VIDEO
Yunwen Tu (Senior UX Designer at Vianai) and Sanchika Gupta (Data Scientist at Vianai) share perspectives on trustworthy artificial intelligence – the judgement of the users of the system, instead of simply trusting the technology. To build trust, you must start with the user and continuously monitor the data (e.g. training, outputs). In this session, gain insight into designing for users, and how to develop human-centered AI requiring human-centered design.
Like what you see here? Our mission-aligned Girl Geek X partners are hiring!
- Check out open jobs at our trusted partner companies.
- Watch all ELEVATE 2023 conference video replays!
- Does your company want to sponsor a Girl Geek Dinner or Career Fair? Talk to us!
Sukrutha Bhadouria: Sanchika Gupta and Tutu. The talk’s title is “Beyond the Algorithm: the Human Element in Developing Trustworthy AI”. Welcome, ladies.
Yunwen Tu: Thank you. Thanks everyone for joining the session today. Today we will share our thoughts and learning about building trustworthy AI. First of all, we will share a little bit more about ourselves, ourselves. My name is Yunwen, go by Tutu. I’m a user experience designer. I enjoy using design as an approach to learn and solve problems for people in both digital and physical way.
Sanchika Gupta: Hey, I’m Sanchika. I’m a data scientist with experience in the field of technology, cybersecurity, and human-centered AI. In a former life, I was a computer science professor, though while we are building the human-centered trustworthy AI and have been working with each other. I’m generally curious about this thought of what is the human involvement in building the trustworthy AI.
Sanchika Gupta: There are the three topics that we are going to discuss in this talk today. The uncertainty and concerns of AI development. How do we build and maintain trust relationship in AI and the unique value of human expertise in the age of AI.
Yunwen Tu: What’s your reason, conversation, or search about AI? For me, I chatted with my designer friends, and we talk about how AI can streamline some of our work, and is there anything can be replaced by it so that we can do things faster, such as making icons or some simple websites.
Yunwen Tu: We also talk about the new design trends that brought by the new development of AI. Since the breakthrough of the large development in large language model, more people have exposed to this technology and learn about the possibility how AI can be applied to their work. Lately, I have even heard users, they share that they have the desire to add this and that function using AI in their product after their self-education.
Yunwen Tu: In general, I got a sense that many of us are concerned and uncertain about the impact that AI can bring to us or our society. We start wondering, has the time finally come, is finally replacing us now? As we already see AI in is applied in many fields such as healthcare, automobile and et cetera. I ask my co-speaker Sanchika, where do you feel AI has already made a big impact and what kind of role is AI playing?
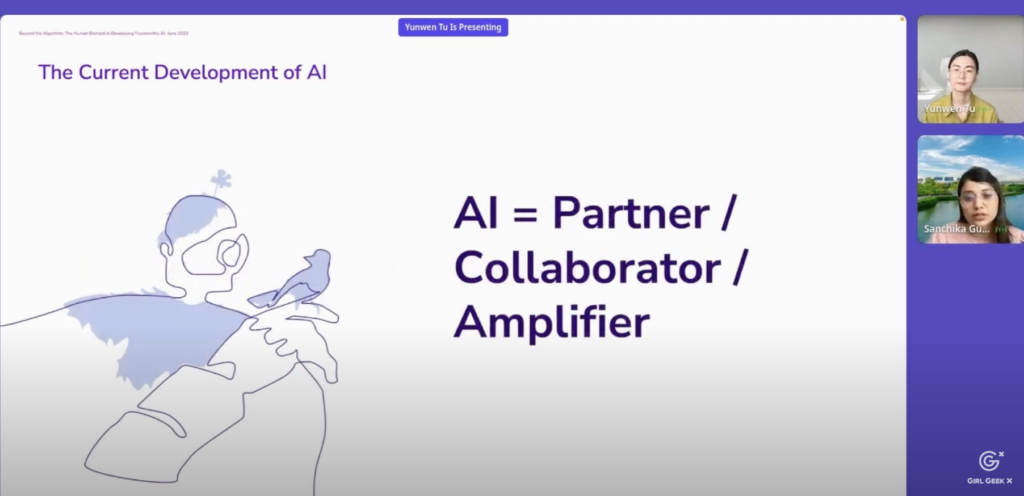
Sanchika Gupta: Automation of AI may lead to replacement of certain human roles. However, AI also presents us with newer opportunities and creates our jobs easier. Let me talk about certain examples where I feel AI has been present around for so, so many decades now, and it already feels as a partner. First example that I would like to talk about is natural language processing.
Sanchika Gupta: AI has significantly improved natural language processing capabilities. There are virtual assistants like Google Assistant, Amazon Alexa, which utilize the AI algorithm to understand and respond to voice commands. Now, it may seem a little trivial to talk about these because they have become a part of our daily lives, and I personally use them on a daily basis to like set up reminders, ask about the weather, ask it to provide directions for a destination while in the car, making our daily lives more convenient and efficient.
Sanchika Gupta: Another example I would like to give is of natural language translation. There are platforms like Google Translate, which leverage AI algorithms to provide real time translations between different languages. If you go to a country where they don’t speak your language, you can still communicate effectively. I have done it myself so many times. With this, I want to say that AI may automate tasks that require basic skills while human can focus on higher level responsibilities, harnessing creativity and imagination.
Sanchika Gupta: The next question is how can we have more access to the system so that we can use AI as a partner? Generally speaking, education and awareness are crucial in fostering trust in ai. Trust is very essential for building a reliable, transparent, and available use of system. AI has been present in various forms for many decades now., even during my university studies, I delved into the neural networks topic and AI’s potential to replace job was already circulating at that time.
Sanchika Gupta: However, the conversation around trustworthy AI only gained prominence with the emergence of large language models, instances of AI generated hallucinations where the system just make stuff up, started gaining attention while getting a recommendation for an unwanted TV show may have minimal impact. A recent incident in which an AI system made a judgment on a legal case without the attorney’s verification highlighted the potential consequence. These recent repercussions have brought the issue of trustworthiness to the forefront, causing it to enter the collective consciousness of all of us.
Sanchika Gupta: Let me throw another example on you and you tell me which would you prefer. If we were to compare a trust in an AI driven car accident versus a human driven car accident, what would you choose the second time? My opinion as humans, we tend to trust other humans more than AI. How can we bridge the gap?
Sanchika Gupta: I believe that by focusing on AI literacy, upskilling collaboration, and ethical considerations, us individuals can also be empowered to embrace AI as a tool to enhance our skills, productivity and relevance in the job market. Now, I would like to ask Tutu, how do you as a designer build and maintain trust relationship in ai?
Yunwen Tu: As a designer, I started this journey by understanding the technology, especially why AI failed and why people don’t trust AI. The major distrust I learned in AI is the lack of transparency. We haven’t considered enough that trustworthiness is a high priority in building that.
Yunwen Tu: Many AI models feel like a giant black box sitting between the input and output. We don’t, we don’t know how it works and it just did it work. When handling very mundane tasks such as grammar correction, language translation, it’s great when they’re magically done by machines. But when it comes to like riskier cases with bigger impacts such as loan approval, it’s impossible to rely on a black box like this, which you don’t even know if can understand 10% of your problem. There are also potential ethical biases in a model that needs to be monitored closely.
Yunwen Tu: We help user increase the transparency, the observability and the visibility to make the process and the model more interpretable and explainable in their context work. That’s also baked in our design principles.
Yunwen Tu: And as part of our design principles, we also use design thinking method that to work with our users to understand what does trust mean to them, and discover how AI can solve their business problems in a trustworthy way. For here, I would like to give you two examples that we use the user interviews and other thinking methods to solve problems for our users.
Yunwen Tu: The first example that we are working with an insurance company to reduce their business loss. Through many rounds of discovery interviews with underwriters and their managers, we actually found their primary challenge is not about finding the best algorithm to analyze their internal data.
Yunwen Tu : Instead, they want to understand better how the events happened in the past 10 decades that had impact their current business performance. In the meanwhile, we feel everything moves way faster now. For our underwriters, they need to quickly catch up with all the new updates such as the regulation change, the new settlements of lawsuits in their professional area. In the end, we build a tool that use natural language processing to help our user to connect the dots and find the needle in the ocean of internet data, which is the result we would not expect if we didn’t spend that much time to talk with our users.
Yunwen Tu: The second example I would like to share is related to our ops platform. And as part of my UX research, I regularly chat with different users such as data scientists, business ops, and et cetera.
Yunwen Tu: I found the expectations from our data scientists on monitoring AI models are very different from other general business users. They’re not looking for a no code or a fully automated experience. Instead, their philosophy is not to trust the data, not to trust the model until they have seen enough evidence to take action. It’s very crucial for us to deliver those insights clearly and efficiently.
Yunwen Tu: From our users, I actually learned trust is not purely top performance, not the best performing model. Trust means making informative decisions after peeling off the complexity and the root causes. Now Sanchika, what does trust mean to you as a data scientist and how have you built trust in your practice?
Sanchika Gupta: Demystifying the AI systems and ensuring reliability helps human use them with confidence. There are certain visible limitations of AI, for example, drift observability, root cause analysis, bias and ethical use are important to establish trust. So let me explain with an example how I establish trust in AI.
Sanchika Gupta: We as data scientists do not tend to trust our model or data. Instead, try to gather enough evidence around it and then be able to trust it. Let me talk you through that process.
Sanchika Gupta: Let’s consider a case of an AI driven customer service chat bot used by an e-commerce company. The AI chat bot is deployed to handle customer inquiries and support requests. Over time, they noticed that there is a decrease in the customer satisfaction scores and there is an increase in the unresolved issues.
Sanchika Gupta: The first step at this point, which I as a data scientist would like to do is to check on the model performance, let’s say, model performance evaluation, reveal that there is a decline in the chatbot’s accuracy and performance compared to previous months indicating a potential issue. Then we can begin closely monitoring the chatbot’s interactions and collect data on input queries, chatbot responses and user feedback. Now by looking at all of this data, closely plotting it, we might be able to identify certain patterns or anomalies in the chat bot’s behavior. During all of this observability and analysis, let’s say that we were able to identify a set of queries that consistently receive incorrect or nonsensical response from the chat bot. Now these queries stand out as potential outliers as they significantly deviate from the expected behavior. Then the next step could be to, let’s say, look at drift analysis on the chat bots performance.
Sanchika Gupta: We can compare key metricses like customer satisfaction scores, response accuracy, and resolution rates over different time periods. During this analysis, we notice there is a significant decline in performance starting around the same time as there was an update in chatbot’s knowledge base. Based on all these findings, we start the root cause analysis and discover that the update to the chatbot’s knowledge base introduced some incorrect or incomplete information resulting in chatbots diminished performance.
Sanchika Gupta: Through this example, we observe how model performance analysis, observability, outlier detection and drift analysis collectively contribute to the identification of the root cause leading to targeted corrective actions for enhancing the chatbots performance. This provides a glimpse into the case that I mentioned above showcasing the methods employed to established trust in an AI system. This also demonstrates the importance of human involvement in analyzing and improving the AI system, reinforcing the notion that despite all of its capabilities, AI cannot fully replace human judgment and decision making.
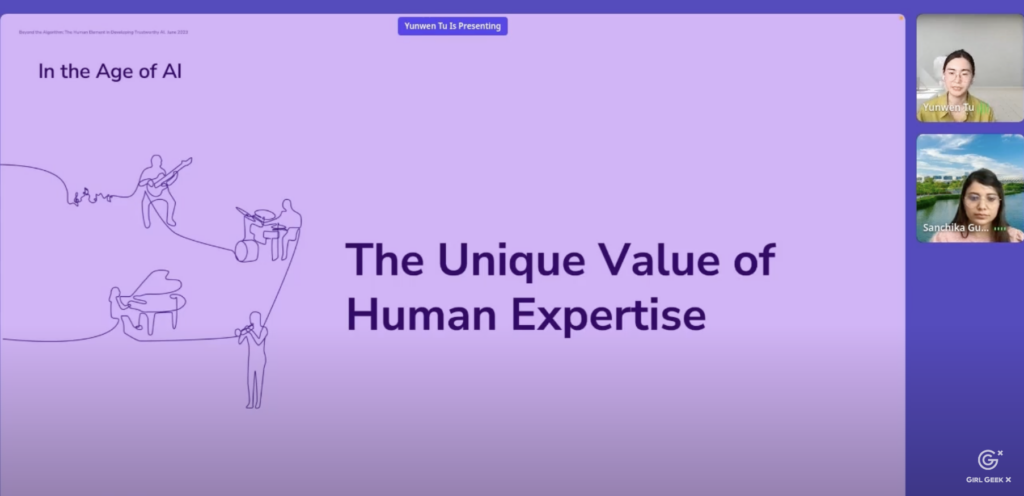
Sanchika Gupta: Now this leads to my third question that we would like to discuss here. What is the unique value of human expertise in this age of AI? Now again, creativity, imagination, and diverse opinions are very unique to humans. If let’s say there were to be a discussion, we humans can participate in a discussion and arrive at different conclusions in the same situation
Sanchika Gupta: At the same time, AI lacks the ability to participate in a discussion as an equal lacking both opinions or any standing in human conversations. Now let me quote another example here. New neural networks founder Professor Jeffrey Hinton, six years back in 2016 said, we won’t need radiologists to analyze scans and image perceptional hams can do all the scanning and diagnosis by themselves. Six years have gone by and we are nowhere nearby. It is not because of compute power or resources because I feel that compute power and resources have only been growing in the last couple of years.
Sanchika Gupta: What I believe is that AI can only solve very well-defined problems. What happens when it is posed with ill-defined problems? That is where human ingenuity comes in. All AI attempts to do is to recreate memory and computation capability of human brain. But what makes human a human is not just being able to solve the task, but be able to synthesize the complexity of this world and make decisions on the basis of that. Now, at this point, I would like to ask Tutu to give her thought process around this topic.
Yunwen Tu: Thank you, Sanchika. Those are great takeaways and what you just shared also, remind me again the user interview process in our design method. We do that to understand user’s journey and the pain points, and then present a personal story that summarize our learnings and the synthesis. Sometimes I feel AI is like an abstract persona that is summarized in a way with a well-designed cover and well-defined title. However, when we are doing the interview, the persona story, it’s not about creating an abstract figure, but to emphasize with our users’ needs, their feelings and the the reason why they’re making those decisions. There are all, and also this process are all done through our communication and synthesis in person. But AI does not learn new insights as we do in those contexts. They also don’t understand the complexity of the world like us.
Yunwen Tu: For example, when I read the news, the debates on the news, when I also work with different people, design for different users, I always feel so, and this comes from our unique experience, our desire and the belief that makes us like diverse, unexpected. And sometimes we argue we also have conflicting ideas, but this also made the world and humans like very unique that AI cannot replace with. That covers all we want to share today. Thanks for staying with us. And if we have a little bit more time, please feel free to ask us question now or reach out to us afterward. Thank you all.
Sukrutha Bhadouria: Thank you. Thank you ladies. Yes I would definitely encourage everyone to reach out to you both on and ask their questions on LinkedIn. Let’s keep the conversations going and I really encourage everybody to rewatch this content, share this content as much as possible with everybody. We really appreciate the time that you’ve taken out Sanchika and Tutu. Thank you everyone for attending. Bye everyone.
Like what you see here? Our mission-aligned Girl Geek X partners are hiring!
- Check out open jobs at our trusted partner companies.
- Watch all ELEVATE 2023 conference video replays!
- Does your company want to sponsor a Girl Geek Dinner or Career Fair? Talk to us!