a neural network for too long, it starts to do what’s called overfitting. You might remember the word overfitting from the title of the paper. In this case, the neural network learns too much detail from the training set that doesn’t really generalize to the rest of the world. And so its performance on the quizzes starts to get worse. So an example of this in this paper, I was training neural networks to do math, basic mathematical equations. For instance, if it happened to be the case that the training data had more even numbers than odd numbers, and if it was trying to learn addition, then it might learn that usually the answer is going to be even. Well, in reality that’s not true in addition.
Alethea Power: In reality, you want to actually know how to add and the number’s going to be whatever it is. So that would be an example where it learned some sort of incorrect, non-generalizable information from the training set and that made it start performing worse on the evaluation set. And you can see here in this situation, the accuracy on evaluation would go back down. Sometimes, and this is very common when you’re trying to get a neural network to do math, you have an even worse situation where the same thing happens with your loss, but it consistently fails the pop quiz every time. Gets to a 100% percent accuracy on the training data and fails the pop quiz. This means the network and we were using similar kinds of networks to the ones Christine was talking about, just math instead of music, this means the network never really understood what it was learning, it just memorized it.
Alethea Power: This is like the kid who knows that when you say six plus four, you’re supposed to respond with 10 but has no idea how to actually add. So this was a common scenario when training neural networks to do math. They’re really good at pattern recognition, but they’re not always good at understanding a deep analytical precise truth underneath the pattern. Well then one day we got lucky and by lucky I mean forgetful. So one of my coworkers was running an experiment like this and he went on vacation and forgot to stop it. And so a week later he came back and it had just kept studying and studying and studying and studying and studying and studying and studying and studying and studying. And it learned. So what happened here was, it went into this overfitting regime where usually we’d say, ah, it’s learned all it can learn from this training data.
Alethea Power: There’s no more to learn and see, it still had zero accuracy and it just kept getting worse and worse and worse. And then suddenly long after it memorized all of the training data, it had an ‘aha’ moment and it was like, oh, all this stuff that I memorized actually makes a pattern and the pattern is addition or division or S5 composition or whichever task we had it working on. And then the loss started coming back down on the pop quizzes and it went up and it got a 100%. This is weird, this never happens in neural networks. We dug in and recreated this many times, implemented it twice, saw the same behavior with two completely independent implementations on a wide variety of tasks and there’s all sorts of other interesting stuff about when this happens and when it doesn’t, ask me in the questions afterwards.
Alethea Power: The point here is at first the network didn’t succeed, but it just kept trying the same way I did when at first I couldn’t get into AI, but I just kept trying. We named this phenomenon where it finally figures it out Grokking, and we named this after Robert Heinlein’s novel Stranger in a Strange Land. It’s a science fiction book and Grok is a Martian word in that book, which means, “To understand so thoroughly that the observer becomes a part of the observed to merge, blend, intermarry, lose identity in group experience.” And it turns out this is exactly what these neural networks do. I’m going to let you take pictures before I change the slide.
Alethea Power: This network was trying to learn modular addition and modular addition you can think of is adding hours on a clock. Also, thank you to Christine for that analogy. If you have 11 and you add 3 to it, you don’t end up with 14, you end up with 2 because that’s what happens on the clock. The clock is modular 12, we were having it learn modular 97, and then we tore open the network that had grokked afterwards to see what was going on inside of it and it had actually built internally this circular structure of the numbers. It had created the mathematical structure we were trying to get it to learn that allowed it to actually solve the problem. Did this with all different kinds of problems, so we had one network learning to compose permutations and it found what are called subgroups and co-sets out of that, details later. But the point is, it worked so hard for so long through so much failure that it became the knowledge it was trying to get.
Alethea Power: The point here is, that if your dream is to get into AI, even if you have no background in AI or whatever your dream is, it doesn’t matter. Keep trying and keep trying and keep trying and keep trying and maybe you can get there eventually. And in particular, if your dream is to work at OpenAI, which I highly recommend because this place is fabulous, then try, even if it’s not the background you have already, even if you feel like you have a weird background or you’re not like the people here or like the people in this field.
Alethea Power: We’re a humanitarian organization. Our core mission embodied in our legal structure and our financial structure is to make sure that artificial intelligence benefits all of humanity instead of just a small number of rich people in Silicon Valley. And to be a humanitarian organization with a humanitarian mission, we need a wide diversity of perspectives here. If you have a different life story, a different path, different perspectives than we’ve seen before, that makes you more valuable here, not less, so please consider applying.
Elena Chatziathanasiadou: Thank you so much, Alethea, That was awesome. And now next we’ll have Tyna, who’s on the policy research team currently doing our rotation on applied research and she participated in the OpenAI Scholars Program, has spent some time researching economic impacts of our models, building safety evaluations, and collaborated on web GPT and moderation API. Let’s hear from Tyna.
Tyna Eloundou: Wow, so many of you. Let’s see. Okay, this works. Hi, everyone, thank you so much for coming. I’m Tyna Eloundou, I’ll be speaking to you today about making language models useful. A bit about myself, let’s see, wow, I’m also a former scholar. I can’t make the claim to third generation because Alethea was not my mentor, but they were super helpful in making my experience here amazing. And part of that culture and that welcoming environment was a reason I chose to stay on after the scholars program [now the Residency program].
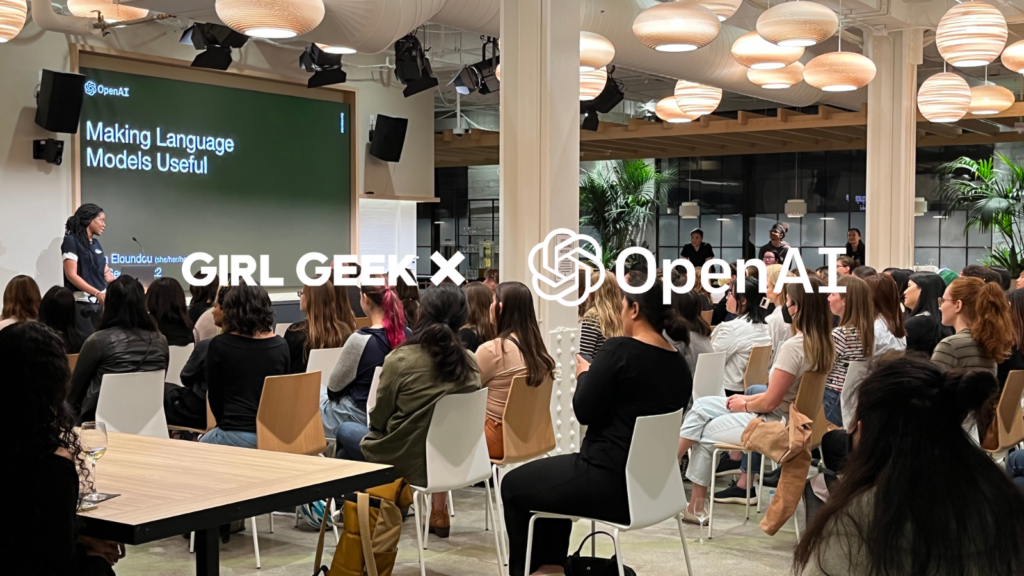
Tyna Eloundou: Today we’re going to be talking about language models and by language model, I mean any model that has language as input and output. So that could mean GPT-3, CODE-X, or BigScience’s Bloom, what have you. Okay, this is going to be the only equation you see throughout this talk and it’s really not that important, but I think it gives us some context as to where we’re going.
Tyna Eloundou: Looking back at this, this is the training objective for GPT-3 and for all GPT like models. Given a corpus of tokens, right? We define the objective to maximize this likelihood, L, which is defined as a conditional log probability over a sequence of tokens that is modeled by a neural network with parameters data that is trained by gradient descent. Now you can forget everything I just said.
Tyna Eloundou: Essentially this optimization produces these models that are trained to predict tokens, but that in itself may not be that useful on its own. I don’t think I’m giving away any secret sauce by revealing this equation to you, but it is remarkable that somehow we go from this to models that can produce, oh sorry, that can do that, right? Write prose, write code or parse data and so on.
Tyna Eloundou: I’d like to talk a bit about the notion of usefulness itself. One way to think about whether language models are useful in the first place is in the pragmatic sense. In the ideal scenario, we would be able to succinctly communicate our goals and preferences to a language agent without having to laboriously explain and list what to do and what not to do.
Tyna Eloundou: How did we initially get usefulness out of language models? When these models were first being developed in research labs, some researchers came with some ideas about how to really get them to do what it is that you want them to do. And these are two of the most prominent ones. One was few shot prompting, which is a method by which you really tell the model what the task is and before putting it on the spot, so to speak, you give it some examples of what you like to do, some demonstrations, right? For translate English to French, you could have a pen to [foreign language], I’m hungry to [foreign language], et cetera. And the translation that you actually want, you say, I would like to eat ice cream and hopefully with that same formatting you get the model to translate to French.
Tyna Eloundou: The other method is supervised fine tuning, which involves essentially just having examples for the model and then kicking off another round of training so the model can become hyper focused on your task and hopefully improve its performance on that task. So as many of you probably know, OpenAI has since then adapted this iterative deployment approach, which helps us put models in the hands of real people and understand how they interact with them. At the time of GPT-3 release, it was doing great by research standards, right? And unfortunately a lot of these research metrics are designed around these methods that we’d spoke about before, which are to prompt with few shot prompting or perhaps to do supervised fine tuning. Once we deployed, we really quickly learned that people don’t like prompt engineering. In fact, they don’t really like to do a lot to communicate their goals to the model, which is fine. It’s a feature, not a bug.
Tyna Eloundou: At its most helpful, a language agent can infer what we want without lots of specification and carry out those inferred goals effectively and efficiently. Unlike researchers, people were using natural language instructions to ask GPT-3 for what they wanted. But because of the training objective that we saw previously, the model was really tempted to just pattern match, right? If you gave it a prompt of write a short poem about a wise frog, it would very helpfully give you similar types of prompts instead of following your intent. This spurred a research effort within our alignment team to teach the models how to follow direct instructions. They did this using two insights. The first is borrowing from the supervised fine tuning or supervised learning literature where you can train the model based on examples or demonstrations, right?
Tyna Eloundou: You have a prompt and you tell them what you would ideally like it to do. And the second insight came from the reinforcement learning literature where you have some humans compare outputs. And so this model learns to generate, that model learns to compare, right? That model learns to tell this is good, this is bad. And so now with these two things, you can kick off this joint training process where you have a model that’s generating and then a model that’s critiquing, and this is good, this is not so good.
Tyna Eloundou: Over the course of training, the model learns to get better at pursuing this objective, which is no longer the pure language model laying objective and now it’s the instruction following objective. So the resulting model was InstructGPT, which is presented here. Well, yeah, you can see the output. It’s a poem, it’s about a frog, mentions wisdom, and it’s pretty short. I feel like all the requirements were met for following instructions there.
Tyna Eloundou: This was a plot that was quite striking to me. This is one of the main results from the InstructGPT paper. When I first saw this, it didn’t make a ton of sense until I really understood the research behind it. But I think that you can think of the Y axis as a proxy for usefulness and the X axis. We have model size and conventional wisdom has it that… We’re at OpenAI as you scale things, things get in general better. But you can see that even at its smaller size, right here, if you can’t see it’s 1.5 billion parameters, even at its smallest size InstructGPT was deemed to be more useful than any permutation of the base GPT model. So I started this discussion by talking about how research based approaches were not pushing far enough in terms of getting us usefulness out of these models. There’s now this emerging literature focused on helping models be more effective in tasks.
Tyna Eloundou: Broadly speaking, this literature involves having models break big problems up into smaller problems or things step by step before coming up with a final answer. And this does not need to be at odds with our human alignment driven research. In fact, right here you see a result by Kojima et al. and although their results are great overall across the board, we do see that they make the Instruct models even greater. There’s such a huge gap, a huge gain that we see with the Instruct series of models.
Tyna Eloundou: I would like to conclude by thinking about the next steps in this line of research. We know that there can be some instructions that can be malicious or exploitative or deceptive. If language models were to pursue usefulness at all costs, they might become dangerous in the pursuit of dangerous instructions or dangerous intent. Could there be other objectives we pursue along with usefulness that get us helpful but not dangerous models, perhaps kindness or hopefulness?
Tyna Eloundou: And lastly, with instructions, we’re mainly in the driver’s seat and we initiate interactions. As language models become smarter, perhaps kinder, more capable, it may be appropriate to think of them as collaborators and they may be capable of initiating ideation, creation among other things. What are the different modes of interaction we would like to have with these models? Would we want them to advise us? Would we want them to inspire us? Perhaps at Girl Geek X 2042, it’ll be a language model presenting about something new. Thank you.
Elena Chatziathanasiadou: Thank you so much all for joining. I guess with that note, I did want to mention that we’ll kick off mingling time and dessert in the area that we were before and our speakers will be available for you to ask them questions. We also have some of our recruiting team members here tonight. If you all want to come up to the front to just quickly introduce yourself or just say hi so that people can see you and then you can all come find us.
Elena Chatziathanasiadou: As I mentioned in the beginning, I’m Elena, I’m also hiring for the Residency program, so come talk to me, come find me. And then we also have some demo stands of our Dolly product and also our GPT-3, if you want to check them out. Jessica and Natalie will be doing those demos. So yeah, go find them as well.
Elena Chatziathanasiadou: Thank you all for being here. I hope you enjoyed it. Thank you to our lovely speakers and to Girl Geek X, to Cory and to all of our ops team and everyone who helped put this together and let’s go enjoy some dessert!
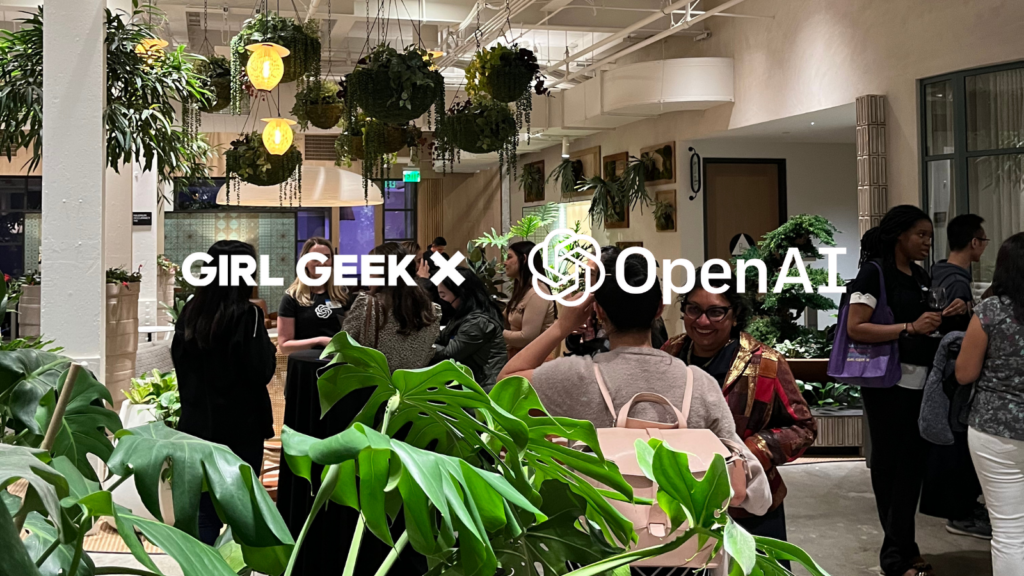
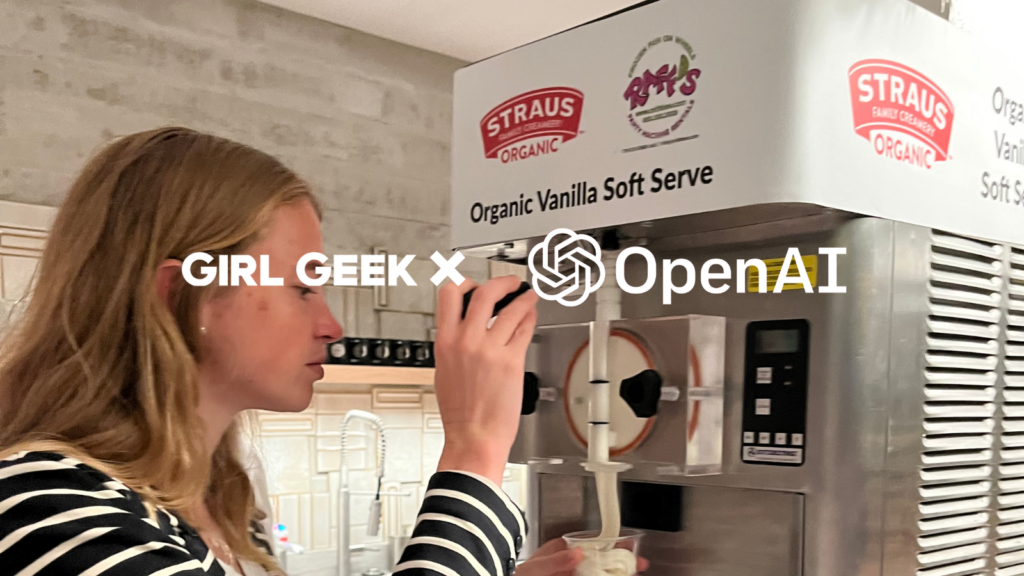
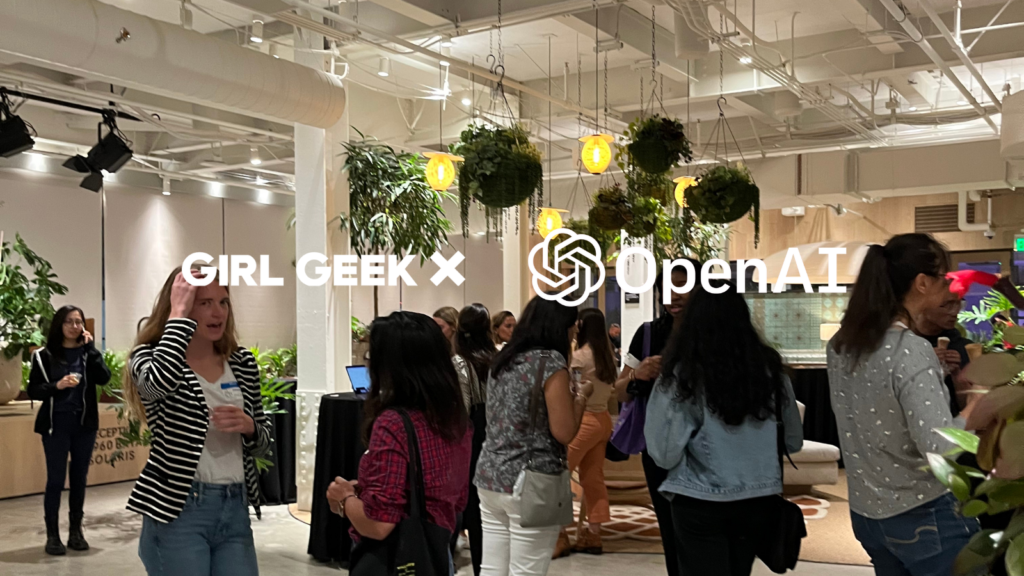
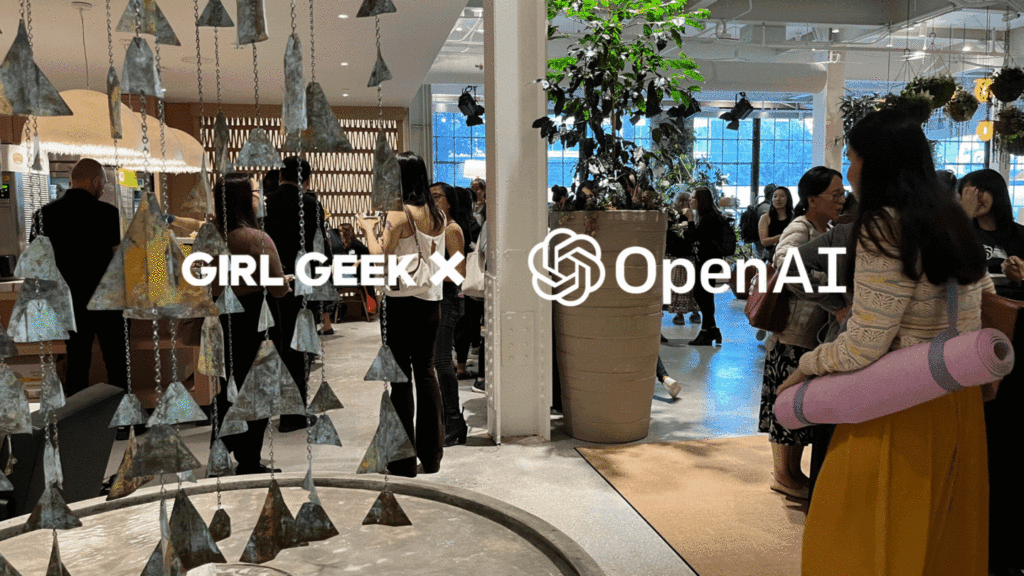
Like what you see here? Our mission-aligned Girl Geek X partners are hiring!
- See open jobs at OpenAI and check out open jobs at our trusted partner companies.
- Find more OpenAI Girl Geek Dinner photos from the event – please tag yourselves!
- Does your company want to sponsor a Girl Geek Dinner? Talk to us!